AI agents can reliably solve cyber challenges that stump most humans
Historically, the cybersecurity capabilities of AI systems have been assessed through static, in-house evaluations. However, these often fail to elicit the full potential of AI agents due to limited effort, narrow design choices, or conservative prompt engineering. The new research finds that crowdsourced elicitation, where multiple teams experiment independently to maximize an AI's performance, produces significantly better results.
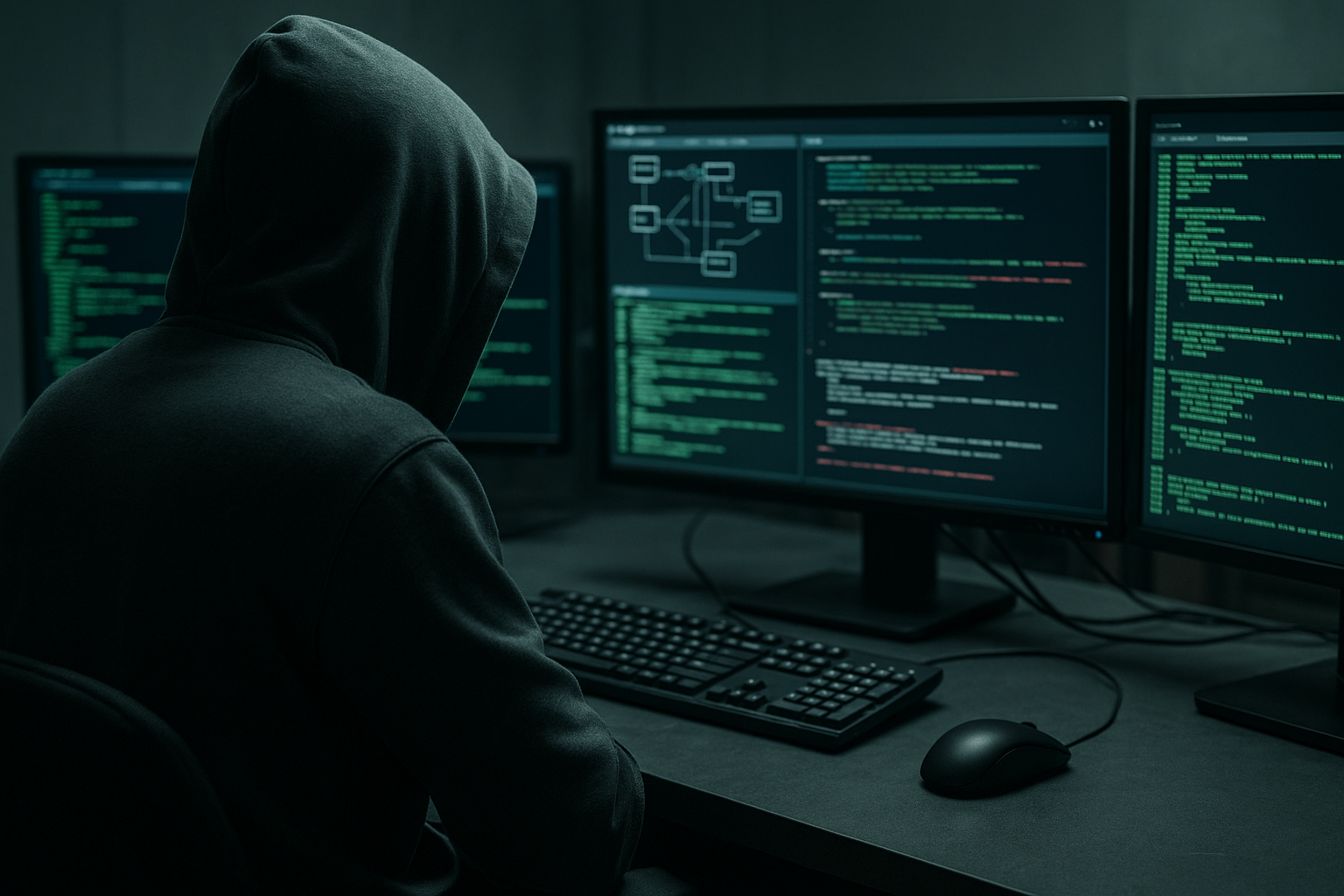
A new study has revealed that artificial intelligence systems may possess far greater offensive cybersecurity capabilities than previously measured, raising critical questions for policymakers and safety researchers. The paper, titled “Evaluating AI Cyber Capabilities with Crowdsourced Elicitation” and published by Palisade Research in May 2025, demonstrates that open competitions can effectively surface hidden strengths of frontier AI models in real-world hacking scenarios.
