Wearable ECGs in home healthcare: Breakthrough or overhyped?
Most commercially available devices, such as the Apple Watch, Samsung Galaxy Watch, and Withings Move ECG, rely on single-lead or limited multi-lead recordings. This restricts their sensitivity in diagnosing conditions like myocardial infarction or acute coronary syndrome, where a full 12-lead ECG is standard. Studies reviewed in the paper found that while these devices excel at identifying atrial fibrillation, their detection of ischemic events remains limited, with diagnostic accuracy improving only marginally with asynchronous multi-lead recordings.
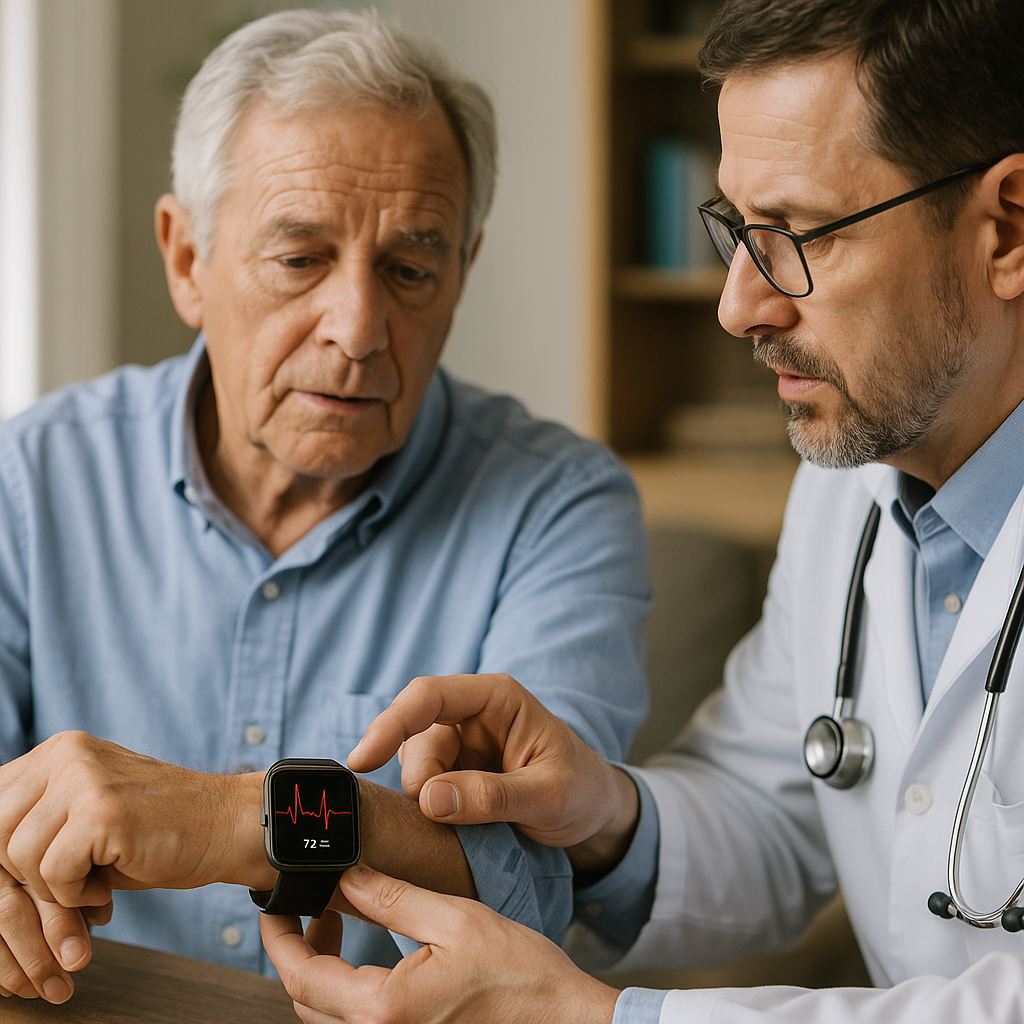
As the healthcare industry shifts toward more decentralized and patient-centered models of care, the question of whether wearable ECG devices are ready for high-stakes clinical roles, such as continuous heart monitoring in Hospital at Home (HaH) programs, has moved to the forefront. A new study, “Are Wearable ECG Devices Ready for Hospital at Home Application?” published in the journal Sensors (2025), critically examines this question through an extensive narrative review guided by a practical framework: the ABCD criteria - Accuracy, Benefit, Compatibility, and Data governance.
