Survival-focused AI optimizes lung cancer treatment using genomic data
By integrating genomic biomarkers with advanced algorithms, the team constructed a decision-support system capable of offering personalized chemotherapy recommendations. The models were designed to address a critical challenge in lung cancer treatment: determining which patients gain meaningful survival advantages from chemotherapy and which do not.
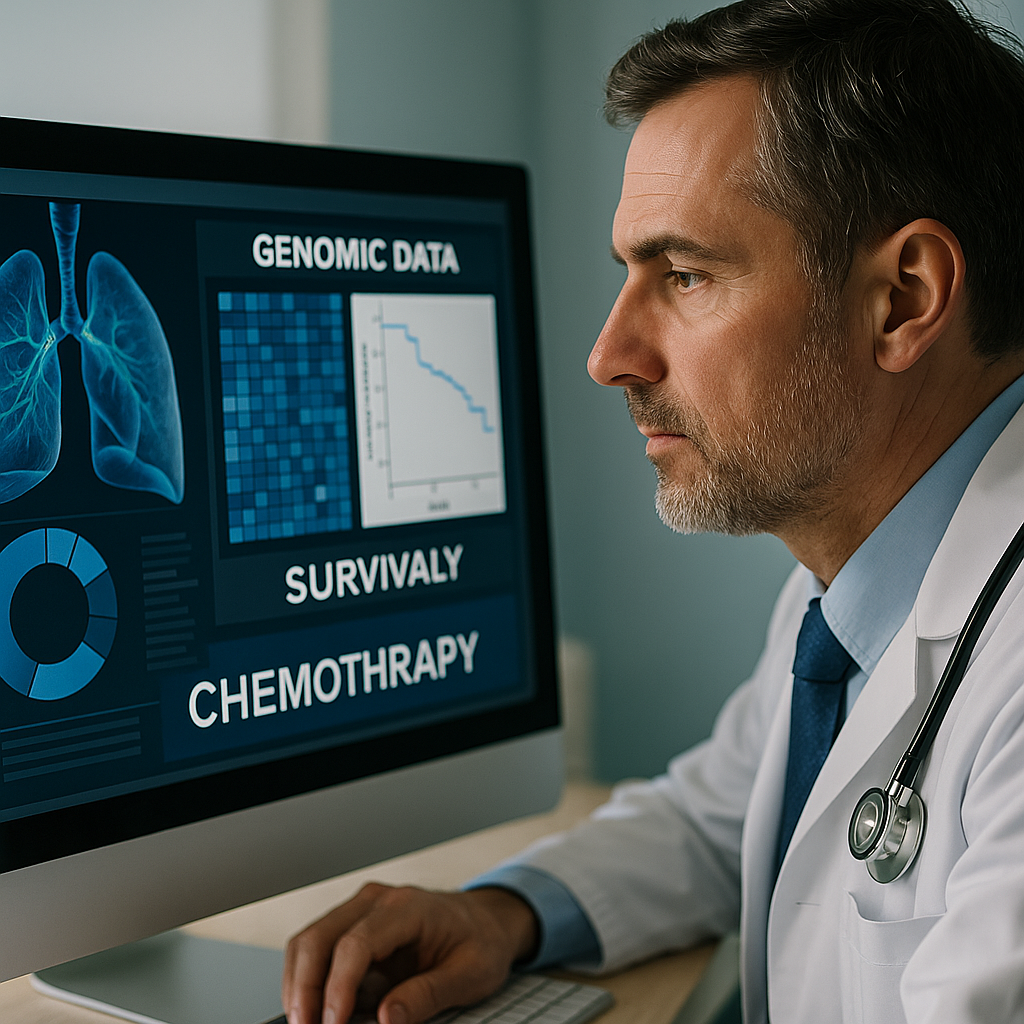
A new study has demonstrated that artificial intelligence can significantly improve chemotherapy treatment decisions for patients with early-stage non-small cell lung cancer (NSCLC). Published in the Journal of Personalized Medicine, the study titled “AI-Guided Chemotherapy Optimization in Lung Cancer Using Genomic and Survival Data” employs machine learning models to predict which patients are likely to benefit from adjuvant chemotherapy, potentially reducing unnecessary treatment and associated toxicities.
