Generative AI powers climate policy simulations for Sub-Saharan Africa
Sub-Saharan Africa continues to be one of the most climate-vulnerable regions globally, facing disproportionate exposure to climate-related disasters while lacking the data infrastructure needed to support conventional policy planning. Integrated assessment models like DICE and RICE, while instrumental in global forecasts, often rely on detailed national emissions inventories and economic projections. For SSA, this level of granularity is either incomplete or absent across many states.
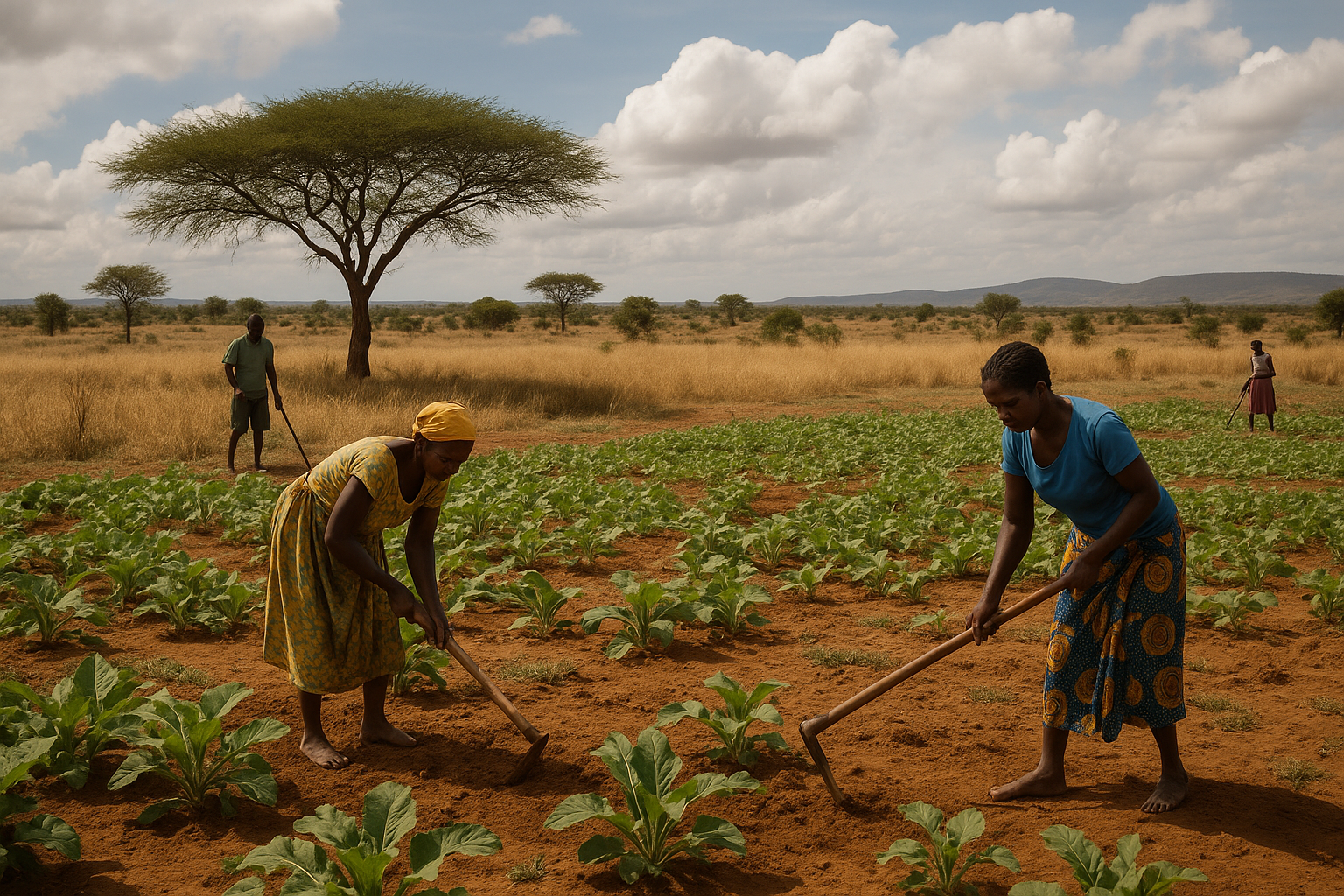
Researchers have introduced an AI-driven method to generate and evaluate climate policy scenarios for Sub-Saharan Africa (SSA), marking a transformative departure from traditional modeling approaches. The study, titled "AI-Driven Climate Policy Scenario Generation for Sub-Saharan Africa" and published on arXiv, outlines a scalable framework that leverages large language models (LLMs) and retrieval-augmented generation (RAG) to simulate policy scenarios rooted in regional climate challenges and COP documentation.
This novel methodology seeks to address persistent limitations in integrated assessment models (IAMs), which often falter under data-scarce conditions typical of the Global South. With a 88% validation rate of AI-generated scenarios and high correlation with human expert assessments, the framework offers both cost-effective agility and contextual precision. In a region grappling with rising emissions, fractured grid infrastructure, and uneven energy access, AI may now offer policymakers a new, scalable blueprint for adaptation and mitigation.
