Smart microgrids expand globally, but data and cybersecurity challenges persist
Geographically, the research spotlighted Italy, China, and India as the most prolific contributors to smart microgrid literature. China led in citations, while Italy had the highest number of publications. These countries have shown robust deployments of renewable microgrid infrastructure supported by intelligent control systems.
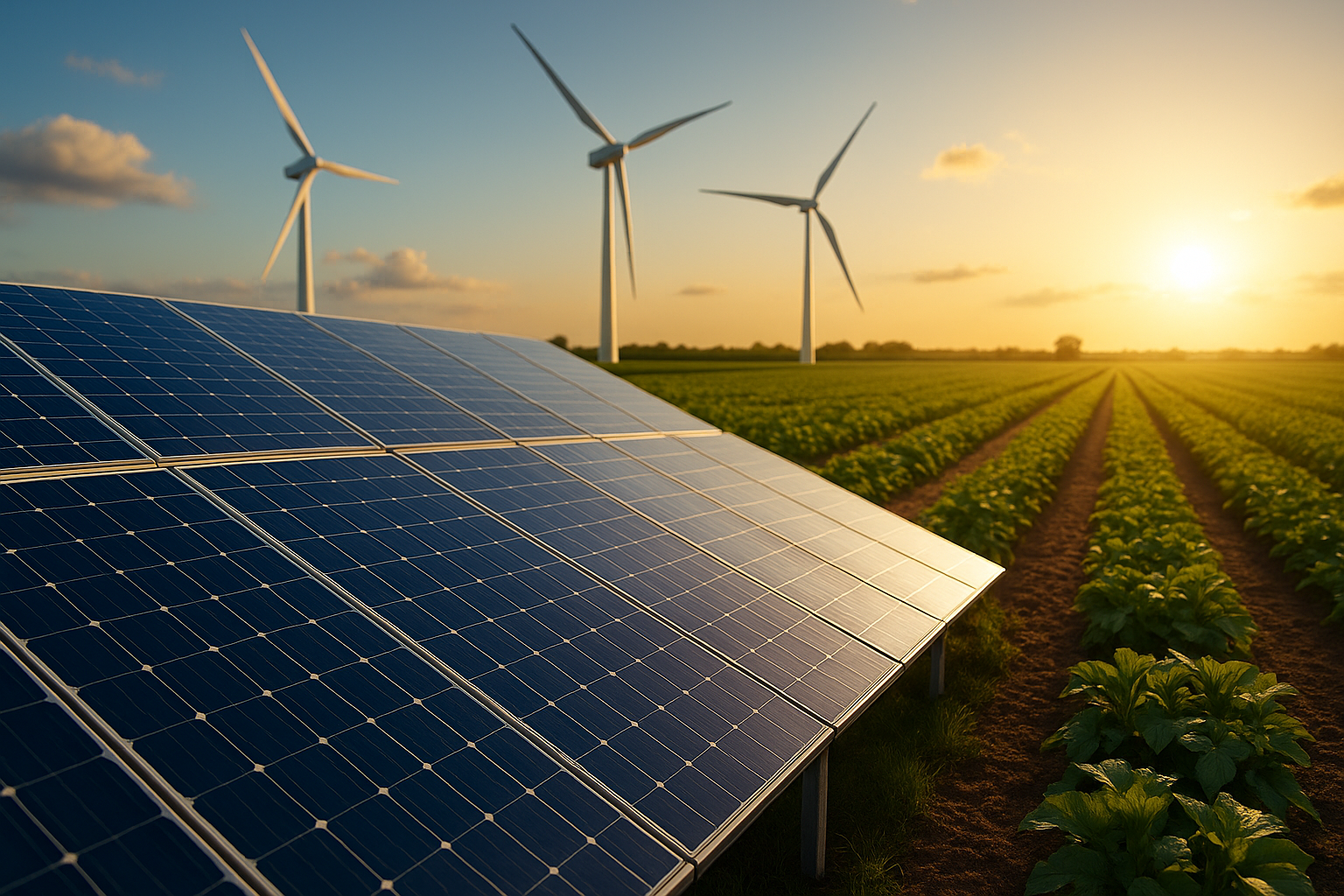
A newly published systematic review outlines the transformative role of smart technologies, particularly artificial neural networks (ANNs) and multi-agent systems (MAS), in optimizing microgrids powered by renewable energy sources. The study, titled “Smart Technologies Applied in Microgrids of Renewable Energy Sources: A Systematic Review,” was published in Energies (Vol. 18, Issue 11, May 2025). As the world races toward decarbonization targets and distributed energy systems, the study highlights both advancements and unresolved barriers that could determine the long-term success of microgrid deployments worldwide.
