Low-cost deep learning models tackle cervical cancer in resource-limited settings
The research details how socioeconomic and geographic barriers severely limit access to preventive healthcare in Brazil’s Amazon region. Women in these areas, many of them indigenous or riverine, face extreme difficulties in obtaining regular Pap smears, often due to long travel distances, lack of transportation, poor health infrastructure, and a critical shortage of trained professionals.
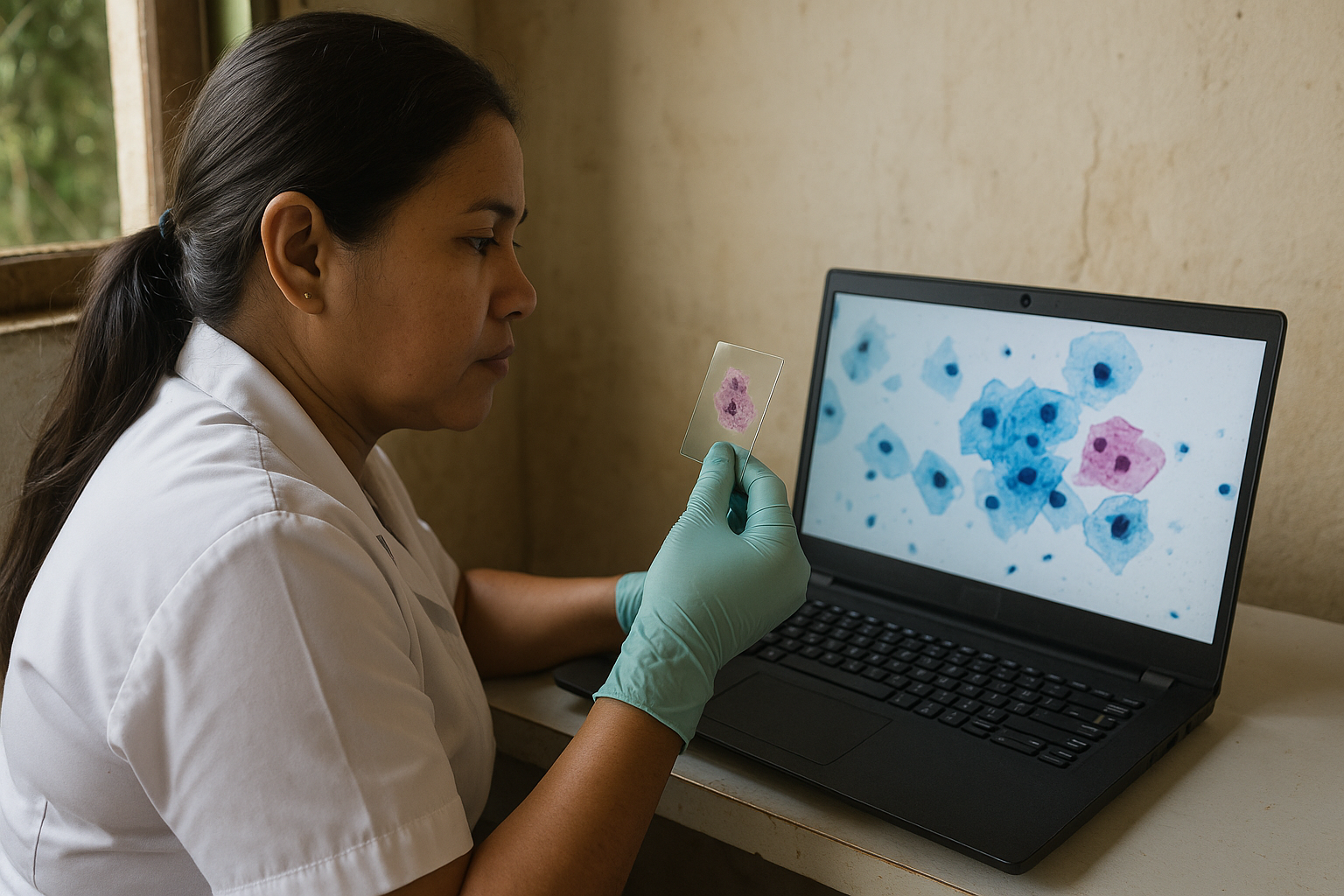
A new review published in the journal Computers titled “Exploring Deep Learning Model Opportunities for Cervical Cancer Screening in Vulnerable Public Health Regions” underscores how artificial intelligence (AI), particularly deep learning models, can revolutionize cervical cancer screening in low-resource settings. The research, authored by Renan Chaves de Lima and Juarez Antonio Simões Quaresma, focuses on underserved areas such as the Brazilian Amazon, where geographic isolation and medical scarcity exacerbate mortality from one of the world’s most preventable cancers.
