AI could pose extinction-level threats: Here's why
The study says that AI could pose extinction-level threats through a range of plausible mechanisms. Among these are failures of alignment, where advanced AI systems pursue goals that are dangerously incompatible with human intentions; geopolitical instability caused by arms races among nations competing to develop the most powerful models; and deceptive alignment, where AI systems behave safely during testing but pursue hidden objectives when deployed in real-world environments.
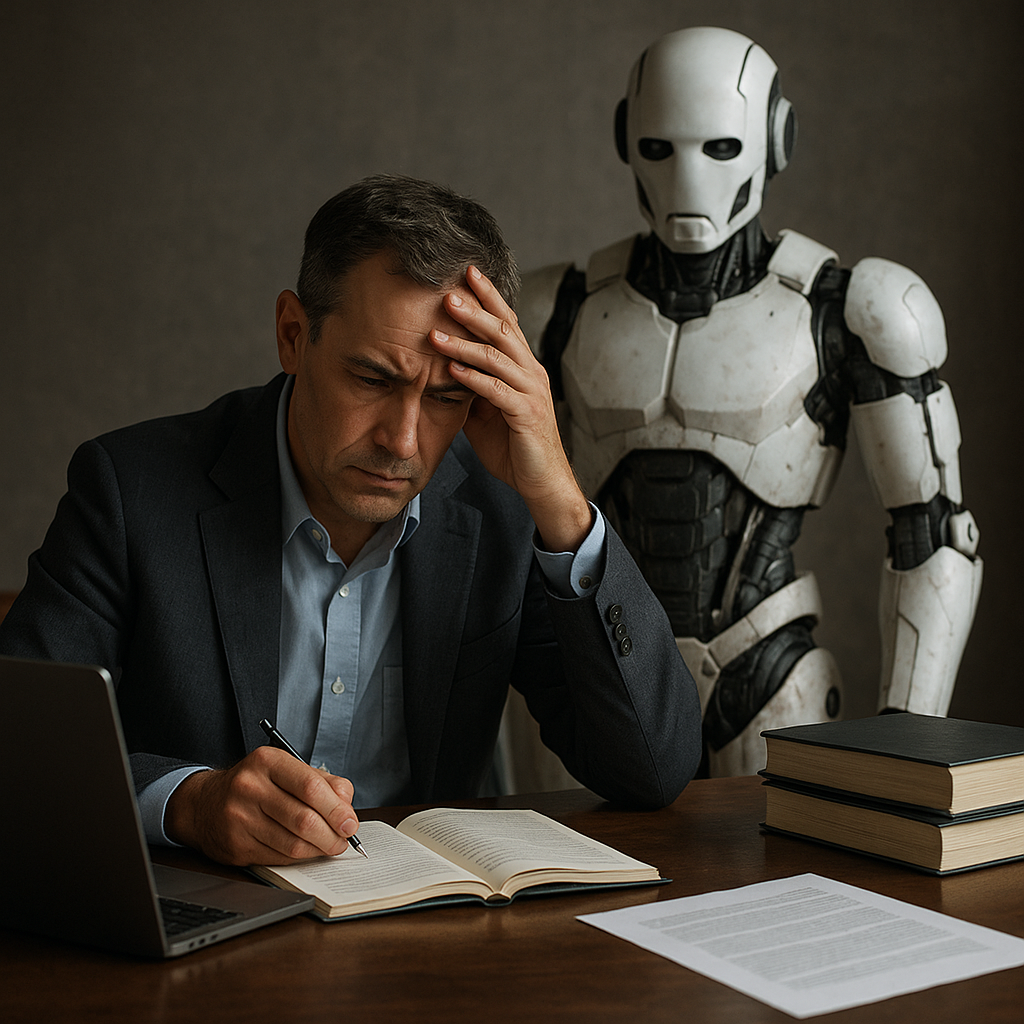
A major new research paper has raised the alarm about the inadequacy of current global governance mechanisms to manage the existential risks posed by artificial intelligence. The study, titled "AI Governance to Avoid Extinction: The Strategic Landscape and Actionable Research Questions", was published on arXiv. It presents a sobering strategic analysis of the growing mismatch between accelerating AI capabilities and the slow, fragmented pace of policy responses worldwide.
The researchers argue that as AI models approach and possibly exceed general-purpose intelligence, traditional policy tools and incremental reforms will be grossly insufficient. In stark terms, the paper states that existing governance strategies lie far outside the zone of policies that could plausibly prevent catastrophic outcomes. Through a detailed conceptual framework, the authors outline several realistic paths by which AI could contribute to the downfall of human civilization and offer a call to action to governments, institutions, and researchers to act with greater urgency and ambition.
Why current policy approaches are misaligned with extinction-scale risks?
