Scaling Data Annotation: Challenges and Strategies for AI Companies
AI companies face challenges in scaling data annotation. Learn how to optimize workflows, control costs, and maintain accuracy.
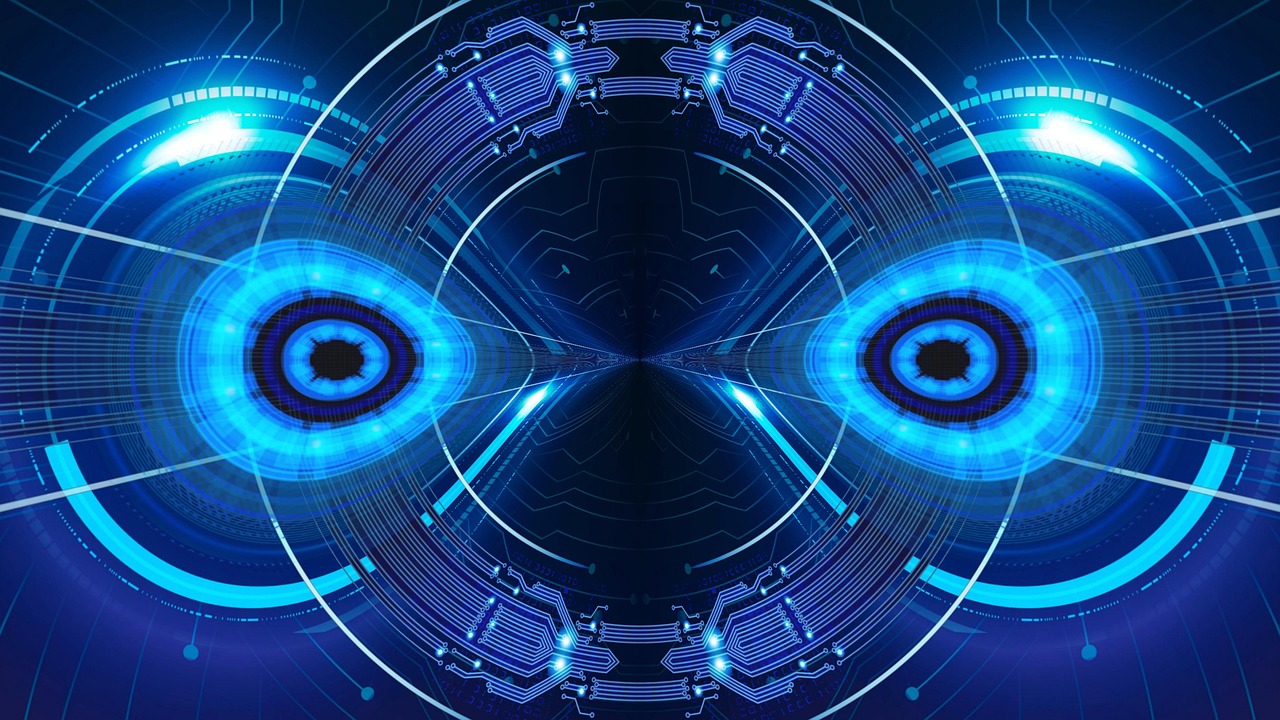
The Challenges of Scaling Data Annotation for AI Companies
High-quality labeled data is key for training AI models. However, scaling data annotation presents major challenges. As datasets grow, ensuring accuracy, maintaining speed, and managing costs become increasingly difficult.
Using in-house teams or data annotation companies can help. However, problems arise as annotation needs grow. AI companies may face slow growth, higher costs, and uneven results without a solid strategy.
Common Challenges in Large-Scale Data Annotation
What is data annotation’s biggest challenge for large-scale projects? Scaling data annotation isn’t just about hiring more people. As projects grow, AI teams face delays, rising costs, and quality issues. Workforce management, tools, and security become major challenges.
Workforce Challenges
Managing a growing team of annotators brings hurdles in hiring, training, and quality control.
Hiring and Training at Scale
Finding and training annotators takes time.
- Large projects need domain experts, but skilled annotators are hard to find
- Language and cultural differences cause inconsistencies
- High turnover slows projects and lowers quality
Fix: Use standardized onboarding and ongoing training to keep teams aligned.
Maintaining Consistent Quality
Bigger teams make quality control harder.
- Different annotators may label data inconsistently
- Fatigue increases mistakes
- Manual reviews slow the process, but skipping them hurts accuracy
Fix: Use multi-step reviews, peer checks, and AI-assisted quality control.
Tooling Issues
Inadequate tools can hinder efficiency and collaboration as annotation projects expand.
Basic Annotation Tools Fall Short
Simple tools work for small projects but struggle at scale.
- Slow processing of large datasets
- Inefficient interfaces increase labeling time
- Harder collaboration for remote teams
Fix: Upgrade to scalable tools with automation, role-based access, and AI integration.
Balancing Automation and Accuracy
AI-assisted labeling speeds up work but has risks.
- Pre-labeled data may be biased
- Over-reliance on automation reduces oversight
Fix: Use AI for pre-labeling but have humans verify accuracy.
Security and Compliance Risks
Handling sensitive data and meeting regulatory demands become trickier at scale.
Handling Sensitive Data
Many AI teams outsource to data labeling companies, increasing security risks.
- Medical and financial data need strict access control
- Weak security policies lead to breaches
Fix: Use encrypted storage, role-based access, and strict compliance policies.
Staying Compliant with Regulations
Laws like GDPR and HIPAA impose strict data rules.
