From beer games to oligopolies: AI now commands complex supply chains
LLMs are not only capable of understanding strategic incentives but can also dynamically adjust behavior to optimize profitability under game-theoretic constraints, mirroring the decision landscape of supply chain managers.
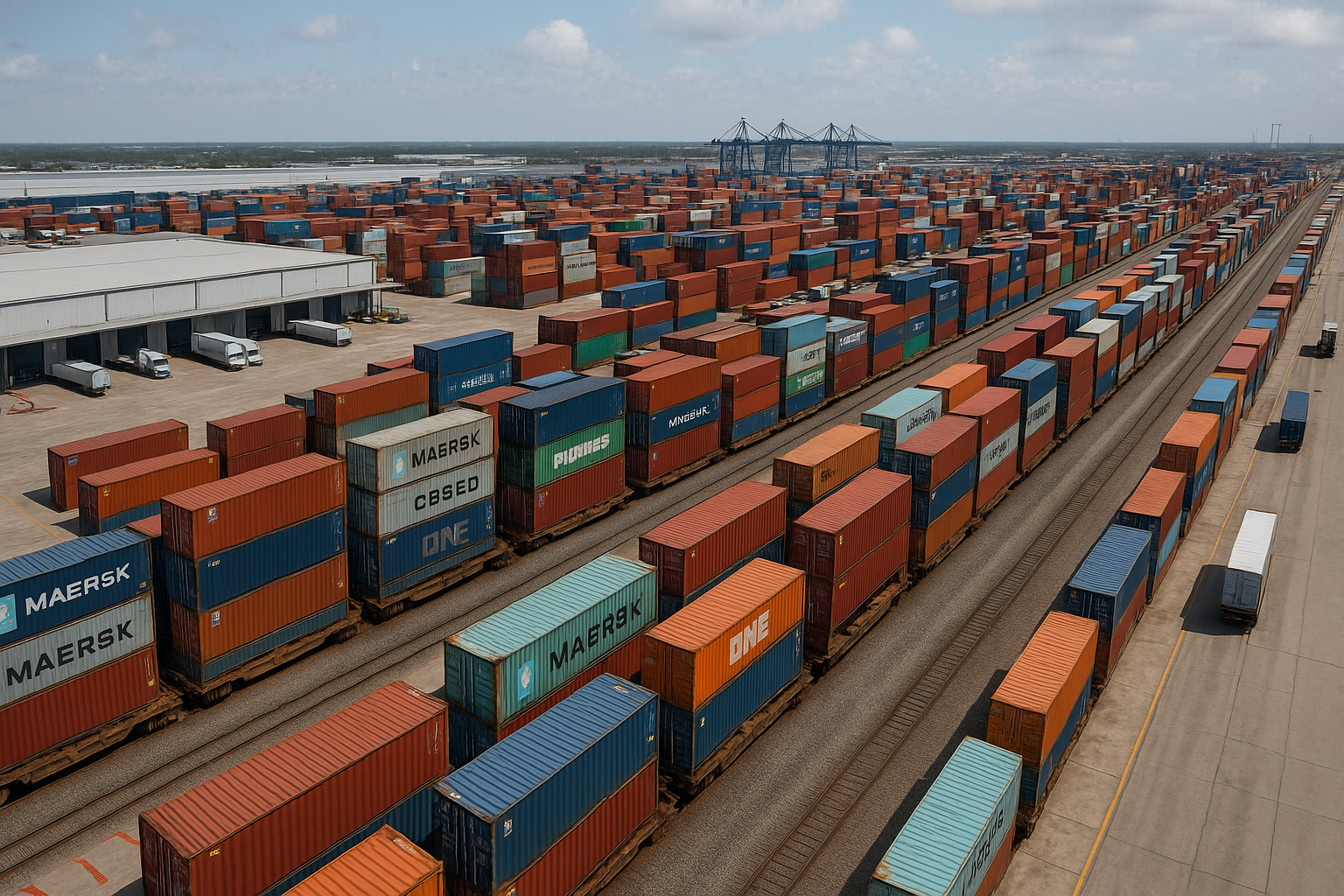
In a significant breakthrough for logistics and operations management, researchers have unveiled a specialized artificial intelligence framework capable of expert-level performance in supply chain decision-making. The study, titled "LLMs for Supply Chain Management" and published on arXiv, introduces a retrieval-augmented generation (RAG) system that transforms large language models (LLMs) into powerful tools for analyzing complex supply chain interactions, simulating market behaviors, and mitigating systemic inefficiencies.
Developed using domain expertise from globally recognized certification frameworks like SCMP and CPIM, the model integrates real-world supply chain knowledge with advanced reasoning capabilities. By embedding strategic simulation scenarios into the system, including beer games, Cournot and Bertrand competition models, the researchers demonstrate how LLMs can replicate classic supply chain dynamics and reveal novel insights under uncertainty, risk, and asymmetrical information.
Can AI truly master supply chain decision-making?
To evaluate if LLMs could meet professional expectations in supply chain management (SCM), the authors designed a two-part benchmark system based on standardized certification exams and operational simulation. Using real CPIM and SCMP questions, they found that the model, especially when enhanced with retrieval-augmented generation, achieved pass-level scores and expert-level reasoning accuracy. The AI model equipped with RAG consistently delivered superior results across various inventory management scenarios, even outperforming traditional strategies under different demand patterns.
These outcomes reinforce the potential of vertical LLMs to operate not just as knowledge engines but also as rational, autonomous agents capable of optimizing inventory, balancing trade-offs, and aligning with human-expert strategies in volatile environments.
How well can AI simulate market competition and strategic behavior?
To go beyond static decision analysis, the researchers modeled multi-agent supply chain interactions using horizontal game theory. Two key economic models, Cournot (quantity competition) and Bertrand (price competition), were used to simulate duopoly and oligopoly environments. In each scenario, the AI agents were given limited information and asked to adapt strategies over multiple rounds.
The agents’ production and pricing decisions consistently aligned with theoretical economic equilibria. Whether adjusting outputs in response to cost asymmetries or pricing under conditions of product differentiation, the LLM agents demonstrated rational, strategic behavior in competitive environments.
These experiments confirm that LLMs are not only capable of understanding strategic incentives but can also dynamically adjust behavior to optimize profitability under game-theoretic constraints, mirroring the decision landscape of supply chain managers.
Can AI help solve the bullwhip effect under risk and uncertainty?
One of the most persistent and costly problems in supply chain management is the bullwhip effect, where small fluctuations in consumer demand create amplified swings in upstream orders, leading to inefficiencies. The researchers adapted a four-tier simulation using the beer game framework to test how AI agents handle this challenge under different risk preferences and information-sharing setups.
When operating with isolated information, risk-averse agents triggered extreme order amplification, while risk-neutral agents maintained stable inventory behavior and minimized order variance. Information sharing across the supply chain dramatically improved performance across agent types, significantly reducing volatility. However, the benefit was less predictable for risk-seeking agents, suggesting that transparency may not always yield optimal outcomes.
These findings suggest that AI agents, when given sufficient domain knowledge and visibility, can actively reduce systemic volatility. This has direct implications for enterprises seeking to deploy AI decision-support systems in live logistics operations.
- READ MORE ON:
- AI supply chain solutions
- artificial intelligence in logistics
- supply chain optimization with AI
- LLMs in supply chain management
- AI-powered inventory management
- how large language models optimize supply chain decisions
- AI systems for strategic supply chain simulations
- autonomous agents in logistics and inventory planning
- FIRST PUBLISHED IN:
- Devdiscourse