Generative AI boosts study efficiency and grades, but raises policy gaps
The system is designed to provide personalized learning paths and real-time assistance. Students access lessons through a smart learning interface where they can interact with the AI assistant. If a question cannot be resolved using the lesson’s knowledge base, the generative AI module dynamically produces a relevant, contextual response.
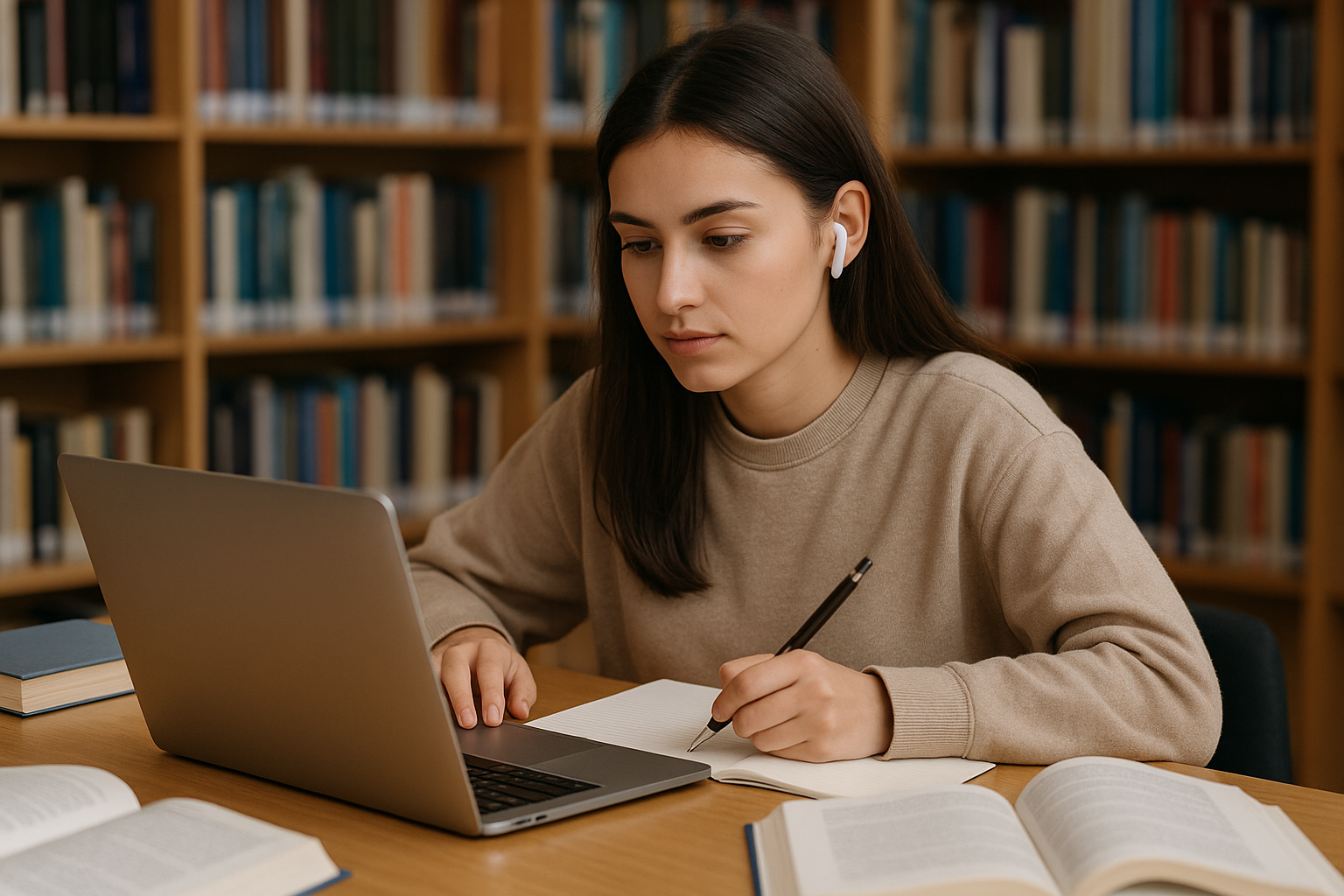
A 2025 European study reveals that students using ChatGPT perceive improvements in study efficiency, assignment quality, and academic performance, driving the design of a new AI-integrated educational system.
A comprehensive quantitative analysis of 4,345 student responses across European universities highlights a decisive shift in student sentiment toward generative AI tools in education. According to the peer-reviewed study, “Transforming Learning with Generative AI: From Student Perceptions to the Design of an Educational Solution,” published in Applied Sciences, most students reported that tools like ChatGPT improved the quality of their assignments, their grades, and study efficiency.
The research utilized a large-scale dataset sourced from a collaborative European survey hosted on Mendeley Data. After extensive data cleaning, researchers identified key findings: students scored ChatGPT’s usefulness in enhancing study efficiency at 3.55 out of 5, improving assignment quality at 3.47, and boosting grades at 3.27. Additionally, the tool’s ability to simulate human language interaction (3.70) and summarize complex information (3.82) received strong approval.
However, the study also exposed gaps in institutional readiness. Students scored their awareness of ethical guidelines surrounding AI usage at just 2.42, signaling the need for better academic frameworks for responsible AI integration. Despite this, researchers noted a positive correlation between frequent ChatGPT use and perceived grade improvement, indicating that increased usage aligns with better academic self-assessments.
Can generative AI be seamlessly integrated into educational systems?
Building on student feedback, the authors conducted a comparative architecture review of four leading adaptive learning platforms: Squirrel AI, Knewton, DreamBox, and a multi-agent system. Notably, none of these existing platforms incorporated a generative AI engine powered by large language models (LLMs). While Squirrel AI utilized an adaptive learning model trained on extensive data, it lacked real-time response capabilities based on generative outputs.
To address this deficiency, the authors proposed a novel architecture that embeds generative AI within a modular learning system. At the core is a virtual AI assistant capable of dynamic content generation, backed by a dual-layered query resolution process: first consulting a curated knowledge base and then invoking a generative AI model, such as LLaMA2, when predefined content is insufficient.
The system is designed to provide personalized learning paths and real-time assistance. Students access lessons through a smart learning interface where they can interact with the AI assistant. If a question cannot be resolved using the lesson’s knowledge base, the generative AI module dynamically produces a relevant, contextual response.
The architecture also includes a teacher interface and monitoring module, allowing educators to track progress, assign tasks, and analyze AI-student interactions. This holistic integration reflects principles from constructionist theory, emphasizing student autonomy and guided exploration.
What risks must be addressed before generative AI becomes a standard in education?
Despite optimism, the study does not overlook the critical limitations of generative AI. The authors address three major risks: hallucinated content, ethical ambiguities surrounding authorship, and the lack of verifiable accuracy in AI-generated responses.
To counter these issues, the system design includes "knowledge grounding" and "structured prompting." Knowledge grounding ensures that generative responses draw exclusively from validated sources, while structured prompting classifies user queries into defined categories, such as explanatory or comparative templates, before passing them to the AI model. This process increases accuracy and contextual relevance.
The authors also recommend using semantic similarity scoring, like cosine distance calculations from Sentence-BERT, to determine whether a question can be answered from the knowledge base or needs generative input. When confidence is low, the system will flag responses as uncertain, adding an essential layer of transparency.
On the policy front, the study urges universities to develop clearer AI usage guidelines and embed academic ethics checks within AI-assisted platforms. The researchers propose detection mechanisms for academic dishonesty and recommend cultural adaptability testing in future iterations of the system.
- FIRST PUBLISHED IN:
- Devdiscourse