New AI system offers real-time flood warnings for mountainous Kabul river basin
The Kabul River Basin is highly vulnerable to flooding due to its steep mountainous terrain, heavy seasonal rainfall, and glacial melt. Conventional hydrological models, such as rainfall-runoff or physically based simulations, have proven inadequate in this environment because they depend on extensive, consistent, and cooperative data collection across borders. But in the Kabul River’s case, upstream data from Afghanistan is scarce, and data-sharing protocols remain weak.
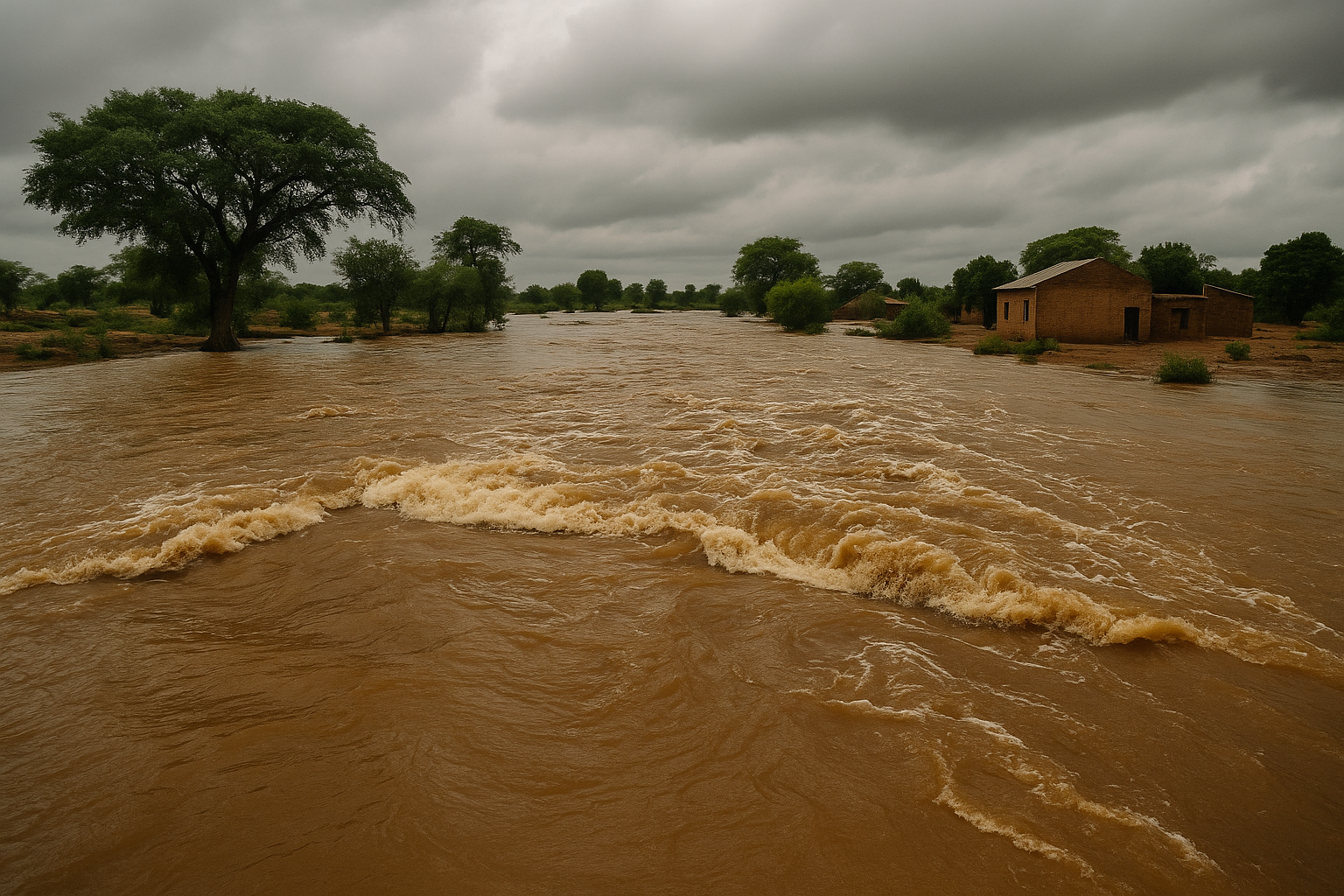
Researchers have developed a high-precision, AI-powered flood forecasting system for the flood-prone Kabul River Basin, offering a powerful new tool to combat rising climate threats in mountainous South Asia. The study, titled “Riverine Flood Prediction and Early Warning in Mountainous Regions using Artificial Intelligence” and submitted on arXiv, focuses on enhancing predictive accuracy through time-series machine learning models such as LSTM and GRU, with demonstrated capabilities for real-time and multi-day forecasts.