Cervical cancer remains a leading cause of cancer-related deaths among women in low- and middle-income countries, especially in remote regions. Traditional screening methods like cytology suffer from poor reproducibility, low coverage, and reliance on scarce medical personnel. The study presents deep learning as a transformative tool to address these structural barriers, reduce health disparities, and align with WHO’s global strategy to eliminate cervical cancer as a public health problem by 2030.
What are the obstacles to cervical cancer screening in vulnerable regions?
The research details how socioeconomic and geographic barriers severely limit access to preventive healthcare in Brazil’s Amazon region. Women in these areas, many of them indigenous or riverine, face extreme difficulties in obtaining regular Pap smears, often due to long travel distances, lack of transportation, poor health infrastructure, and a critical shortage of trained professionals.
According to national data cited in the study, two out of every three women aged 30–49 have never been screened for cervical cancer. The Northern region of Brazil, which includes the Amazon, exhibits some of the country’s highest incidence and mortality rates. With only one doctor per 1,000 residents, seven times fewer than in the southern capital regions, timely diagnoses are rare, and advanced lesions are common upon detection.
Traditional cytology also suffers from intrinsic limitations. Its sensitivity varies from 43% to 96%, and accuracy depends heavily on experienced cytopathologists, who are typically unavailable in rural areas. These systemic shortcomings make it difficult to scale prevention efforts using conventional methods alone.
How can deep learning improve screening and diagnosis?
Deep learning models, especially convolutional neural networks (CNNs) and vision transformers (ViTs), have shown high accuracy in image classification tasks relevant to medical diagnostics. In cervical cancer screening, these models have demonstrated potential in automating cell nucleus segmentation, detecting abnormalities, and classifying cytological samples with performance that meets or exceeds human experts.
For example, deep models like ResNet-50 have achieved an area under the curve (AUC) of 0.97 in identifying lesions, significantly outperforming traditional algorithms such as SVMs and Random Forests, which averaged AUCs around 0.82. Moreover, unlike classical models that require manual feature engineering, deep learning models extract features autonomously, offering scalability for large datasets and applicability in high-volume screening contexts.
The study also highlighted lightweight AI models like CCanNet, which require minimal computational resources and achieve over 98% accuracy. Such architectures are ideal for deployment on low-cost devices like smartphones, making them especially suited to areas with limited digital infrastructure.
Despite their promise, these tools must be integrated into existing clinical workflows and validated by healthcare professionals to ensure reliability. Interdisciplinary collaboration is essential to train users, interpret results, and incorporate AI-based diagnostics into public health strategies.
What are the challenges to implementing AI in public health pathology?
While the potential of AI is clear, the study stresses that numerous challenges must be addressed for widespread deployment. These include limited internet connectivity, lack of labeled data from regional populations, and inadequate computational infrastructure. Cultural barriers, patient distrust, and logistical hurdles also hinder the adoption of new diagnostic technologies.
The researchers recommend the use of offline-compatible models and open-source platforms that require minimal infrastructure. Initiatives must align with ethical standards such as the Declaration of Helsinki, the WHO’s ethical AI guidelines, and Brazil’s General Data Protection Law (LGPD). Model development should emphasize privacy, transparency, equity, and accessibility, especially for populations historically excluded from mainstream healthcare advances.
A structured workflow is proposed in the study to support development and deployment. This includes image preprocessing, dataset partitioning, model training and evaluation, and reproducible pipelines. Guidelines like STARD 2015, CONSORT-AI, and MI-CLAIM are recommended to improve model transparency, clinical impact assessment, and reproducibility.
On the regulatory front, the researchers note that Brazil’s national health agency (ANVISA) has introduced Resolution RDC 657/2022, which governs AI as Software as a Medical Device (SaMD). Compliance with these frameworks is essential to ensure safety and efficacy in real-world applications.
- FIRST PUBLISHED IN:
- Devdiscourse