Generative AI beyond human comprehension? Scholars propose new governance model
The first key issue addressed in the study is the conventional wisdom that transparency is the cornerstone of AI accountability. While transparency technologies such as explainable AI and interpretability tools can enhance oversight, the paper stresses that transparency alone is not a sufficient condition for accountability. This is particularly true for generative AI models whose behavior stems from highly dynamic, data-driven, and often opaque internal processes.
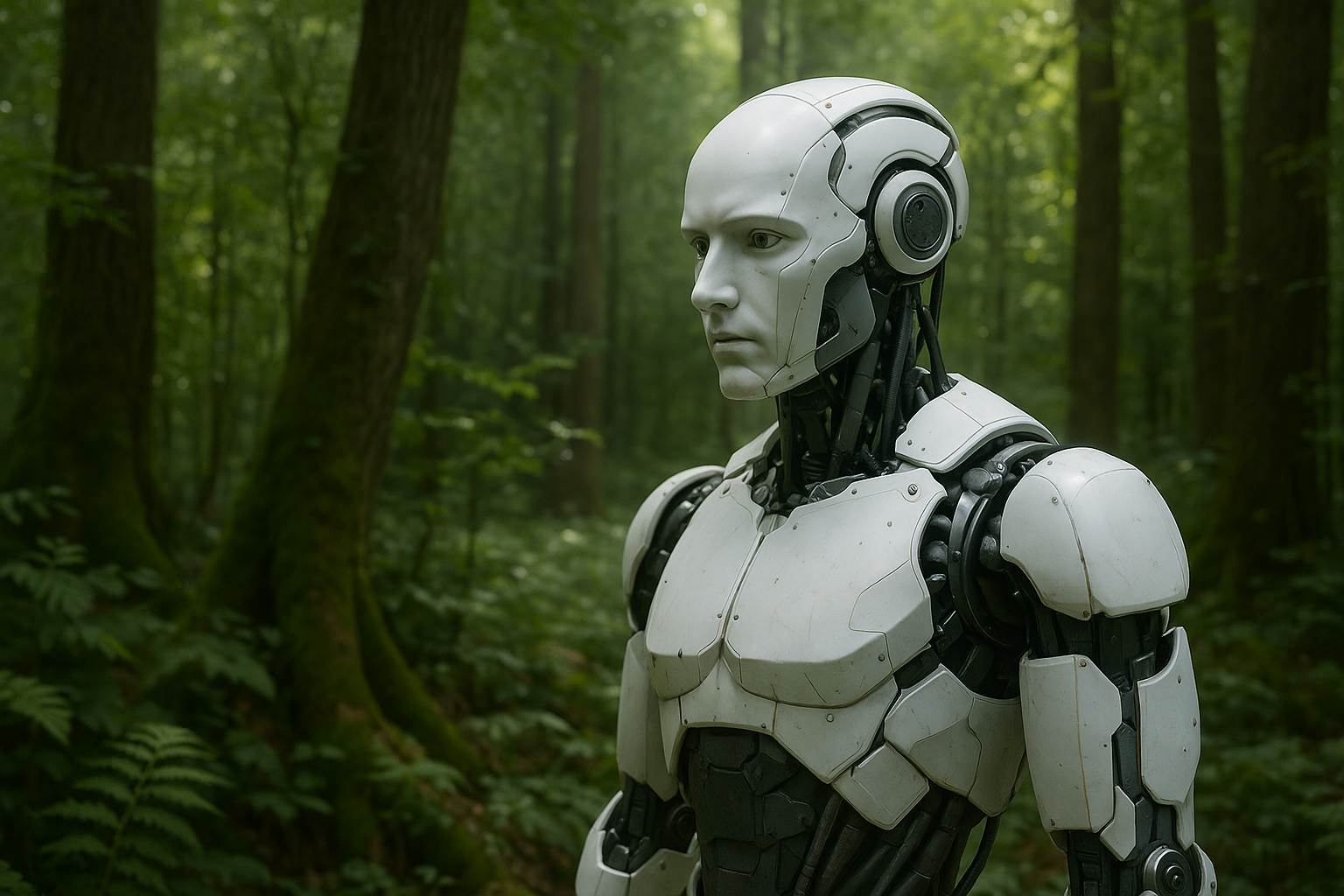
A new academic paper titled “Accountability of Generative AI: Exploring a Precautionary Approach for ‘Artificially Created Nature’” by Yuri Nakao, published for the STAIG’25 conference, lays the groundwork for a revised framework to address the accountability of generative artificial intelligence systems that elude human interpretability. The paper argues that existing expectations for transparency are increasingly insufficient in a technological environment where even AI developers cannot fully explain how models reach their conclusions.
