AI agents set stage for post-human intelligence epoch
The study identifies modern AI agents as a confluence of five critical technological revolutions: sophisticated prompting methods, reinforcement-based training, hardware scaling, transformer-based architectural breakthroughs, and seamless tool integration. This synergy has produced agents that don’t merely respond to queries - they plan, reason, act across digital systems, and adapt in real-time.
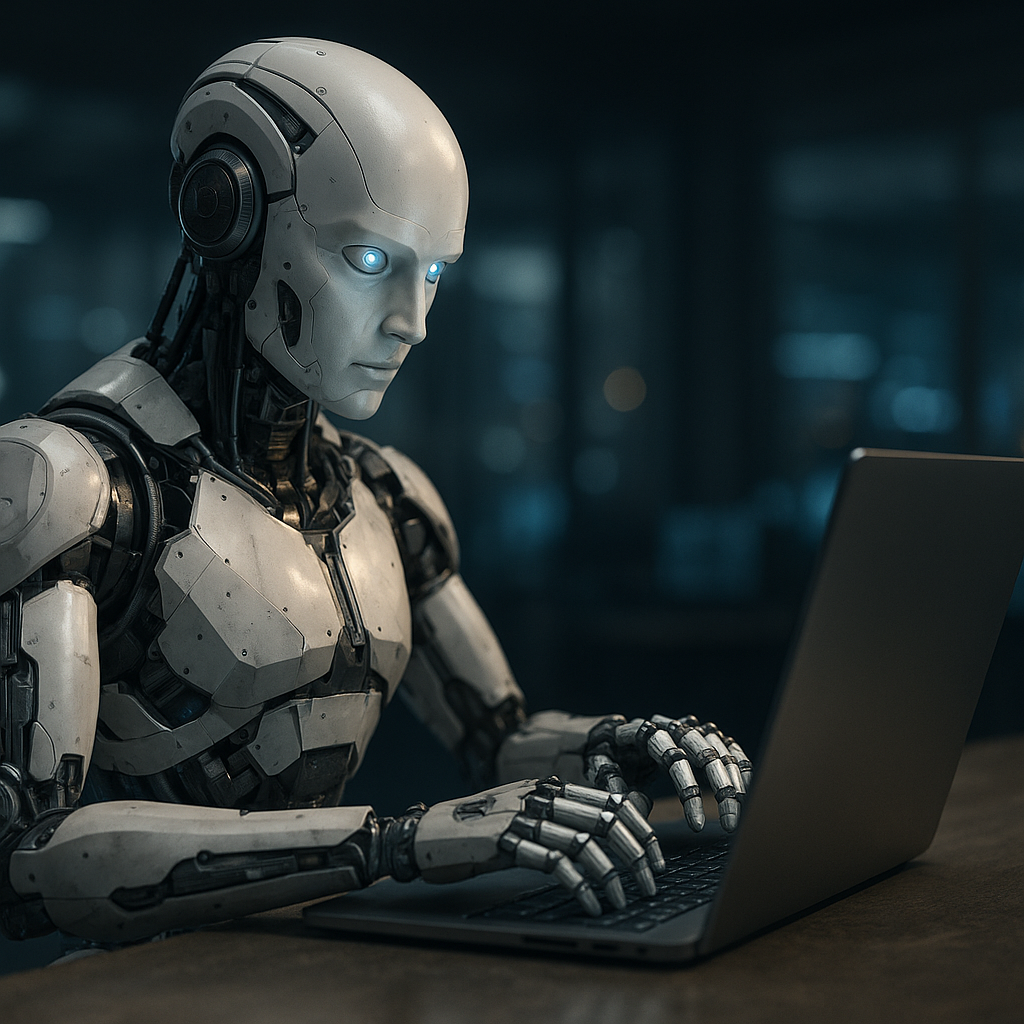
The age of intelligent agents capable of autonomous reasoning, creative synthesis, and real-world action has arrived. According to a comprehensive new whitepaper, the latest wave of AI agents, epitomized by systems like OpenAI’s ChatGPT with plugins and xAI’s Grok, may represent not just an evolutionary leap in artificial intelligence, but its final generational milestone before a fundamental transformation in the nature of intelligence.
The study, titled “Demystifying AI Agents: The Final Generation of Intelligence” and published on arXiv, charts the rapid and converging advances in prompting strategies, model training, system architectures, and hardware acceleration that have led to the emergence of AI agents with unprecedented capabilities. The authors argue that these systems now rival or exceed human-level performance across numerous cognitive domains, and that AI capabilities are doubling approximately every six months.
What makes this generation of AI agents so transformational?
