Digital twin and AI combine to streamline industrial pump maintenance strategy
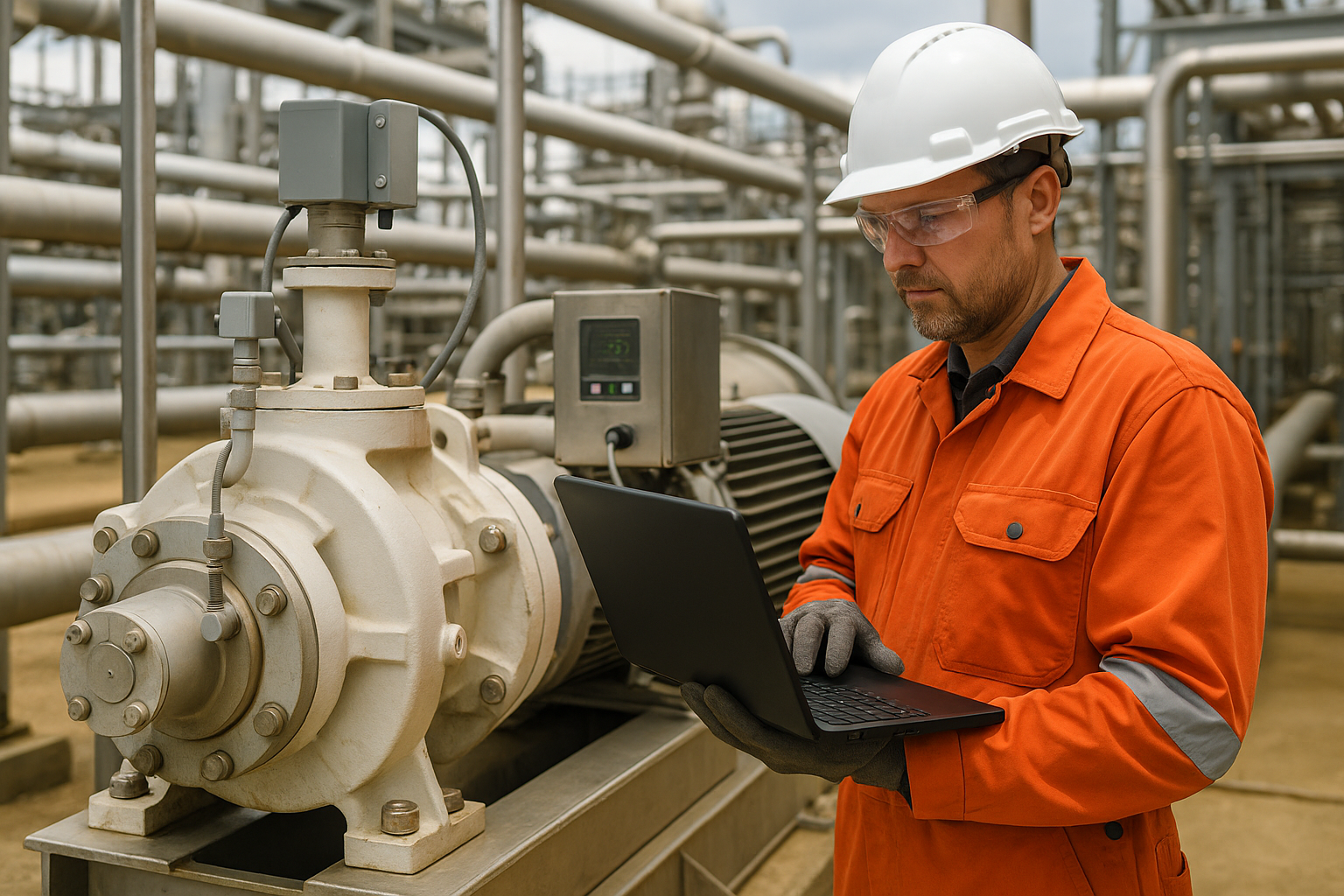
A cutting-edge artificial intelligence-driven solution has demonstrated its potential to transform maintenance planning for critical infrastructure in the oil and gas industry. Published in the journal Processes (2025, Vol. 13, Issue 5), the peer-reviewed study titled “AI-Driven Maintenance Optimisation for Natural Gas Liquid Pumps in the Oil and Gas Industry: A Digital Tool Approach” details the development, implementation, and validation of a digital tool that significantly reduces maintenance costs and downtime for Natural Gas Liquid (NGL) pumps.