Researchers developed and tested three AI-powered survival prediction models using gene expression data and clinical outcomes from 155 NSCLC patients. By integrating genomic biomarkers with advanced algorithms, the team constructed a decision-support system capable of offering personalized chemotherapy recommendations. The models were designed to address a critical challenge in lung cancer treatment: determining which patients gain meaningful survival advantages from chemotherapy and which do not.
Can Machine Learning Discriminate Treatment Outcomes Effectively?
The study applied three distinct modeling frameworks: a bagging ensemble of elastic net-regularized Cox regression models, Random Survival Forests (RSF), and a deep learning neural network model known as DeepSurv. All three incorporated clinical variables, such as age, sex, and cancer stage, alongside genomic features extracted from two public NSCLC gene expression datasets: GSE37745 and GSE29013. Feature selection was performed using Cox-based univariate screening and leave-one-out cross-validation, reducing over 54,000 probes to a relevant subset of 1,834 features.
Among the models tested, RSF yielded the most consistent performance across both training and test datasets. The RSF model achieved a concordance index of 0.885 in the test set, a strong indicator of its predictive discrimination. Patients who adhered to RSF-generated treatment recommendations exhibited significantly improved survival outcomes compared to those who did not, with a median survival advantage of more than two years. The log-rank test showed statistical significance (p = 0.014), validating the model’s clinical relevance.
The DeepSurv model, while outperforming others in raw accuracy with a test C-index of 0.982, demonstrated weaker separation between survival curves for adherent and non-adherent patients. This limited its interpretability and suggested potential overfitting or a reduced ability to stratify patient groups effectively. The bagging Cox model, although highly interpretable and effective in training data (C-index of 0.996), suffered a drop in test performance (C-index of 0.709), indicating issues with generalizability.
Which Biomarkers Were Identified as Key Predictors?
In addition to modeling survival outcomes, the study also aimed to identify genomic signatures that could predict chemotherapy benefit. Through the RSF model’s variable importance rankings, the researchers highlighted several genes strongly associated with survival outcomes in NSCLC. Among these, transthyretin (TTR) emerged as the top-ranked feature. TTR is known for its role in transporting thyroid hormones and retinol, and low levels have been linked to malnutrition and systemic inflammation in cancer patients. The study referenced prior work establishing TTR as a marker for poor prognosis in lung cancer patients undergoing chemotherapy.
Other notable genes included MTURN and ETV3. MTURN, involved in neural differentiation, has recently been implicated in lung cancer through blood-based mRNA signatures, particularly in platelet-derived RNA. ETV3, a transcription factor, is associated with immune modulation and tumor suppression. Its dysregulation may influence how tumors evade immune response and resist systemic therapies. Together, these findings not only support the use of genomic data in clinical decision-making but also contribute to the ongoing search for reliable biomarkers in lung cancer therapy.
The integration of these markers into predictive models allows for a refined risk stratification framework. Instead of treating all patients uniformly, clinicians can use such data to determine which individuals are likely to achieve meaningful survival benefits from ACT and which may safely avoid it, sparing themselves from the toxicity and cost of unnecessary treatment.
How Will These AI Models Influence Future Lung Cancer Treatment?
The implications of this research extend beyond algorithmic modeling. The RSF model, which delivered the best trade-off between accuracy and interpretability, is poised for potential integration into clinical settings. Unlike more opaque neural network models, RSF offers visibility into which variables drive its predictions, making it more compatible with current oncological workflows. The authors emphasized that while DeepSurv demonstrated high precision, its complexity may hinder practical adoption without supporting tools for interpretability, such as SHAP values or explainable AI frameworks.
Despite these advances, the study acknowledged several limitations. The sample size, particularly in the test cohort of 31 patients, may limit the statistical power of subgroup analyses. The datasets used, while publicly available and well-annotated, lacked demographic diversity, which may restrict the model’s generalizability. Additionally, although normalization techniques were applied to harmonize the data, residual batch effects from merging different gene expression studies could not be fully excluded.
Future research will need to validate these findings in larger, more diverse patient populations, potentially using external datasets like those from The Cancer Genome Atlas (TCGA). Incorporating multi-omics data, including proteomics and metabolomics, could further enhance the precision of these AI models.
- FIRST PUBLISHED IN:
- Devdiscourse