The study examined 156 peer-reviewed articles published between 2015 and 2023 using the PRISMA method. The analysis identified ANNs and MAS as the most prevalent technologies in renewable microgrid applications. ANNs were primarily used for predictive modeling, particularly in forecasting energy loads and generation variability, while MAS supported decentralized decision-making and coordination within distributed energy networks.
Secondary technologies included clustering algorithms, regression models, and hybrid optimization frameworks. These were applied more sporadically but offered critical support for forecasting, demand-response modeling, and system control under fluctuating conditions.
ANNs were praised for their adaptability and predictive accuracy, with use cases ranging from solar irradiance forecasting to grid stability enhancement. MAS, on the other hand, were deployed in simulations of autonomous agent interactions, allowing distributed energy resource (DER) coordination. Their integration enabled real-time system responses to demand changes, outage scenarios, and variable energy inputs.
Yet, these technologies were not without limitations. ANN models often struggled when reliant solely on historical data, especially under climate volatility or extreme weather conditions. MAS faced challenges in modeling complex human-technology interactions, leading to occasional oversimplification in simulations.
Where and how are these technologies being applied globally?
Geographically, the research spotlighted Italy, China, and India as the most prolific contributors to smart microgrid literature. China led in citations, while Italy had the highest number of publications. These countries have shown robust deployments of renewable microgrid infrastructure supported by intelligent control systems.
Key real-world applications include experimental deployments in Hainan, China, where electric vehicle charging was managed using MAS, and a project in Egypt’s Zafarana region that used ANN models to predict grid behavior under changing climate conditions. In Europe, peer-to-peer (P2P) microgrid architectures using smart control layers were evaluated in Austria, Belgium, and Slovenia to optimize energy distribution and reduce carbon emissions during the 2022 energy crisis.
Smart technologies were particularly valuable in hybrid systems combining solar, wind, and battery storage. They supported functions such as load prediction, voltage regulation, and energy arbitrage, helping balance intermittent supply with fluctuating demand. Still, these benefits were often limited by real-time processing constraints and insufficient energy storage infrastructure in many regions.
Some models attempted to mitigate these issues using edge computing to reduce latency and computational loads, while others incorporated learning-based intrusion detection to address rising cybersecurity concerns in smart energy networks.
What are the risks and technical barriers hindering smart microgrid deployment?
Despite the potential of smart technologies, the study emphasized several unresolved risks. Chief among them was data quality. Microgrid management heavily depends on accurate, granular, and continuous data input, conditions often unmet in remote or resource-constrained environments. The intermittent nature of renewables like wind and solar exacerbates this, introducing unpredictability into load forecasting and system optimization.
Another major concern was computational complexity. High-performing models require significant processing power, which in turn demands substantial energy and cooling resources, creating a paradox for sustainability. This was especially problematic in low-income regions, where infrastructure and funding for high-efficiency computation remain scarce.
Security vulnerabilities also emerged as a critical issue. As microgrids become more connected, they increasingly face risks from cyber threats. The study cautioned that sensitive consumption and generation data must be protected using robust encryption and monitoring frameworks to prevent system tampering or data misuse.
The lack of standardization across platforms, coupled with region-specific deployment challenges, further complicates scalability. Inconsistent internet connectivity, limited sensor availability, and the absence of regulatory clarity in some regions slow down the integration of intelligent systems, despite their technical maturity.
Furthermore, reliance on historical datasets for AI training often leads to poor generalization across different climates or usage patterns, increasing the risk of model overfitting. Researchers pointed out that unless real-time adaptability and contextual intelligence are built into these models, their practical utility will remain constrained.
- FIRST PUBLISHED IN:
- Devdiscourse