The paper analyzes a wide spectrum of commercial and experimental wearable ECG devices, assessing their capacity to substitute or complement standard 12-lead electrocardiograms used in hospital settings. It also evaluates their potential to detect arrhythmias, monitor drug-induced QT prolongation, and even help diagnose acute coronary syndromes, all from a home setting enhanced by artificial intelligence and machine learning.
Can wearable ECG devices match hospital-grade diagnostic accuracy?
The review begins by acknowledging the evolution of wearable ECG devices from simple fitness trackers to complex diagnostic tools. Many now offer capabilities such as real-time arrhythmia detection, heart rate variability assessment, and ST-segment monitoring. However, significant gaps remain in terms of diagnostic equivalence.
Most commercially available devices, such as the Apple Watch, Samsung Galaxy Watch, and Withings Move ECG, rely on single-lead or limited multi-lead recordings. This restricts their sensitivity in diagnosing conditions like myocardial infarction or acute coronary syndrome, where a full 12-lead ECG is standard. Studies reviewed in the paper found that while these devices excel at identifying atrial fibrillation, their detection of ischemic events remains limited, with diagnostic accuracy improving only marginally with asynchronous multi-lead recordings.
The inclusion of AI-powered algorithms in wearables has improved signal interpretation and diagnostic support. For example, convolutional neural networks (CNNs) and other deep learning models have shown impressive results in classifying arrhythmias from single-lead data. Still, even high-performing AI systems are fundamentally limited by the quality of input data, impacted by factors like electrode placement, motion artifacts, and sampling rates. The American Heart Association recommends a 500–1000 Hz sampling rate for adult diagnostics, while most wearable devices operate between 100 and 350 Hz, often compromising granularity.
Are AI-enhanced wearables beneficial and compatible for remote cardiac monitoring?
The study emphasizes the dual promise and challenge of integrating wearable ECG devices into HaH settings. On the benefits front, continuous remote monitoring could drastically reduce hospital readmissions, improve early intervention, and enhance chronic disease management. Devices paired with AI not only support anomaly detection but could also be adapted for personalized models that learn from individual patients' baseline rhythms, paving the way for tailored cardiac care.
Compatibility, however, remains a sticking point. Successful deployment requires seamless integration with hospital electronic health records (EHRs), clinician workflows, and decision-making protocols. While the hardware may be user-friendly for patients, its adoption by clinicians demands rigorous validation, oversight mechanisms, and training. Moreover, trials assessing the use of wearables in pharmacological monitoring (e.g., during COVID-19 treatment with drugs like hydroxychloroquine) showed that wearable-derived QT measurements were reasonably aligned with hospital ECGs, though concerns about noise and data fidelity persisted.
Notably, patient-centric AI strategies that utilize both local and global datasets, such as CNNs trained on wearable-collected ECGs, are emerging as potential game-changers. These systems can monitor for specific arrhythmias and myocardial abnormalities in real-time, using embedded systems like Raspberry Pi and Moto 360 smartwatches. Yet, the trade-offs in real-time responsiveness, energy efficiency, and diagnostic fidelity are not trivial.
How safe and ethically sound are wearable ECGs in a HaH framework?
The study concludes by confronting a critical aspect often overlooked in tech-forward health discussions: data governance and ethical use. The integration of AI-enhanced wearable devices into HaH settings introduces serious privacy, security, and fairness concerns. Data collected from consumer devices are typically image-based, not raw digital signals, complicating transmission and storage. Additionally, most wearables transmit data to proprietary cloud platforms, raising questions about regulatory compliance, patient consent, and medical liability.
Federated learning models, where AI is trained across multiple devices without centralized data aggregation, are explored as a privacy-preserving alternative. However, these models must still overcome issues of data heterogeneity and algorithmic fairness. Socioeconomic disparities in device access, the exclusion of minority populations in training datasets, and reliance on AI outputs without human verification are flagged as ongoing risks.
Ethical reviews included in the study reveal a mixed picture: while wearable ECGs could increase patient autonomy and enable earlier stroke prevention through AF detection, their widespread use without robust educational frameworks could undermine the doctor–patient relationship. False positives and over-reliance on algorithms may lead to anxiety, unnecessary interventions, and even systemic bias.
- READ MORE ON:
- wearable ECG devices
- hospital at home technology
- smart ECG devices for home use
- wearable health tech
- remote patient monitoring devices
- smartwatches for heart monitoring at home
- AI-powered ECG smart devices for remote care
- telehealth cardiac care
- real-time heart monitoring
- personalized AI cardiology
- FIRST PUBLISHED IN:
- Devdiscourse