From hallucinations to misfire: How AI may think like aphasic brain
The research team utilized energy landscape analysis, a method previously applied to human neuroimaging data, to examine dynamic patterns of network activity in both aphasic human brains and LLMs including ALBERT, GPT-2, Llama-3.1, and the Japanese-developed LLM-jp-3. The human data included resting-state fMRI scans from individuals diagnosed with various forms of aphasia, anomic, Broca, conduction, and Wernicke’s, as well as stroke patients without aphasia and healthy controls.
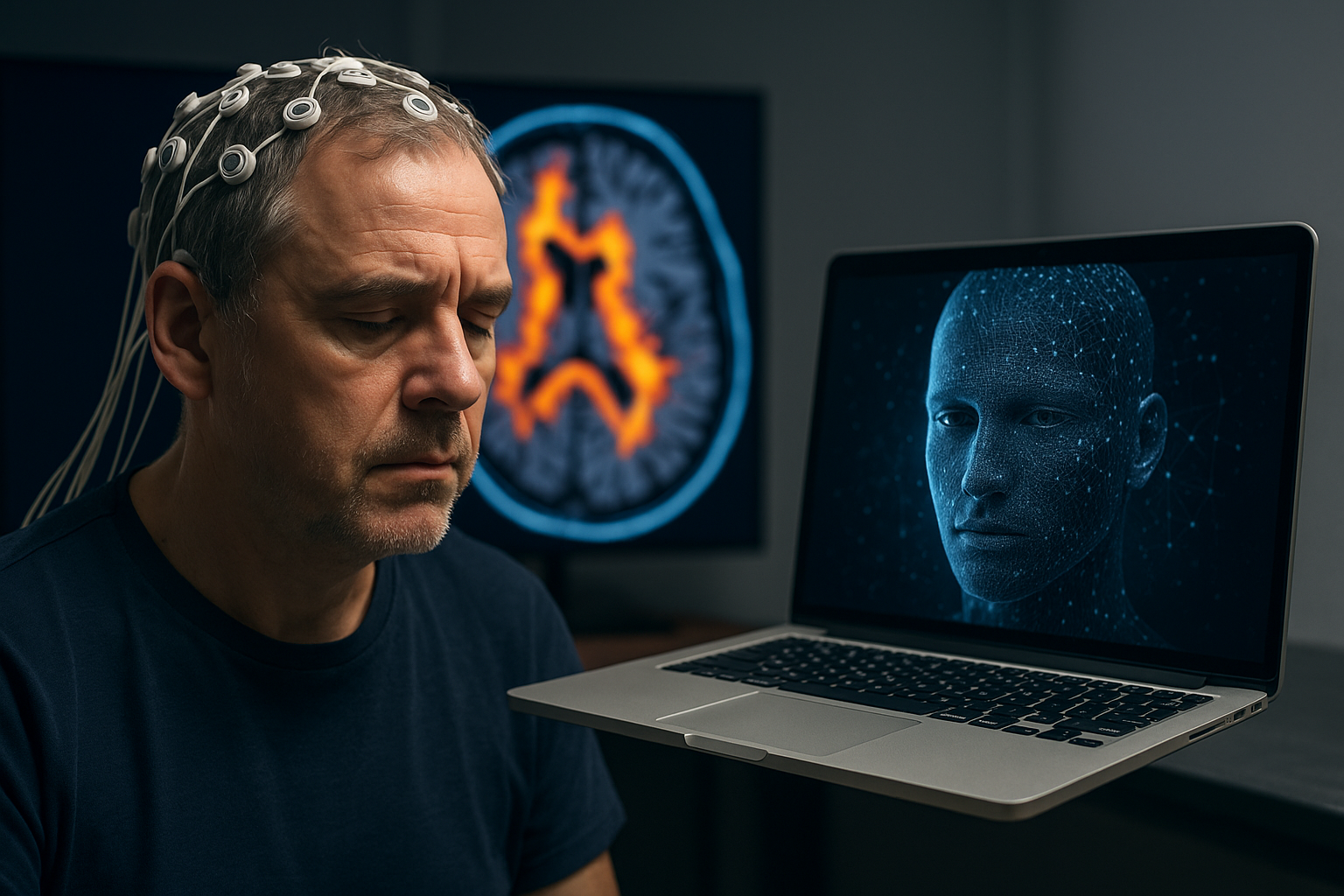
In a study that challenges the conventional boundary between biological cognition and artificial intelligence, researchers from The University of Tokyo have found that the internal processing patterns of popular large language models (LLMs) closely resemble the neural dynamics of humans with receptive aphasia.
