AI on the blockchain? Next-generation novel framework makes it a reality
The rise of Blockchain 5.0 stems from a growing demand to overcome persistent bottlenecks in earlier AI–blockchain integrations. Existing solutions, like Ethereum-based smart contracts or decentralized marketplaces such as SingularityNET, often require AI model execution to happen off-chain, relying on external nodes that reduce transparency and introduce potential vulnerabilities. Furthermore, these models are typically hampered by high computational costs, lack of native support for AI logic, and limited consensus mechanisms capable of verifying AI outcomes.
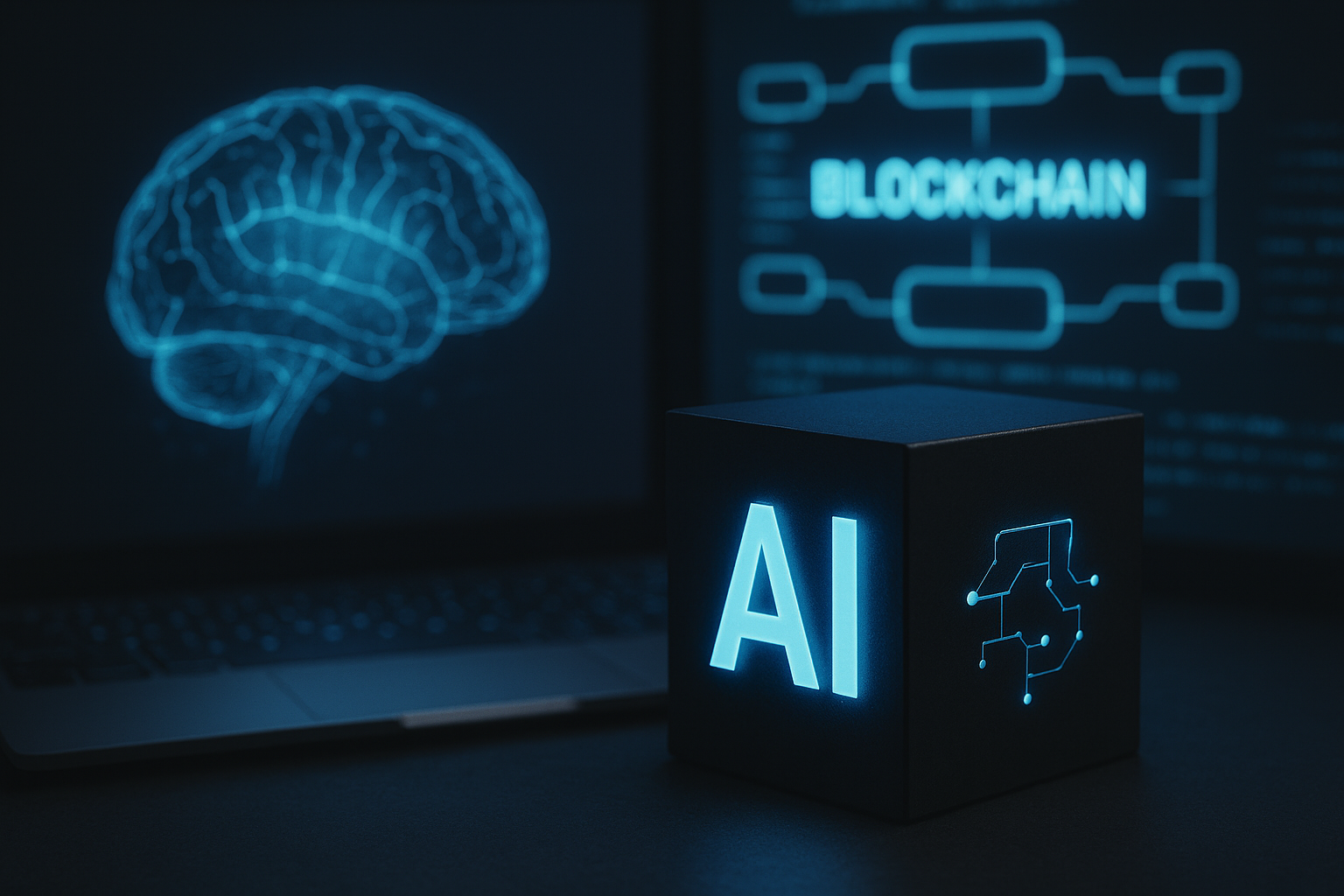
In a landmark development at the convergence of blockchain and artificial intelligence (AI), researchers from Inha University have unveiled a revolutionary hybrid blockchain framework that allows AI models to be executed directly on-chain. The study, titled “Doctrina: Blockchain 5.0 for Artificial Intelligence” and published in the journal Applied Sciences, introduces the Doctrina architecture as a prototype for Blockchain 5.0 - a generation that transcends storage and smart contracts to enable full-fledged AI processing within blockchain ecosystems.
