Wheat fields go digital: AI leads precision crop monitoring revolution
Yield prediction is one of the most extensively explored areas in wheat-focused AI research. Using satellite data, UAV imagery, and machine learning algorithms, researchers have developed models capable of identifying complex patterns across vast agricultural landscapes. AI systems, particularly ensemble learning and deep learning models like LSTM, CNN, and XGBoost, have achieved remarkable accuracy, in some cases exceeding 0.95 R² scores. These models analyze variables such as vegetation indices, canopy structure, soil moisture, and climatic trends to anticipate harvest outcomes weeks in advance.
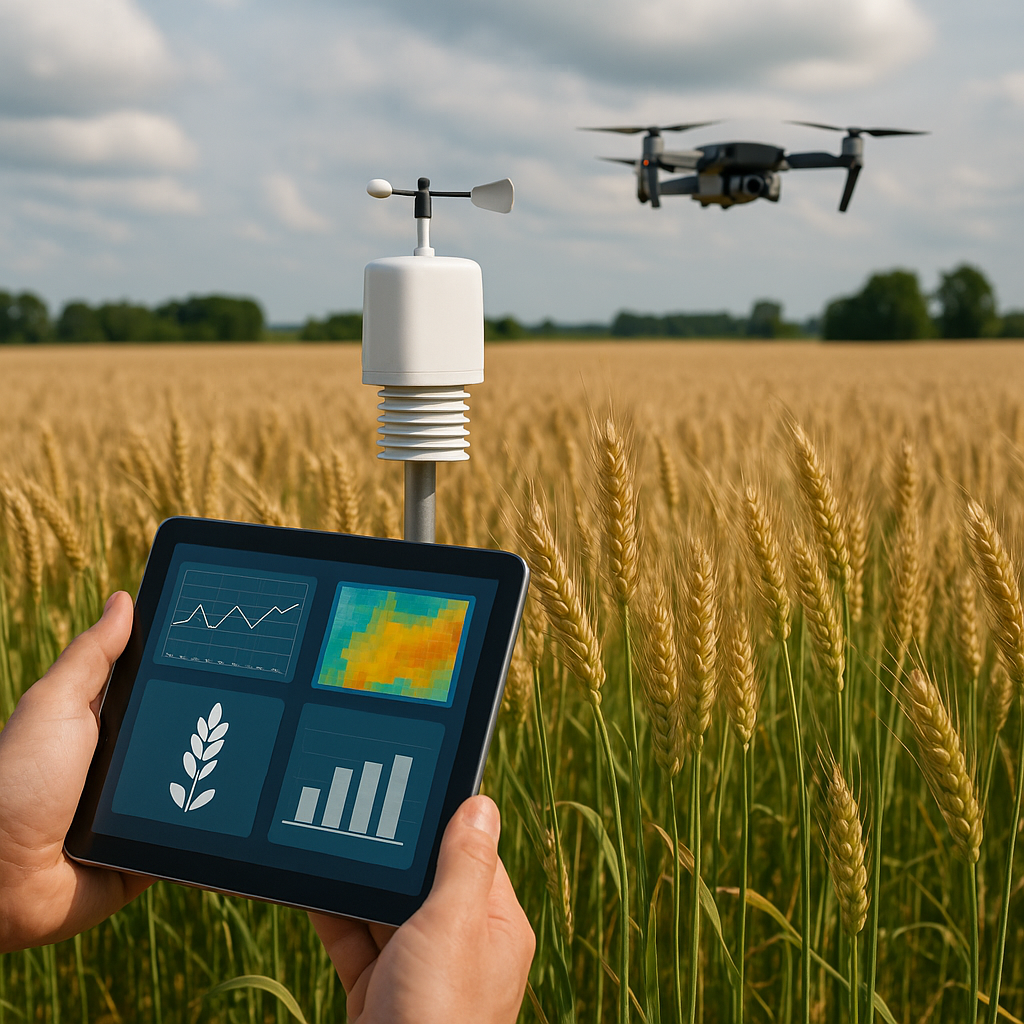
A growing body of evidence underscores the transformative impact of artificial intelligence in modern agriculture, with wheat production emerging as a major frontier. Amid global concerns about food security and climate resilience, the integration of AI in crop management promises to revolutionize how farmers monitor, predict, and respond to environmental and biological challenges in the field.