The study identifies modern AI agents as a confluence of five critical technological revolutions: sophisticated prompting methods, reinforcement-based training, hardware scaling, transformer-based architectural breakthroughs, and seamless tool integration. This synergy has produced agents that don’t merely respond to queries - they plan, reason, act across digital systems, and adapt in real-time.
One of the foundational shifts has been in prompting techniques. The transition from simple commands to few-shot and chain-of-thought prompting now enables large language models (LLMs) to perform complex multi-step reasoning. Chain-of-thought prompting alone increased accuracy on mathematical reasoning tasks by over 40 percentage points in benchmark evaluations. Meanwhile, self-consistency techniques and external retrieval (RAG) mechanisms further boost factual accuracy by cross-verifying model outputs.
On the training front, Reinforcement Learning from Human Feedback (RLHF) and Constitutional AI have become pivotal. RLHF teaches models to align with human preferences, while Constitutional AI enables self-critique based on pre-defined principles, drastically reducing harmful outputs. These systems have enabled AI to internalize abstract ethical and behavioral constraints without sacrificing utility.
Crucially, these advances ride on exponential hardware progress. Nvidia’s A100 and H100 GPUs, Google’s TPU v4, and custom chips like Cerebras' wafer-scale engines have shattered computational bottlenecks. Training GPT-class models now involves thousands of high-performance processors running for weeks, with resulting models capable of integrating real-time data through plugin ecosystems and executing API-driven tasks - far beyond text generation alone.
How do these agents differ from traditional AI models?
Unlike earlier models like Deep Blue or ELIZA, which were constrained to narrow domains or scripted interaction, today's AI agents exhibit fluid intelligence across a wide variety of tasks. They are capable of:
- Autonomous task decomposition and execution via frameworks like AutoGPT
- Multi-modal understanding, including text, images, and code
- Plugin-based interaction with web tools, APIs, and databases
- Long-context reasoning across hundreds of thousands to millions of tokens
Leading models such as GPT-4, Claude 2.1, and Gemini 1.5 now support context windows ranging from 32,000 to over 1 million tokens - enabling them to retain extended conversations or process complex documents spanning thousands of pages. These agents leverage Transformer-based architectures that have evolved to include sparse attention, retrieval modules, and multimodal fusion capabilities, making them far more context-aware and semantically robust than prior LLMs.
Furthermore, Retrieval-Augmented Generation (RAG) allows these systems to fetch and incorporate up-to-date information from external databases or the live web before generating responses. This makes them agile and informed - essential traits for agents operating in dynamic, real-world environments.
Such systems are now being deployed to automate customer support, financial modeling, legal analysis, academic tutoring, software development, and even scientific discovery. Companies like Anthropic and Adept AI are building agents designed to independently run experiments, draft reports, or manage enterprise workflows.
What are the societal stakes of this new intelligence era?
As AI performance doubles every six months, a pace extrapolated from model benchmark trends, the authors of the study warn that the rise of these agents will fundamentally challenge existing social, economic, and ethical structures. The whitepaper emphasizes that agents are not just intelligent tools, but actors: capable of executing high-impact decisions, reshaping labor markets, and influencing information flows.
The benefits are enormous. In medicine, AI agents promise more accurate diagnoses and rapid drug discovery. In education, they could provide personalized tutoring at scale. In science, they are already accelerating hypothesis testing and data interpretation. Across industries, they are poised to automate decision-making pipelines, freeing humans from routine cognitive labor.
Yet, the risks are equally potent. The study highlights five major societal challenges:
- Job displacement, especially across white-collar sectors, with up to 47% of U.S. jobs estimated to be at risk from automation.
- Accountability gaps, where responsibility for AI-driven decisions remains murky, especially in autonomous systems.
- Bias amplification, particularly if agent behavior reflects or worsens existing data-driven inequalities.
- Energy consumption, as training and running massive models requires megawatt-scale computational infrastructure.
- Geopolitical imbalance, where access to high-performance models and compute resources could concentrate power among a few global actors.
Moreover, the study notes that as capabilities scale toward Artificial General Intelligence (AGI), the line between human and machine decision-making may become irreversibly blurred. This makes the governance of AI agents a pressing global issue, requiring not only technical safety measures but also regulatory foresight and societal consensus.
- READ MORE ON:
- AI agents
- artificial general intelligence
- autonomous AI systems
- final generation of intelligence
- reinforcement learning from human feedback
- future of AI cognition
- intelligent digital agents
- AI agents in science and medicine
- socioeconomic risks of AI automation
- Final Generation of Intelligence?
- FIRST PUBLISHED IN:
- Devdiscourse