How does the AI tool overcome shortcomings in traditional maintenance systems?
NGL pumps are essential in oil and gas operations, particularly in fractionation processes. Failures in these rotating assets can lead to costly 24-hour production halts. Historically, maintenance strategies for such equipment relied on static schedules or expert intuition, methods that neither predict failures accurately nor optimise maintenance intervals effectively.
The newly proposed AI-based methodology integrates dynamic optimisation, condition assessment, and rule-based diagnostics within a unified system. By utilising both limited historical data and real-time sensor inputs, the digital tool calculates the Mean Time Between Failures (MTBF), predicts the Remaining Useful Life (RUL), and identifies optimal maintenance intervals on a component-specific basis.
Unlike many predictive models, which require large volumes of training data or address only single failure types, this tool functions effectively even with sparse datasets. Its core innovation lies in combining real-time input with adaptive algorithms to support both proactive scheduling and reactive diagnostics across multiple components.
What key features enable predictive and cost-efficient maintenance?
The AI-powered platform comprises several functional modules that simulate a digital twin of pump operations. These modules perform critical tasks including:
- Optimising maintenance routines using reliability and cost trade-offs.
- Prioritising components with Failure Mode and Effects Analysis (FMEA) based on their criticality.
- Evaluating component health using vibration and leakage sensor data.
- Estimating RUL, particularly for bearings, using a vibration-based formula refined by a continuously updated linear regression model.
- Generating diagnostic outputs with rule-based and fuzzy logic algorithms to isolate fault causes and recommend actionable maintenance strategies.
For instance, bearings are evaluated using ISO 20816 vibration thresholds, while mechanical seal conditions are assessed through fuzzy logic models informed by pressure readings and operational safety guidelines. Diagnostic decision trees guide users through possible fault origins, such as poor lubrication or casing misalignment, and suggest precise maintenance steps.
Two scheduling strategies were evaluated: Route 1 (component-level optimisation) and Route 2 (integrated scheduling). Route 2 aligns maintenance actions across components during shared downtime windows. The unified approach dramatically reduced operational disruptions and associated costs, cutting total maintenance expenditure by over 80% during the 10-year planning horizon through 2034.
How was the tool validated and what did industry experts say?
Validation was conducted in two phases. First, the tool was tested on real-world data from a Saudi Aramco facility's pump unit (G221), evaluating its performance using actual failure and sensor records. This test confirmed the accuracy of MTBF calculations and RUL estimations, with results closely aligning with OEM expectations and industry standards.
For example, the inboard bearing showed a calculated MTBF of 6.5 years versus an OEM baseline of five years, while mechanical seals yielded estimates of 3.2 years, matching API 682 recommendations. The cost-benefit analysis revealed Route 2 reduced downtime-related losses from over $15 million to just $2.5 million, primarily by consolidating shutdowns.
Second, a structured survey involving 15 maintenance experts from major Middle Eastern oil companies including Saudi Aramco and SABIC evaluated the tool’s logic, usability, and industrial relevance. Over 90% of respondents strongly agreed on the necessity of AI deployment for NGL pump maintenance. They also confirmed the soundness of the technical assumptions used in RUL estimation and fuzzy logic modelling for diagnostics.
While Saudi Aramco staff praised the tool’s simplicity and Excel-based interface, SABIC engineers suggested that integration with enterprise systems like SAP could enhance its adoption. The diagnostic module, which identifies early-stage failures and recommends targeted interventions, was also highly regarded, particularly for its utility in reducing reliance on manual inspections.
- FIRST PUBLISHED IN:
- Devdiscourse