- Global teams may not follow local regulations
- Compliance is harder with multiple vendors
Fix: Work with a trusted data annotation company that ensures compliance.
Strategies to Overcome Data Annotation Scaling Issues
Scaling data annotation requires more than just adding resources. AI teams must balance speed, quality, and cost while keeping workflows efficient. Here’s how to tackle common challenges.
Balancing Quality and Speed
Maintaining accuracy without sacrificing pace is critical for large-scale annotation success.
Use a Tiered Review System
Mistakes increase with scale. A tiered approach helps maintain accuracy.
- First pass: Annotators label data.
- Second pass: Reviewers check for errors.
- Final check: AI-assisted validation flags inconsistencies.
This reduces human errors without slowing down the process.
Prioritize Data with Active Learning
Not all data needs the same level of review. Train your AI models on high-quality samples and let it identify low-confidence cases for human review. This approach speeds up annotation while improving model performance.
Automating Repetitive Tasks
Automation can streamline repetitive labeling work while preserving human oversight.
Pre-Label Data with AI
AI can handle repetitive tasks like object detection or text classification. Humans only review and correct, instead of starting from scratch. This cuts annotation time and improves efficiency.
Leverage Weak Supervision
Instead of manually labeling everything, use existing datasets and rules-based automation. AI applies broad labels and humans refine the edge cases. This approach reduces workload while keeping quality high.
Building and Managing a Scalable Workforce
A well-trained, flexible workforce is key to meeting growing annotation demands.
Centralized vs. Distributed Teams
Different models work for different needs:
Model |
Pros |
Cons |
Centralized |
Easier training and quality control |
Higher costs, limited scalability |
Distributed |
Scales quickly, lower costs |
Harder to standardize quality |
Most AI companies use a hybrid approach — keeping critical tasks in-house while outsourcing bulk annotation.
Upskilling Annotators
Instead of constantly hiring, invest in training existing teams. Teach annotators advanced labeling techniques and provide feedback loops to improve accuracy. This reduces turnover and improves long-term efficiency.
Choosing the Right Data Annotation Platform
Selecting the right tools can make or break efficiency and quality at scale.
Key Features to Look For
Scaling requires better tools. An ideal platform should offer:
- AI-assisted labeling
- Role-based access
- Quality control workflows
- API integration
In-House vs. Outsourced Solutions
- In-house: More control but higher costs
- Outsourced: Faster scaling but potential quality risks
A mix of both is often the best approach.
Avoiding Cost Pitfalls in Large-Scale Data Annotation
Scaling data annotation can quickly become expensive if not managed properly. AI companies often underestimate the hidden costs of growing annotation operations. Here’s how to keep costs under control while maintaining quality.
Hidden Costs of Scaling Annotation
Unexpected expenses like turnover and inefficiencies often catch teams off guard.
High Turnover and Training Expenses
Hiring more annotators isn’t enough — you need to train and retain them. High turnover leads to frequent onboarding, which slows down projects. It also causes inconsistent annotation quality as new workers learn the process. Not to mention the increased management overhead to oversee shifting teams.
Solution: Invest in structured training and career progression for annotators to reduce churn.
Inefficiencies in Annotation Workflows
Poorly optimized workflows lead to wasted time and effort. Common issues include redundant manual tasks that could be automated. Also, unclear guidelines causing annotators to redo work. And the lack of real-time feedback, leading to repeated errors.
Solution: Use AI-powered pre-labeling, clear documentation, and real-time feedback loops to speed up work without sacrificing accuracy.
Scaling Infrastructure Without Overspending
Larger datasets require better storage, processing power, and security. If not planned correctly, costs can spiral out of control.
Solution:
- Use cloud-based annotation platforms to scale resources on demand.
- Optimize storage by archiving completed annotations and only keeping active datasets.
- Choose data annotation companies that offer cost-effective solutions for bulk labeling.
Managing Edge Cases in Large-Scale Data Annotation
As datasets grow, so do the number of complex, ambiguous, or rare cases that don’t fit standard labeling rules. These edge cases can seriously impact AI model performance if not handled correctly.
Why Edge Cases Matter
- AI models trained on clean, predictable data struggle with real-world variability.
- Mislabeling rare cases can introduce bias and reduce accuracy.
- Edge cases often require subject matter expertise, making them harder to annotate at scale.
How to Handle Edge Cases Efficiently
- Create a dedicated review team for difficult annotations to ensure consistency.
- Use dynamic labeling guidelines that evolve based on edge case patterns.
- Leverage AI-assisted annotation to flag uncertain cases for human review instead of manually reviewing everything.
By planning for edge cases, AI teams can improve model generalization and prevent costly retraining.
Solution: Use AI-powered pre-labeling, clear documentation, and real-time feedback loops to speed up work without sacrificing accuracy. Additionally, consider integrating an AI-powered customer service tool to enhance labeling efficiency and ensure better quality control across annotation workflows.
Final Thoughts
Scaling data annotation is about working smarter. Without the right strategy, AI teams face rising costs, slow development, and inconsistent data quality.
AI companies can maintain high accuracy by using automation. They can also implement structured workflows and scalable annotation platforms. This helps them manage larger datasets effectively. Investing in the right tools and training helps keep things running smoothly. This way, annotation becomes a strength, not a hurdle.
(Disclaimer: Devdiscourse's journalists were not involved in the production of this article. The facts and opinions appearing in the article do not reflect the views of Devdiscourse and Devdiscourse does not claim any responsibility for the same.)