Unlike earlier blockchain generations that relied on off-chain computation or served as ledgers for AI outputs, Doctrina achieves direct, decentralized AI model deployment and execution through a dual-layer blockchain composed of both public and permissioned nodes. This innovation opens new possibilities for secure, scalable, and auditable AI applications in domains where trust, transparency, and privacy are paramount.
What problem does Doctrina solve in the current blockchain - AI integration landscape?
The rise of Blockchain 5.0 stems from a growing demand to overcome persistent bottlenecks in earlier AI–blockchain integrations. Existing solutions, like Ethereum-based smart contracts or decentralized marketplaces such as SingularityNET, often require AI model execution to happen off-chain, relying on external nodes that reduce transparency and introduce potential vulnerabilities. Furthermore, these models are typically hampered by high computational costs, lack of native support for AI logic, and limited consensus mechanisms capable of verifying AI outcomes.
Doctrina addresses these limitations head-on by separating general blockchain operations and AI-specific tasks into two interoperable layers. The public blockchain handles immutable transaction logging, validation, and decentralization incentives. Simultaneously, a permissioned layer executes AI models in a secure, access-controlled environment, preserving data privacy and computational integrity.
This design mitigates the overhead of full-chain execution by reserving compute-heavy AI tasks for a trusted subnetwork, while recording verifiable results on the public chain. It ensures transparent model behavior without exposing sensitive algorithms or proprietary data. In doing so, Doctrina becomes the first architecture to reconcile the computational demands of AI with blockchain’s trustless infrastructure.
How does Doctrina enable native, secure AI model execution on the blockchain?
At the heart of Doctrina is a hybrid architecture built atop Ethereum’s smart contract infrastructure. Its architecture is characterized by a novel concept of “block tails”—metadata-enriched AI service containers linked to individual blocks. These tails, which include deployment scripts, dependencies, and versioning data, are verified within the permissioned network before being indexed into the public ledger. Once validated and signed through a record–approval–consensus (RAC) mechanism, the services are deployed, and execution costs are calculated dynamically based on size and resource usage.
AI model execution proceeds through a transaction-based request initiated by the user. The permissioned nodes monitor the public chain for such requests, execute the models securely, and emit results back through smart contract events, closing the loop between decentralized logging and private computation. The use of collective signatures ensures that all model executions are verifiable yet tamper-proof, offering strong guarantees against manipulation or spoofing.
Doctrina also introduces a gas-efficient cost structure. Initial deployment is costed based on model size and computational demand, while subsequent executions incur only minimal transaction fees. This predictability and fairness in cost modeling make Doctrina viable for organizations and developers who aim to scale AI services without unsustainable overhead.
What are the broader implications of Doctrina for future AI–blockchain ecosystems?
Doctrina’s architecture aligns with global trends demanding greater post-pandemic digital resilience and ethical AI governance. It directly addresses critical real-world needs in sectors such as healthcare, finance, and supply chain management, where AI insights must be explainable, secure, and audit-ready. For instance, medical institutions can use Doctrina to execute federated learning models without compromising patient data privacy, while public verifiability ensures clinical accountability.
Unlike Hyperledger or Ocean Protocol, which either lack native AI execution or emphasize data monetization, Doctrina takes an infrastructure-first approach. Its modularity allows stakeholders to manage AI model lifecycles, deployment, updates, validation, and monetization, within a unified, trust-minimized ecosystem. Moreover, the separation of duties between public and private layers permits efficient scaling: public networks maximize decentralization and visibility, while private nodes safeguard sensitive computation and enforce compliance.
Evaluation metrics from the study confirm Doctrina’s scalability and cost-efficiency. Tests across varying node configurations demonstrated that increased public node participation reduced marginal deployment costs, while larger private networks offered predictable, stable costs - ideal for enterprise-grade operations. These dynamics validate Doctrina’s hybrid design as both economically viable and technically superior for distributed AI workloads.
The system’s flexibility further supports cryptographic enhancements, including zero-knowledge proofs and verifiable computation, to bolster its security posture. Future enhancements may introduce asynchronous data relay protocols and compression strategies to optimize cross-layer interactions.
- FIRST PUBLISHED IN:
- Devdiscourse