Why are traditional models inadequate for Sub-Saharan Africa?
Sub-Saharan Africa continues to be one of the most climate-vulnerable regions globally, facing disproportionate exposure to climate-related disasters while lacking the data infrastructure needed to support conventional policy planning. Integrated assessment models like DICE and RICE, while instrumental in global forecasts, often rely on detailed national emissions inventories and economic projections. For SSA, this level of granularity is either incomplete or absent across many states.
Such constraints have made scenario planning slow, expensive, and often disconnected from the ground realities of African energy transitions. In response, the study introduces a generative AI-based method that bypasses the need for complete datasets by training LLMs on historical COP documents, enabling the creation of synthetic yet plausible policy pathways. Prompts were crafted around themes such as renewable energy, equitable energy access, green hydrogen, and digital climate governance.
Scenarios were generated using the LLaMA3.2-3B model in a zero-shot configuration with a RAG setup that retrieved relevant passages from 94 pre-processed COP texts. The generation process also included structured follow-ups requesting detailed milestones, KPIs, risks, and dependencies, mimicking policy documents typically drafted by human experts.
How accurate and useful are these AI-generated scenarios?
Of the 34 climate scenarios produced, 30 passed validation by expert raters, who assessed the scenarios based on three key criteria: faithfulness (factual alignment with source material), answer relevancy (directness in addressing prompts), and context utilization (focused use of retrieved data). Evaluation was carried out using both human experts and two additional LLMs - Gemma2-2B and Mistral-7B.
Quantitatively, the AI-generated scenarios exhibited impressive alignment:
- Faithfulness scores ranged from 0.760 (human) to 0.966 (Gemma2-2B)
- Answer relevancy was highest across the board, averaging 0.993 (human)
- Context utilization peaked with Mistral-7B at 0.980, reflecting deep integration of COP-sourced material into policy responses
While LLMs generally matched human ratings for factual accuracy, discrepancies emerged in more interpretive aspects like answer relevancy. Spearman correlation analysis showed strong alignment between models and humans in faithfulness (r > 0.91), but only moderate or weak correlation in other areas, highlighting the importance of human oversight in final policy review.
Importantly, variability in scoring remained low across most metrics, affirming the reliability of mean performance scores. However, the study also flagged model behavior nuances: Gemma2-2B often favored strict context adherence, potentially sacrificing direct prompt alignment. Conversely, Mistral-7B excelled in recontextualizing information but showed inconsistent scoring on factual faithfulness.
What are the broader implications for climate governance?
The implications of this AI-assisted scenario framework are both technical and geopolitical. On the technical side, it significantly reduces the time, cost, and data burden typically associated with scenario planning. On the policy side, it allows regional actors, such as ministries, ECOWAS, and NGOs, to quickly generate tailored, evidence-informed pathways for issues ranging from energy poverty to carbon pricing to climate-resilient infrastructure.
Sample validated scenarios included structured pathways for:
- Achieving 30% renewable energy by 2030 through subsidies and community-based energy funds
- Green hydrogen development as an export sector supported by geopolitical risk modeling
- Decentralized microgrids and peer-to-peer trading to address last-mile electrification
- Smart grid investments and digital climate tools to enhance emissions tracking and adaptive policymaking
Notably, the methodology empowers policymakers to simulate high-risk or worst-case scenarios, such as governance collapse or unchecked emissions, which are rarely explored due to political sensitivities. By including speculative but plausible outcomes, this approach aligns with the broader climate planning imperative to anticipate not just expected futures, but also disruptive ones.
Still, the paper acknowledges ethical and operational limitations. Many LLMs are trained predominantly on Western data corpora, which risks encoding cultural or policy biases that may misalign with African values or institutional realities. Furthermore, the zero-shot prompting technique may limit response depth. To mitigate these risks, the authors suggest fine-tuning models on region-specific corpora and combining human judgment with AI-generated insights in a hybrid evaluation framework.
A new frontier for data-constrained climate policy
As Sub-Saharan Africa faces growing climate volatility with constrained institutional capacity, this study provides a practical, scalable framework for inclusive policy simulation. With funding from the Hamoye Foundation and tools open-sourced for reproducibility, the work underscores a commitment to democratizing access to advanced AI methods in low-resource settings.
By allowing governments and researchers to test policy pathways before committing to costly infrastructure or governance reforms, this AI-driven approach could fundamentally shift how climate adaptation is planned in the Global South. While challenges around representation, bias, and oversight remain, the study’s successful pilot implementation marks an important step toward aligning technological innovation with climate justice.
In a world rapidly transitioning toward digital decision-making, this research shows that climate resilience in Africa can be not just reactive, but strategically engineered.
- READ MORE ON:
- AI climate policy generation
- generative AI for climate planning
- AI climate governance tools
- Sub-Saharan Africa energy transition
- LLMs in climate change strategy
- artificial intelligence climate mitigation
- AI-powered climate scenario generation for Sub-Saharan Africa
- using large language models for climate policy in Africa
- machine learning for sustainable development in Africa
- FIRST PUBLISHED IN:
- Devdiscourse