AI transforms global supply chains with precision, resilience and sustainability
AI’s contribution to process optimization spans demand forecasting, inventory control, logistics planning, and operational automation. The review details how AI improves decision support systems by enhancing accuracy, reducing operational errors, and increasing cost-efficiency. Generative AI and digital twins have emerged as transformative technologies, allowing organizations to simulate operations and proactively adapt to disruptions.
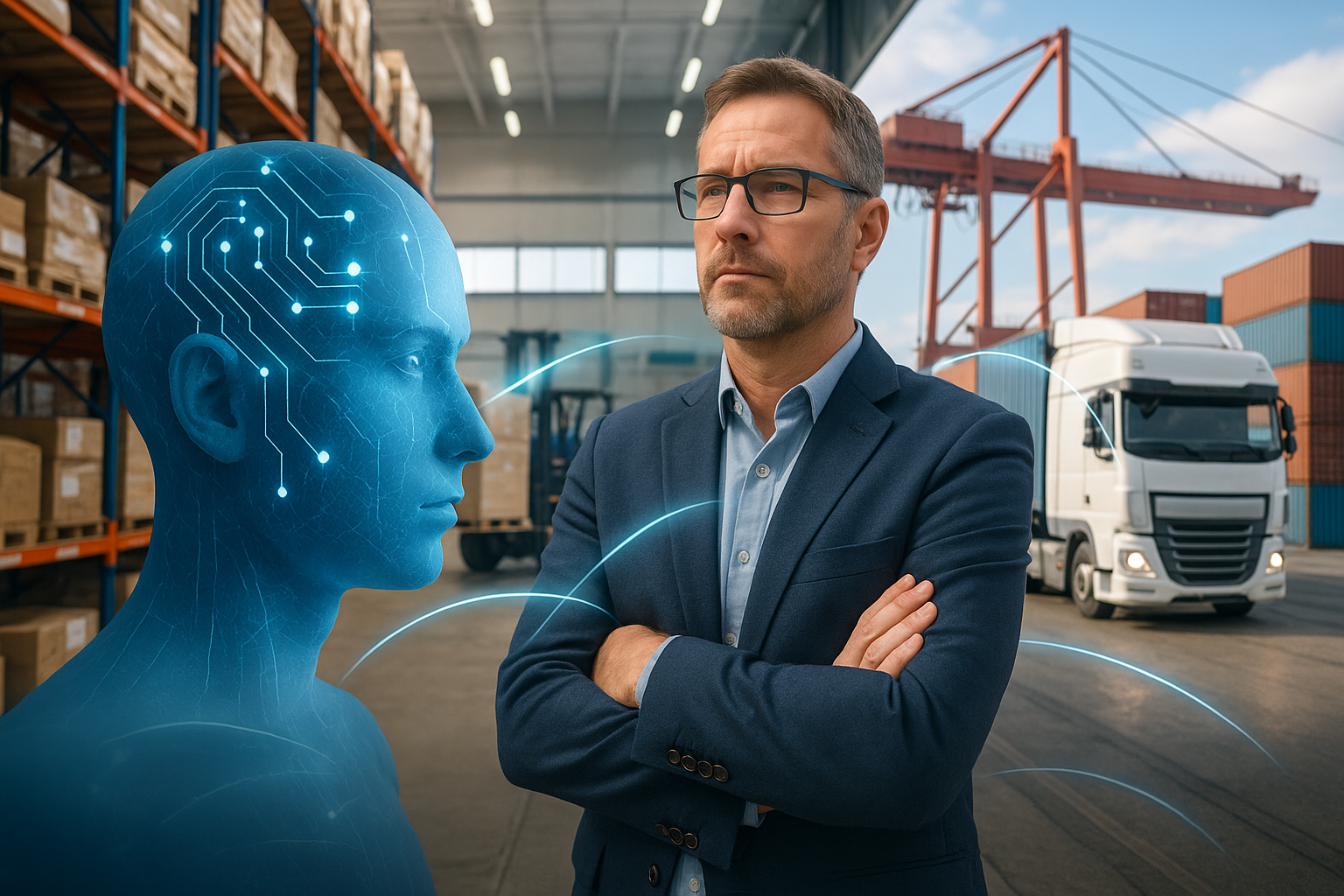
A sweeping transformation in supply chain management is underway, driven by artificial intelligence technologies that are redefining resilience, optimization, and sustainability across industries. A new study titled “Intelligent Supply Chain Management: A Systematic Literature Review on Artificial Intelligence Contributions”, published in the journal Information (2025, Volume 16, Article 399), offers the most comprehensive analysis to date of AI’s evolving role in the supply chain landscape, synthesizing insights from 66 peer-reviewed studies published between 2021 and 2024.
How is AI enhancing supply chain resilience?