While much of the current policy dialogue around AI accountability is centered on transparency and explainability, the study highlights that these standards are becoming misaligned with the internal complexity of generative models like large language models (LLMs) and diffusion architectures. Rather than pursue unattainable transparency, the paper advocates a shift toward a precautionary principle, similar to the approach used in environmental and pharmaceutical regulation, treating some generative AI systems as metaphorical “artificially created nature.”
Can transparency still guarantee accountability in generative AI?
The first key issue addressed in the study is the conventional wisdom that transparency is the cornerstone of AI accountability. While transparency technologies such as explainable AI and interpretability tools can enhance oversight, the paper stresses that transparency alone is not a sufficient condition for accountability. This is particularly true for generative AI models whose behavior stems from highly dynamic, data-driven, and often opaque internal processes.
Citing current research, the study explains that many generative systems rely on attention-based architectures and operate as black boxes, unable to provide consistent reasoning behind specific outputs. Moreover, real-world usage of generative AI, especially through APIs, has become so abstracted from underlying code and data layers that even expert users cannot clearly distinguish whether a model’s output changes are due to prompt variation or backend updates by developers.
This obfuscation leads to a systemic absence of accountability. Without the ability to trace why a particular result occurred, responsibility for those outputs becomes unassignable. This risks eroding the ethical foundation upon which AI applications are justified, especially when used in high-stakes public decision-making contexts like healthcare, justice, or governance.
Should generative AI be treated as “artificially created nature”?
In response to the collapse of traditional transparency mechanisms, the study introduces a provocative reframing: if humans can’t understand the inner workings of generative AI, then these technologies should be regulated as “artificially created nature.” In other words, AI models should be treated akin to natural phenomena, technologies created by humans but operating beyond full human control or comprehension.
To manage risks under this paradigm, the study proposes a precautionary principle approach. Commonly applied in environmental and medical fields, this principle stipulates that in the face of scientific uncertainty, actions or technologies suspected of posing significant risks should be restricted or rigorously evaluated before widespread deployment. If the risks of generative AI are real but poorly understood, this principle offers a safer and more ethically sound framework than retrospective fixes after harm occurs.
The study underscores that risk-based regulation, as exemplified by frameworks like the EU AI Act, addresses application-level harms but fails to evaluate the foundational risks embedded in the technology itself. Unlike nuclear power, for example, where baseline global agreements on safety, waste management, and proliferation exist, generative AI lacks similar foundational governance. Without this, AI’s growing deployment in public systems risks exceeding society’s ability to manage its consequences.
How can society participate in AI risk governance?
A final but crucial dimension of the paper addresses the role of public participation in shaping precautionary AI governance. Historically, most AI risk assessments and accountability debates have occurred within expert or multi-stakeholder forums. However, the study argues that if AI systems are to be governed as if they were unpredictable natural phenomena, society must create democratic spaces for non-experts to deliberate on acceptable risk levels.
The study advocates for platforms that foster citizen participation, such as consensus conferences, citizen juries, or digital deliberation tools, to help set ethical boundaries and acceptable use cases for generative AI. Drawing parallels to public involvement in bioethics and environmental regulation, it notes that even when technologies are difficult to understand, non-experts have a right to decide which risks they are willing to accept.
Such participatory frameworks are especially important given that generative AI may not produce immediate physical harm but could gradually alter social, economic, or epistemic structures in ways that are difficult to reverse. Decisions about its deployment must therefore incorporate a broader conception of harm and be informed by diverse social perspectives, not just technological feasibility.
Toward a new AI governance paradigm
The study ultimately calls for a dual-track approach. First, where transparency and interpretability are technically feasible, accountability mechanisms should follow the traditional AI governance models emphasizing clarity of design, traceability, and enforceable standards. Second, where transparency fails, especially in large, inscrutable generative models, the precautionary principle should be invoked to prevent uncontrolled risks.
This hybrid framework would better align AI deployment with societal values, allowing both innovation and protection to coexist. Generative AI, the study concludes, may represent the early stages of a broader shift in technological epistemology - where artifacts no longer remain fully knowable or controllable. By recognizing this and adopting an accountability model informed by the same prudence used for environmental or pharmaceutical risks, society can better navigate the coming era of opaque yet powerful technologies.
This reconceptualization not only reframes accountability but redefines how humans relate to machines whose internal logic we can no longer decipher. In doing so, it urges regulators, technologists, and citizens alike to confront the deeper philosophical question: if we cannot understand AI, can we still control it?
- READ MORE ON:
- Generative AI accountability
- AI transparency and risk
- Artificially created nature AI
- Generative AI governance
- AI interpretability crisis
- AI ethics and responsibility
- Governance of unexplainable AI systems
- “Accountability in generative AI systems”
- “Can we regulate AI we don’t understand?”
- FIRST PUBLISHED IN:
- Devdiscourse