AIoT sparks smart energy revolution in solar power systems
The review highlights multiple AI techniques, such as convolutional neural networks (CNNs), long short-term memory (LSTM) models, and fuzzy logic, that support accurate short-term forecasting, efficient energy dispatch, and robust fault detection. Meanwhile, IoT-enabled components like microcontrollers, analog sensors, and smart meters gather critical data such as solar irradiance, panel temperature, and voltage. These are processed by edge devices or cloud platforms to enable adaptive solar tracking, MPPT (Maximum Power Point Tracking), and automated panel cleaning systems.
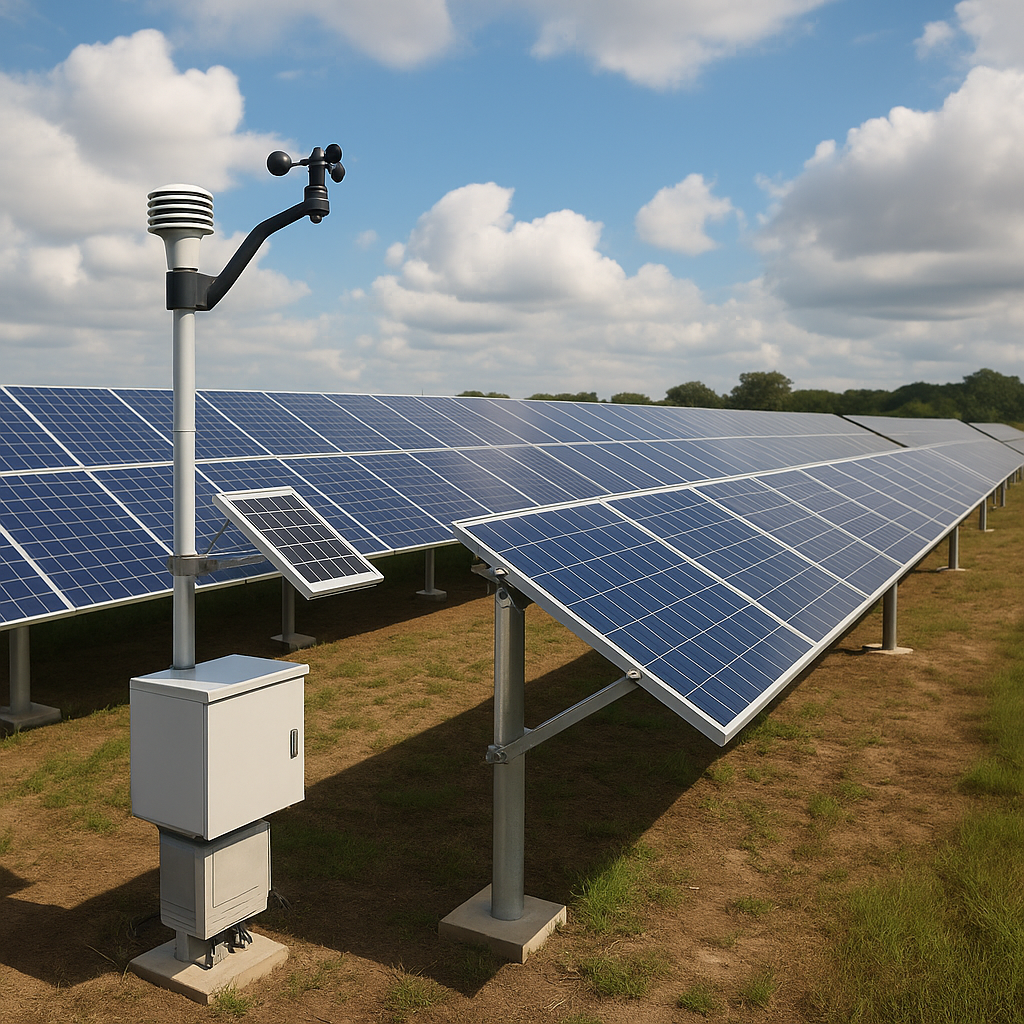
A landmark review of smart energy systems underscores how the convergence of artificial intelligence (AI) and the Internet of Things (IoT) is transforming the monitoring and management of solar power systems. The study, titled “Artificial Intelligence of Things for Solar Energy Monitoring and Control”, was published in Applied Sciences. It presents a comprehensive survey of how AIoT technologies are enabling unprecedented efficiencies in real-time energy monitoring, predictive maintenance, and fault detection in photovoltaic (PV) systems.
