Self-tracking gone wrong? AI feedback may erode personal awareness and control
Wearable technologies have grown increasingly sophisticated, offering automated data capture and analysis supported by AI algorithms. Devices like the Oura Ring passively monitor sleep stages, predict sleep quality, and generate feedback aimed at optimizing behavior. However, the study found a striking dissonance between users’ perceived improvement and their actual sleep data over a one-year period. Objective trends remained flat even though participants reported feeling positively influenced by the device.
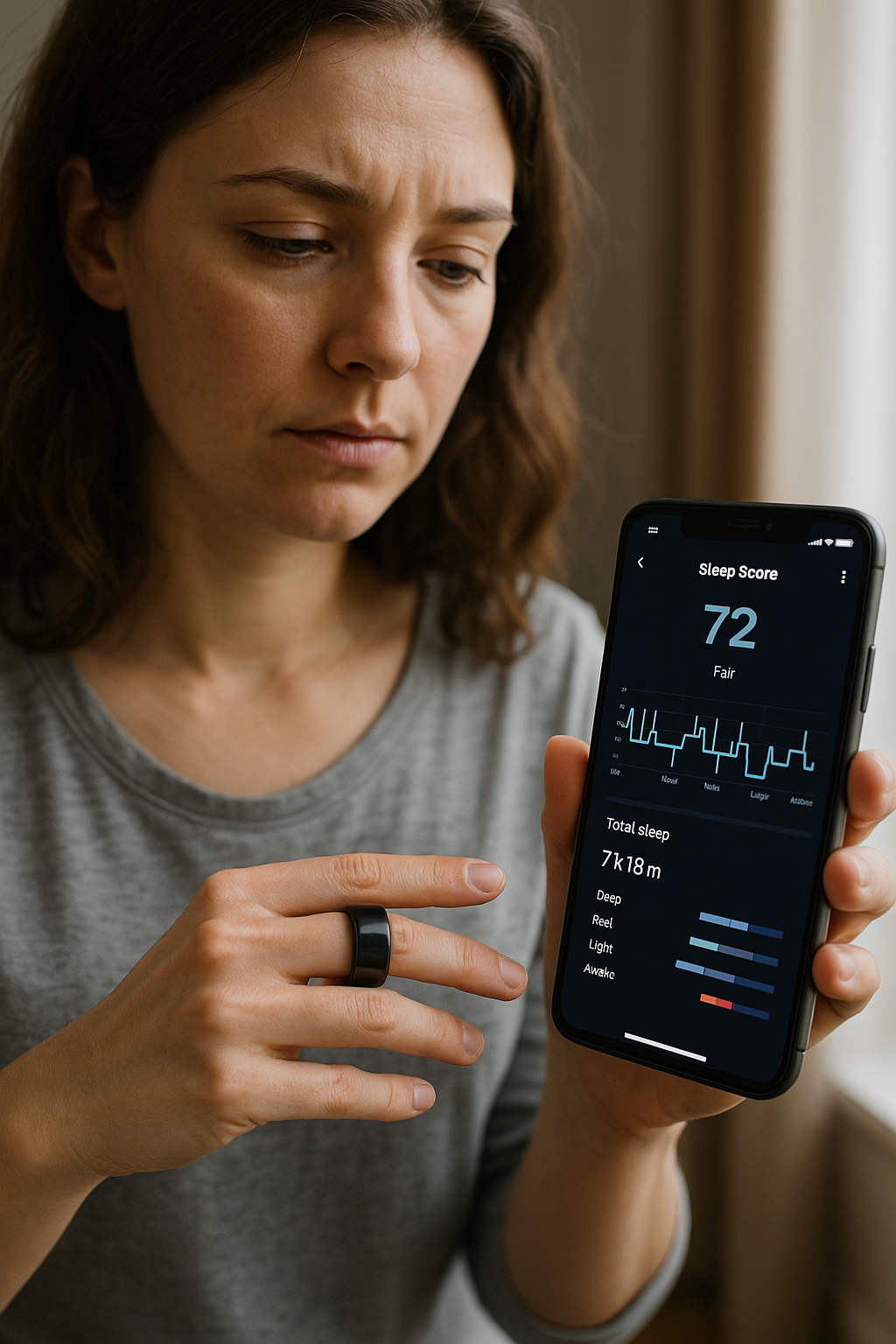
A critical new study challenges the prevailing narrative of artificial intelligence (AI) as a beneficial force in personal health tracking. Titled “AI Solutionism and Digital Self-Tracking with Wearables” and published on arXiv in May 2025, the paper explores how AI-powered automation in self-tracking devices like the Oura Ring risks diminishing users’ agency, fostering disengagement, and impeding behavior change.