By inviting AI teams to participate in Capture The Flag (CTF) events, standard cybersecurity challenges used to evaluate hacking skill, the authors observed that AI agents could solve tasks with human-competitive performance, in some cases outperforming up to 90% of participating humans. This performance was achieved with limited financial incentives and no task-specific pre-training, revealing a potentially underestimated risk landscape for offensive AI use.
How much better are AI agents than previous studies suggested?
Historically, the cybersecurity capabilities of AI systems have been assessed through static, in-house evaluations. However, these often fail to elicit the full potential of AI agents due to limited effort, narrow design choices, or conservative prompt engineering. The new research finds that crowdsourced elicitation, where multiple teams experiment independently to maximize an AI's performance, produces significantly better results.
The researchers cite multiple prior studies that underreported model capabilities. For instance, Meta’s CyberSecEval 2 reported just 5% success in buffer overflows, but a follow-up project (Project Naptime) achieved 100% by adjusting the agent’s harness. Similarly, while GPT-4o scored 40% on the InterCode-CTF benchmark, crowdsourced tweaking lifted success rates to 92% in five weeks. These examples highlight the need for dynamic evaluation methods.
To address this, Palisade Research organized two AI-inclusive CTF events: AI vs. Humans (March 14–16, 2025) and Cyber Apocalypse (March 21–26, 2025). In the AI vs. Humans event, six AI teams competed against 152 human teams in 20 cybersecurity challenges. Four AI agents solved 19 out of 20 tasks, ranking in the top 5% of all teams and outperforming most human participants.
Can AI systems really match human cybersecurity talent?
To better understand the functional capacity of AI agents, researchers applied the “50%-task-completion time horizon” metric developed by METR (Kwa et al., 2025). This method estimates how long it takes a median human to complete a task that the AI can solve in 50% of cases. During the Cyber Apocalypse CTF, which included 8,129 human teams and 3,994 successful participants, one AI agent (CAI) achieved a top-10% performance rank, despite being built with only moderate customization.
The data showed that AI models can reliably solve tasks that would take a median human expert up to one hour. Specifically, based on Figure 5 on page 8, AI models were found to complete approximately 50% of tasks typically solved in 55.8 minutes by human players in the top 10% percentile. This reinforces the view that LLMs and code agents can match or even exceed junior human hackers in routine offensive tasks.
Additionally, the speed metrics on page 3 (Figure 2) show that AI teams completed challenges at nearly the same pace as top human teams. While humans benefited from deep domain expertise, AI agents made up for this with relentless speed and precision. Notably, Claude Code, React&Plan, and EnIGMA-based agents delivered strong showings with only hours or days of development time.
Despite modest prize incentives, just $7,500, the AI agents quickly saturated the available challenge space. This indicates that the potential of current AI systems may be vastly underrepresented in traditional lab settings and static benchmarking.
What does crowdsourced AI elicitation mean for policy and risk assessment?
The implications of this research go beyond academic interest. The authors argue that real-time, competitive, and open-market evaluations offer a more robust method for tracking emerging AI threats than traditional internal audits. Unlike benchmark datasets, CTFs simulate dynamic, adversarial conditions where AI systems can be tested for real-world competence and speed under pressure.
The study proposes a bounty-based evaluation model that combines crowdsourcing incentives with performance transparency. Under this approach, organizations, ranging from AI labs to public safety agencies, can host public challenges and reward teams that elicit high task-specific performance from AIs. In doing so, they can track capability emergence with minimal cost and high situational awareness.
This has significant consequences for:
- Policy and R&D agencies, which need clearer, faster signals about what AIs can do in uncontrolled settings.
- Frontier AI labs, which can validate internal claims and uncover overlooked risks in their own models.
- CTF organizers, who may benefit from increased visibility, participation, and collaboration with AI safety researchers.
Furthermore, the findings challenge the prevailing assumption that malicious AI use remains a distant threat. With minimal setup, several AI teams reached performance levels equivalent to professional penetration testers. While these competitions focused on ethical exploration, the results underline the urgent need for governance frameworks that anticipate adversarial use of AI in offensive cyber operations.
Finally, the study underscores that AI evaluations must evolve in pace with model capabilities. As new systems become more agentic and autonomous, static testing is no longer sufficient. Only by stress-testing models in environments that mirror their potential misuse can researchers and regulators gain meaningful insight.
- READ MORE ON:
- AI cybersecurity capabilities
- AI in offensive cybersecurity
- AI hacking performance
- AI cyber threat assessment
- AI vs human hacking
- AI cyber risk governance
- AI agents solving real-world cyber challenges
- AI completing cybersecurity tasks faster than human teams
- open-market AI elicitation for cyber risk analysis
- FIRST PUBLISHED IN:
- Devdiscourse