The research, led by scholars from Algeria and Mexico, introduces a structured taxonomy of AIoT applications across PV systems and addresses the challenges that must be overcome to achieve full deployment. With the global PV capacity exceeding 1200 gigawatts and solar contributing nearly 7% of total global electricity, the study offers vital insights into how advanced analytics and connectivity are redefining energy sustainability and resilience in solar infrastructure.
How is AIoT enhancing the efficiency and reliability of solar energy systems?
At the core of AIoT integration lies the synergy between intelligent data processing and connected sensor networks. Traditional PV systems, while capable of harvesting solar energy, lack the responsiveness and optimization that modern power demands require. AIoT remedies this by fusing machine learning (ML), deep learning (DL), and Generative AI (GenAI) with IoT infrastructure, creating systems that can self-diagnose, forecast energy needs, and automatically adapt operations in real time.
The review highlights multiple AI techniques, such as convolutional neural networks (CNNs), long short-term memory (LSTM) models, and fuzzy logic, that support accurate short-term forecasting, efficient energy dispatch, and robust fault detection. Meanwhile, IoT-enabled components like microcontrollers, analog sensors, and smart meters gather critical data such as solar irradiance, panel temperature, and voltage. These are processed by edge devices or cloud platforms to enable adaptive solar tracking, MPPT (Maximum Power Point Tracking), and automated panel cleaning systems.
Together, AIoT allows solar installations to identify inefficiencies, mitigate risks from fluctuating weather conditions, and maintain system integrity through automated diagnostics, cutting maintenance costs and prolonging system life.
What challenges must be overcome for AIoT-enabled solar systems to scale?
Despite its promise, the deployment of AIoT in real-world PV systems faces several technical and environmental challenges. According to the study, data quality and model adaptability remain major hurdles. Many AI models are vulnerable to poor generalization due to overfitting on clean training datasets, which don’t reflect the noise and variability of actual field conditions. Limited access to high-resolution, real-time data, due to degraded or miscalibrated sensors, also compromises decision accuracy.
Another major constraint is the computational burden of deep learning models, which demand substantial processing power and are often incompatible with energy-constrained edge devices. In locations with unstable network connectivity, maintaining real-time data transmission becomes even more difficult. These operational hurdles are compounded by cybersecurity risks, particularly when AIoT systems rely on distributed or cloud-based infrastructures.
To address these challenges, the study proposes future directions including the development of lightweight AI architectures, robust signal-processing methods for noisy environments, and the deployment of self-calibrating, energy-efficient sensors.
What emerging technologies will define the future of AIoT in solar energy?
Looking ahead, the evolution of AIoT systems in PV environments will be shaped by the convergence of Edge AI, Federated Learning, Blockchain, Digital Twins, and 6G networks. Each technology contributes uniquely to the enhancement of performance, security, and scalability.
- Edge AI allows data processing directly on local IoT devices, reducing latency and energy consumption while improving real-time responsiveness.
- Federated Learning enables collaborative AI training across multiple PV installations without centralized data storage, enhancing privacy and data security.
- Blockchain introduces secure and transparent peer-to-peer energy transactions, essential for decentralized energy markets.
- Digital Twins provide virtual models of solar systems that simulate scenarios for predictive maintenance and performance tuning without physical intervention.
- 6G Networks will facilitate ultra-reliable, high-speed communication across dispersed energy networks, allowing for seamless AI-based automation and synchronization.
In parallel, advanced analytics, such as anomaly detection, synthetic data generation using GenAI, and hybrid cloud–edge computing, will support predictive insights and dynamic system control. These tools collectively lay the groundwork for self-optimizing, autonomous solar infrastructures that adapt to real-time conditions and ensure peak efficiency.
Widespread adoption will depend on international collaboration, standardization of communication protocols, and open-source initiatives that democratize access to training data and tools. According to the researchers, scaling AIoT solutions in the PV sector will require unified frameworks that ensure interoperability among diverse systems while safeguarding operational transparency and cybersecurity.
- READ MORE ON:
- AIoT in solar energy
- solar energy monitoring systems
- AI for photovoltaic systems
- artificial intelligence in renewable energy
- AI-powered PV systems
- integration of AI and IoT in solar energy systems
- artificial intelligence of things for solar power optimization
- AIoT-enabled real-time solar energy analytics
- smart infrastructure for renewable energy control systems
- FIRST PUBLISHED IN:
- Devdiscourse