The study identifies supply chain resilience as a major area of AI deployment. Machine learning, predictive analytics, neurosymbolic systems, and digital twins are among the leading tools used to enhance visibility, agility, and recovery from disruptions. These technologies have proven critical in forecasting risks, managing supply fluctuations, and mitigating disruptions, especially in crisis scenarios such as the COVID-19 pandemic.
Notably, AI facilitates real-time decision-making, empowers managers with predictive models, and supports proactive strategies such as link prediction for hidden supply chain vulnerabilities. In humanitarian and healthcare supply chains, AI enables rapid response and agility, particularly in remote logistics and inventory planning. Neurosymbolic AI, combining machine learning with symbolic reasoning, also contributes to explainable risk assessments, boosting transparency and trust.
Advanced forecasting techniques, including LSTM models, SARIMA, and reinforcement learning, have demonstrated superior performance in cold-chain logistics and inventory optimization, showcasing AI’s strength in precision-driven operational planning. Additionally, AI-human collaboration frameworks, such as those used in disaster response, blend computational efficiency with contextual judgment, further improving resilience across complex networks.
What AI tools are driving process optimization?
AI’s contribution to process optimization spans demand forecasting, inventory control, logistics planning, and operational automation. The review details how AI improves decision support systems by enhancing accuracy, reducing operational errors, and increasing cost-efficiency. Generative AI and digital twins have emerged as transformative technologies, allowing organizations to simulate operations and proactively adapt to disruptions.
AI-based platforms using blockchain integration, federated learning, and agent-based models streamline data flows, automate procurement, and improve traceability. In sectors like cosmetics and e-commerce, AI supports omnichannel logistics, demand prediction, and fraud detection through CNNs, BiLSTM networks, and multi-agent coordination systems.
Moreover, augmented reality (AR) and AI integration have improved human resource training and real-time performance monitoring, particularly in warehousing and logistics. Explainable AI methods, such as SHAP and LIME, are being applied in decision support systems to make algorithmic outcomes transparent and accountable.
Significant strides are being made in digital transformation initiatives, where AI acts as a catalyst for operational agility and customer-centric services. In e-commerce, AI tools drive product recommendation systems and supply-demand matching, enhancing user experience and responsiveness.
Can AI help achieve sustainable supply chains?
A central contribution of the study is its investigation into how AI supports environmental, social, and governance (ESG) objectives. AI-powered models are increasingly used to reduce emissions, optimize resource use, and enforce ethical sourcing. Techniques such as life-cycle sustainability assessments, carbon neutrality index prediction, and green supplier selection are supported by machine learning, fuzzy logic, and ensemble forecasting.
In circular economy practices, AI enables reverse logistics, remanufacturing optimization, and closed-loop supply chains by forecasting returns and managing reusables efficiently. Studies focusing on fashion and construction sectors demonstrate AI’s ability to prioritize eco-design and reduce environmental impacts through digital twin simulations.
Healthcare and humanitarian sectors also benefit from AI-driven green logistics solutions, such as medical drone delivery systems and responsive routing tools that reduce emissions while improving service delivery in remote areas.
Emerging use cases involve integrating AI with the Internet of Things (IoT) and blockchain to enhance supply chain traceability and regulatory compliance. Generative AI further facilitates automated ESG reporting and scenario modeling, contributing to governance transparency.
What barriers are hindering AI implementation?
Despite the promising results, the review outlines persistent challenges to AI adoption. Key barriers include:
- Data governance and quality: Many organizations lack the infrastructure or willingness to share reliable data.
- Scalability and integration: Difficulty aligning AI systems with legacy supply chain software slows deployment.
- Ethical concerns: Issues of algorithmic bias, lack of explainability, and privacy risks complicate adoption.
- Workforce readiness: A shortage of AI-savvy professionals and resistance to automation hinder integration.
- Lack of real-world validation: Many studies remain conceptual, with limited deployment in industry contexts.
The study emphasizes that to fully harness AI’s benefits, organizations must invest in transparent and inclusive governance frameworks, develop human-AI collaboration models, and adopt context-sensitive strategies tailored to geographic and sectoral differences.
What future directions does the study recommend?
The authors propose a research agenda focused on several key areas:
- Hybrid AI models integrating GNNs, symbolic AI, and machine learning for greater transparency.
- Explainable AI (XAI) frameworks to improve interpretability and trust.
- Digital twins and reinforcement learning for real-time simulation and optimization.
- AI-enabled ESG compliance and sustainability tracking tools.
- Federated learning for privacy-preserving collaboration across supply chain partners.
Additionally, there is a need for cross-regional studies to understand how AI adoption varies globally, and longitudinal research to evaluate real-world impacts over time. The review also highlights the role of ChatGPT-like generative AI in scenario modeling, educational simulations, and communication optimization within supply chain ecosystems.
- FIRST PUBLISHED IN:
- Devdiscourse