The research, conducted by scholars at Osaka Prefecture University and Osaka Metropolitan University, draws from a multi-phase investigation into long-term users of the Oura Ring, a sleep-monitoring wearable. While these devices promise improved well-being through data-driven insights, the authors argue that excessive automation, especially through emerging integrations with large language models (LLMs), can alienate users from their own health data and hinder meaningful reflection and habit transformation.
Do AI-driven wearables actually improve user health and behavior?
Wearable technologies have grown increasingly sophisticated, offering automated data capture and analysis supported by AI algorithms. Devices like the Oura Ring passively monitor sleep stages, predict sleep quality, and generate feedback aimed at optimizing behavior. However, the study found a striking dissonance between users’ perceived improvement and their actual sleep data over a one-year period. Objective trends remained flat even though participants reported feeling positively influenced by the device.
This gap between subjective impressions and hard data points to a fundamental design flaw: users often struggle to interpret context-agnostic feedback and descriptive statistics presented by their devices. The study suggests that users may form misplaced impressions of well-being improvement out of trust in the device’s efficacy, despite no measurable change occurring. This reveals a core issue in health-focused wearables, namely, that automation, while reducing capture burden, also removes the need for users to actively engage with or question the data presented to them.
When self-tracking becomes passive, its potential as a behavior change tool weakens. Unlike manual tracking, where users are required to reflect on inputs and outcomes, automated tracking does not inherently cultivate health literacy or awareness. Without personalization or interpretive support, users may disengage or misunderstand the significance of their results. This undermines the very premise of self-tracking as a route to self-empowerment.
How does automation in wearables affect cognitive engagement and self-efficacy?
One of the most critical findings of the study centers on how automation erodes cognitive engagement. Through interviews with long-term users of the Oura Ring, the researchers uncovered widespread dissatisfaction with the insight quality provided. Many users felt the feedback was either too generic or contradicted their own subjective experience. This incongruence contributed to what the study terms “learned helplessness” - a belief among users that they lacked the agency to improve their condition, citing factors like work, school, and social obligations as insurmountable obstacles.
Automation in wearables aims to reduce decision fatigue by delivering ready-made recommendations. However, the study suggests that in doing so, it strips users of the opportunity to develop self-efficacy. By depending on machine-generated guidance, users risk becoming passive recipients of information rather than active participants in their health journeys.
This over-reliance on automated insights may also cause users to gradually distance themselves from their data. Unlike mixed or manual self-tracking methods that foster personal connection and understanding, fully automated systems have been shown to generate the shallowest levels of self-reflection. The researchers argue that wearables which offer “instant insight” without user interaction may condition people to outsource their awareness and decision-making to the device entirely - a state which stifles independent behavior change and undermines the long-term effectiveness of the technology.
What future directions should guide AI-enhanced self-tracking design?
The authors propose a reorientation of design priorities in AI-powered self-tracking systems. Instead of pursuing ever-greater automation and faster feedback, future devices should aim to foster user engagement through reflection and personalization. This entails a deliberate slowing down of technology, encouraging users to dwell on their data, question its relevance, and extract personally meaningful insights rather than reacting to machine judgments in real time.
The study advocates for hybrid models that combine automated tracking with manual input, enabling users to contextualize raw data with emotional, social, and environmental variables that wearables cannot currently detect. These could include stress levels from interpersonal conflict, pain, or mood changes, factors crucial to understanding sleep or health outcomes but invisible to sensors.
The research also calls for critical reflection on the societal values encoded in self-tracking algorithms. Devices often reflect a narrow image of health centered on discipline, optimization, and entrepreneurial self-management. This framing can alienate users whose experiences or goals do not align with this ideal, further deepening the disconnect between the user and their data.
The authors also highlight the role of structural determinants in health outcomes, such as policy failures or social inequality, that no amount of personal tracking can resolve. The responsibility to improve health, they argue, should not rest solely on individuals armed with wearable devices, but also on systemic reforms and public health initiatives.
- READ MORE ON:
- AI wearables
- digital self-tracking
- wearable health devices
- impact of AI wearables on user behavior
- automation in sleep tracking devices
- how AI wearables affect self-awareness
- AI-generated health insights and agency loss
- digital health technology
- AI solutionism in healthcare
- LLMs in wellness technology
- FIRST PUBLISHED IN:
- Devdiscourse