A comprehensive review titled “A Review of Artificial Intelligence Techniques for Wheat Crop Monitoring and Management” published in Agronomy (2025, 15, 1157) by Jayme Garcia Arnal Barbedo presents a detailed synthesis of over 200 peer-reviewed studies. The study examines how machine learning and deep learning techniques are advancing key areas such as yield prediction, disease detection, and stress monitoring in wheat agriculture. While AI applications in wheat cultivation are making measurable strides, the review also highlights critical limitations, particularly the persistent barriers in dataset quality, model interpretability, and field deployment.
How are AI models transforming wheat yield prediction?
Yield prediction is one of the most extensively explored areas in wheat-focused AI research. Using satellite data, UAV imagery, and machine learning algorithms, researchers have developed models capable of identifying complex patterns across vast agricultural landscapes. AI systems, particularly ensemble learning and deep learning models like LSTM, CNN, and XGBoost, have achieved remarkable accuracy, in some cases exceeding 0.95 R² scores. These models analyze variables such as vegetation indices, canopy structure, soil moisture, and climatic trends to anticipate harvest outcomes weeks in advance.
However, the study identifies a fundamental bottleneck: poor generalizability. Most high-performing models are trained on limited datasets from single geographic regions, making them prone to overfitting and ill-suited for broader application. The review urges future studies to incorporate longer time-series data, diverse agro-ecological zones, and advanced validation protocols like k-fold cross-validation. Additionally, the complexity of tuning deep learning architectures and managing computational costs presents challenges for widespread adoption, especially in low-resource farming contexts.
What are the challenges in disease detection and stress management?
AI has shown enormous potential in automating the detection of wheat diseases and abiotic stresses. Convolutional neural networks (CNNs) dominate this domain, excelling in classifying leaf and ear images of wheat plants affected by rust, blight, and other common diseases. Some models have reached near-perfect accuracy scores (0.98–1.00) using RGB imagery or hyperspectral imaging.
Yet, these advances come with caveats. Many studies rely on small, lab-controlled datasets, leading to overoptimistic results that fail to translate to field conditions. Real-world diagnosis often involves multiple co-occurring diseases and stressors, but most AI models are trained to recognize only one condition at a time. There is also a heavy dependence on high-resolution, annotated images—which are expensive and labor-intensive to acquire.
The review discusses the growing interest in using multimodal data and advanced sensing technologies like hyperspectral imaging for early stress detection. However, the cost and technical expertise required to deploy these systems remain prohibitive. Furthermore, the lack of interpretability in deep learning models hinders trust and transparency, especially when AI decisions guide pesticide use or harvest timing.
What needs to change for AI to scale in wheat agriculture?
Despite the promise of AI, its implementation in wheat farming remains restricted by data quality, model complexity, and real-world feasibility. The study emphasizes that dataset scarcity, particularly the lack of labeled, diverse, and temporally rich data, continues to obstruct progress. Existing datasets often overlook variations in soil quality, management practices, or regional climate, which undermines the robustness of trained models.
Another key limitation is the “black-box” nature of many deep learning models. Their opaque decision-making processes reduce confidence among stakeholders and impede regulatory approval. The review suggests that future AI tools must prioritize model interpretability, ease of deployment, and computational efficiency. Lightweight models optimized for smartphones or edge devices could significantly expand the use of AI tools in low-infrastructure settings.
The study also explores novel approaches such as few-shot learning and semi-supervised learning to tackle dataset limitations, though their application in wheat monitoring is still nascent. More cross-disciplinary collaboration and investment in public datasets are needed to build AI systems that are not only accurate but also transparent, scalable, and equitable across different farming environments.
- READ MORE ON:
- AI in wheat farming
- precision agriculture monitoring
- artificial intelligence in agriculture
- AI-powered wheat monitoring
- wheat crop management AI
- how AI transforms wheat field monitoring
- precision agriculture tools for wheat crops
- smart farming solutions using machine learning
- AI applications in wheat disease detection
- AI-based crop health monitoring in agriculture
- FIRST PUBLISHED IN:
- Devdiscourse