Using satellite-based climatic data from NASA’s MERRA-2 and ground discharge data from WAPDA, the system effectively predicts daily and five-day river discharge for early warning in the transboundary Kabul River that flows between Afghanistan and Pakistan. The models promise better disaster preparedness in a region where catastrophic floods in 2010 and 2022 displaced millions and exposed the limitations of traditional hydrological forecasting methods.
Why traditional flood prediction fails in mountainous and transboundary zones
The Kabul River Basin is highly vulnerable to flooding due to its steep mountainous terrain, heavy seasonal rainfall, and glacial melt. Conventional hydrological models, such as rainfall-runoff or physically based simulations, have proven inadequate in this environment because they depend on extensive, consistent, and cooperative data collection across borders. But in the Kabul River’s case, upstream data from Afghanistan is scarce, and data-sharing protocols remain weak.
Legacy systems often fail to capture the complex, non-linear interactions among climate variables like rainfall, snowmelt, and humidity. Further, these models are static and lack real-time adaptability. This makes them ineffective in fast-changing weather conditions where precipitation can rapidly lead to flooding in downstream districts like Nowshera and Charsadda.
The new AI-based models bypass these limitations by directly learning patterns from available climate and discharge data. By integrating multiple meteorological and hydrological variables, such as temperature, precipitation, humidity, and past discharge levels, the system allows for scalable and accurate forecasting even in data-scarce and politically sensitive zones.
How does the AI model improve forecast accuracy?
The researchers evaluated five machine learning and deep learning algorithms, SVR, XGBoost, ANN (MLP), LSTM, and GRU, using 15 years of data (2005–2020). The standout performer was the Long Short-Term Memory (LSTM) model, which achieved an R² of 0.9619 and a Root Mean Square Error (RMSE) of 140.96 m³/s in daily discharge prediction, significantly outperforming earlier models applied in the region.
The architecture was trained on a 5-day window of sequential meteorological inputs and discharge history. The model was optimized using a grid search, with best results at 16 epochs, batch size of 10, and the stochastic gradient descent optimizer. The accuracy was confirmed through visual analysis on page 15 of the report, where the graph comparing real and predicted discharge values showed the LSTM closely tracking daily fluctuations and spikes in river flow.
The Gated Recurrent Unit (GRU) model also delivered strong results, with an R² of 0.9551 and RMSE of 153.03 m³/s, making it a viable alternative where computational efficiency is prioritized. In comparison, more traditional models like SVR and XGBoost lagged, especially in modeling long-term dependencies inherent in flood behavior.
The researchers further validated the models using five-day ahead multi-step forecasting. On day one, the LSTM achieved nearly perfect accuracy (R² = 0.9378, RMSE = 2.23), but performance declined by day five (R² = 0.8357, RMSE = 293.48), suggesting that longer-term forecasts require additional data or more complex architectures. The GRU model demonstrated similar trends but slightly better long-term accuracy, with day five R² of 0.8613.
What are the real-world implications for disaster preparedness?
This model holds transformative potential for disaster management authorities in both Pakistan and Afghanistan. The system enables real-time flood prediction, helping mitigate the socio-economic impacts of recurrent flooding. The Kabul River Basin supports over 9 million people, many of whom rely on the river for agriculture and household water. With limited infrastructure and delayed alerts, past floods have destroyed homes, fields, and lives, especially during the massive 2010 and 2022 floods.
By integrating AI forecasting with existing early warning systems, agencies like Pakistan’s NDMA can now plan evacuation routes, allocate resources more effectively, and enhance community-level preparedness. Notably, the study aligns with key UN Sustainable Development Goals, particularly:
- SDG 6: Clean water and sanitation
- SDG 11: Sustainable cities and communities
- SDG 13: Climate action
- SDG 15: Life on land
Another critical outcome is the model’s use of satellite data, which offsets the chronic lack of upstream monitoring and opens the door for non-intrusive, remote-sensing-driven solutions. This circumvents the diplomatic sensitivities that often hinder transboundary hydrological collaboration, especially in politically tense regions.
The study also acknowledges the limitations posed by missing upstream discharge data, which could further enhance model performance if made available. It recommends regional cooperation for data sharing across the Kabul River Basin and suggests future work on ensemble AI models and integration with Internet of Things (IoT) sensors.
- READ MORE ON:
- AI flood forecasting
- Kabul River Basin flood prediction
- machine learning flood early warning
- flood prediction in mountainous regions
- AI flood warning system Pakistan
- artificial intelligence for riverine flood prediction in South Asia
- LSTM-based early warning system for transboundary floods
- machine learning in climate disaster preparedness
- climate resilience with AI-based flood prediction in South Asia
- FIRST PUBLISHED IN:
- Devdiscourse