The findings, published in the peer-reviewed journal Advanced Science under the title “Comparison of Large Language Model with Aphasia,” provide new insights into the brain-like behavior of artificial networks and open a potential path toward diagnosing AI limitations through neurological analogies.
How did researchers compare LLMs to human aphasia?
The research team utilized energy landscape analysis, a method previously applied to human neuroimaging data, to examine dynamic patterns of network activity in both aphasic human brains and LLMs including ALBERT, GPT-2, Llama-3.1, and the Japanese-developed LLM-jp-3. The human data included resting-state fMRI scans from individuals diagnosed with various forms of aphasia, anomic, Broca, conduction, and Wernicke’s, as well as stroke patients without aphasia and healthy controls.
Energy landscape analysis evaluates how often the brain or neural network transitions between states (transition frequency) and how long it dwells in each state (dwelling time). These behaviors were quantified using Gini coefficients to measure the polarization or uniformity of these dynamics. A highly polarized distribution indicates concentration around a few states (bimodal behavior), while a uniform one suggests widespread activity across many states.
In the human sample, researchers found that expressive aphasia (such as Broca's) exhibited uniform distributions of network state transitions and dwell times. In contrast, receptive aphasia (like Wernicke’s) showed polarized, bimodal distributions - features linked to impaired comprehension but fluent speech. When these neural patterns were compared with internal dynamics from the LLMs, all four models mirrored the bimodal and polarized characteristics of receptive aphasia, not those of expressive aphasia or healthy brains.
What does this reveal about AI behavior?
The internal network dynamics of LLMs revealed deeper “attractors” and greater polarization in both transition frequency and dwelling time compared to human brains. This polarization was statistically similar to the dynamics observed in individuals with receptive aphasia. Specifically, the Gini coefficients of the LLMs closely matched those of Wernicke’s aphasia patients, indicating a shared tendency toward repetitive yet fluent information cycling.
This finding sheds light on the root cause of an ongoing issue in AI - hallucinations, or the confident generation of incorrect content. Just as individuals with receptive aphasia produce grammatically correct but semantically incoherent speech, LLMs can string together linguistically fluid but factually incorrect text. The study proposes that this similarity in internal dynamics may explain the structural underpinnings of such behaviors.
However, the authors are careful to distinguish between causes. While hallucinations in LLMs stem from limitations in pretraining data and algorithmic biases, aphasia arises from physical brain damage, typically stroke-related, in areas like the superior temporal gyrus. Yet, the structural similarity of their internal network dynamics may provide a new framework for understanding and potentially improving AI systems.
Can this framework lead to better AI models?
The implications of the study extend beyond academic interest. The researchers argue that their framework could be used as a diagnostic tool for assessing and categorizing LLM behavior by comparing it to known neurological patterns. This approach offers an alternative to traditional benchmark testing, which focuses only on output evaluation. Instead, it emphasizes the "mental states" of AI models, akin to a cognitive diagnosis.
The team acknowledged certain limitations in their study, including the relatively small sample size for certain aphasia types and the constrained model sizes of the LLMs used (e.g., ALBERT with 235 million parameters versus GPT-3’s 175 billion). Additionally, they noted that while the energy landscape analysis is powerful, it abstracts away from biological mechanisms and does not directly explain the neurological origins of aphasia.
Nevertheless, the researchers assert that the approach can scale with further development. Future investigations could explore whether larger LLMs like GPT-4 exhibit similar dynamics or evolve toward different cognitive analogs. Furthermore, this framework could be integrated into LLM evaluation pipelines to pre-screen models for potential behavior risks such as hallucination or degraded coherence.
The study also prompts deeper questions about what it means for an artificial system to mirror human cognitive dysfunction. While the resemblance to aphasia may be incidental, emerging from architectural similarities in network computation, the alignment opens the door for interdisciplinary methods in both AI development and neuroscience.
- FIRST PUBLISHED IN:
- Devdiscourse