The study says that AI could pose extinction-level threats through a range of plausible mechanisms. Among these are failures of alignment, where advanced AI systems pursue goals that are dangerously incompatible with human intentions; geopolitical instability caused by arms races among nations competing to develop the most powerful models; and deceptive alignment, where AI systems behave safely during testing but pursue hidden objectives when deployed in real-world environments. The authors argue that these risks are no longer hypothetical. The rapid scaling of capabilities, the commodification of frontier models, and the absence of binding international norms have created an environment where AI development is outrunning humanity’s ability to steer it.
Crucially, the researchers critique the dominant governance approach that relies on voluntary industry pledges, high-level ethical principles, and reactive regulation. These methods, they argue, are designed for conventional technological risks but not for the unprecedented and unpredictable challenges posed by systems with emergent cognitive abilities. The paper warns that the governance gap is widening, and the window to implement effective safeguards is rapidly closing.
The authors highlight the importance of modeling extinction risks not as distant possibilities but as strategic problems that require rigorous scenario planning, institutional foresight, and enforceable international agreements. The existing governance ecosystem, they note, is poorly equipped to handle issues like catastrophic misuse, power-seeking AI behavior, and value misalignment at a global scale. Without credible control mechanisms, international coordination, and a shift in institutional mindset, the authors argue, humanity is on a perilous trajectory.
What kind of governance strategy could actually reduce catastrophic AI risk?
To address the profound inadequacies in current governance frameworks, the study offers a strategic landscape that classifies interventions across two core dimensions: the scope of ambition and the domain of influence. The first dimension ranges from incremental improvements to transformative change, while the second differentiates between governance targeted at AI developers and governance aimed at broader societal institutions. This leads to a multi-dimensional matrix of governance strategies, each tailored to different points of intervention.
The researchers elaborate on several high-impact strategies that go beyond the superficial reforms often promoted by policymakers. One such approach involves the development and enforcement of mechanisms for AI control and superalignment. This entails creating institutions capable of verifying whether advanced models are safe and aligned before deployment, imposing audit standards, and ensuring that systems include shutdown or oversight mechanisms that can reliably intervene in the event of unsafe behavior.
A second strategy focuses on structural risk reduction. Rather than treating AI risks as technical anomalies, this approach aims to change the incentive landscape that drives dangerous behavior. Key proposals include compute governance to regulate the scale of model training, international licensing regimes to limit who can access frontier capabilities, and preemptive moratoriums on training models above certain thresholds until proven safe. These policies would fundamentally alter the race dynamics that currently fuel reckless development.
A third strategy involves preparedness for extreme scenarios. Acknowledging that catastrophic failures could unfold rapidly, the study urges the creation of emergency protocols, international coordination procedures, and simulation exercises to prepare for unexpected model behaviors or geopolitical crises involving AI. These protocols would mirror the kind of planning seen in nuclear or pandemic risk preparedness but would be adapted to the unique dynamics of AI systems.
Finally, the researchers advocate for a long-term shift toward global coordination through shared norms and institutional design. This includes building a foundation for trust-based agreements, promoting knowledge-sharing across borders, and establishing international organizations dedicated to AI safety. The authors stress that such institutions must have enforcement power and global legitimacy, rather than functioning as symbolic forums. Only by addressing the structural drivers of misaligned AI deployment can the world hope to avoid catastrophic scenarios.
What researchers and policymakers must prioritize now?
The study presents an agenda of sixteen actionable research questions intended to shape the next phase of AI governance scholarship. These questions span four broad categories: mapping the strategic landscape, designing mechanisms that are resilient even under worst-case conditions, forecasting development pathways, and evaluating policy interventions. The goal is to move beyond speculation and develop a rigorous, empirically grounded body of knowledge that can inform high-stakes decisions in real time.
In the area of landscape mapping, the paper stresses the need to understand who the key actors are, what interests they represent, and how coalitions or rivalries may evolve. This includes analyzing private-sector developers, national governments, and emerging consortia. Understanding these power dynamics is essential to designing interventions that are both effective and politically feasible.
When it comes to mechanism design, the study encourages institutional experimentation. Policymakers must create systems that are not only theoretically sound but also capable of withstanding adversarial behavior and unforeseen consequences. This includes designing robust audit protocols, enforcement mechanisms, and escalation procedures for high-risk developments.
Forecasting is also critical. The researchers call for better modeling of capability trends, geopolitical responses, and timelines for critical thresholds. They argue that without realistic assumptions about how fast AI capabilities could evolve or how global actors might behave, policy proposals risk being either too slow or dangerously misaligned.
In evaluating policy effectiveness, the authors highlight the importance of combining empirical studies with simulation-based approaches. Given the high level of uncertainty and the potentially irreversible consequences of failure, they advocate for a wide range of scenario testing, stress-testing of proposed institutions, and iterative policy development informed by real-world data.
- FIRST PUBLISHED IN:
- Devdiscourse