From honey to meat: How AI is exposing hidden food frauds with 98% accuracy
Machine learning (ML) and deep learning (DL) algorithms now enable robust, non-invasive authentication. Tools such as convolutional neural networks (CNNs), support vector machines (SVMs), and random forests (RFs) are commonly applied to datasets derived from spectroscopic analysis, chromatographic profiles, and digital images. For example, CNN-based systems have achieved over 97% accuracy in identifying adulteration in red meat using hyperspectral imaging (HSI).
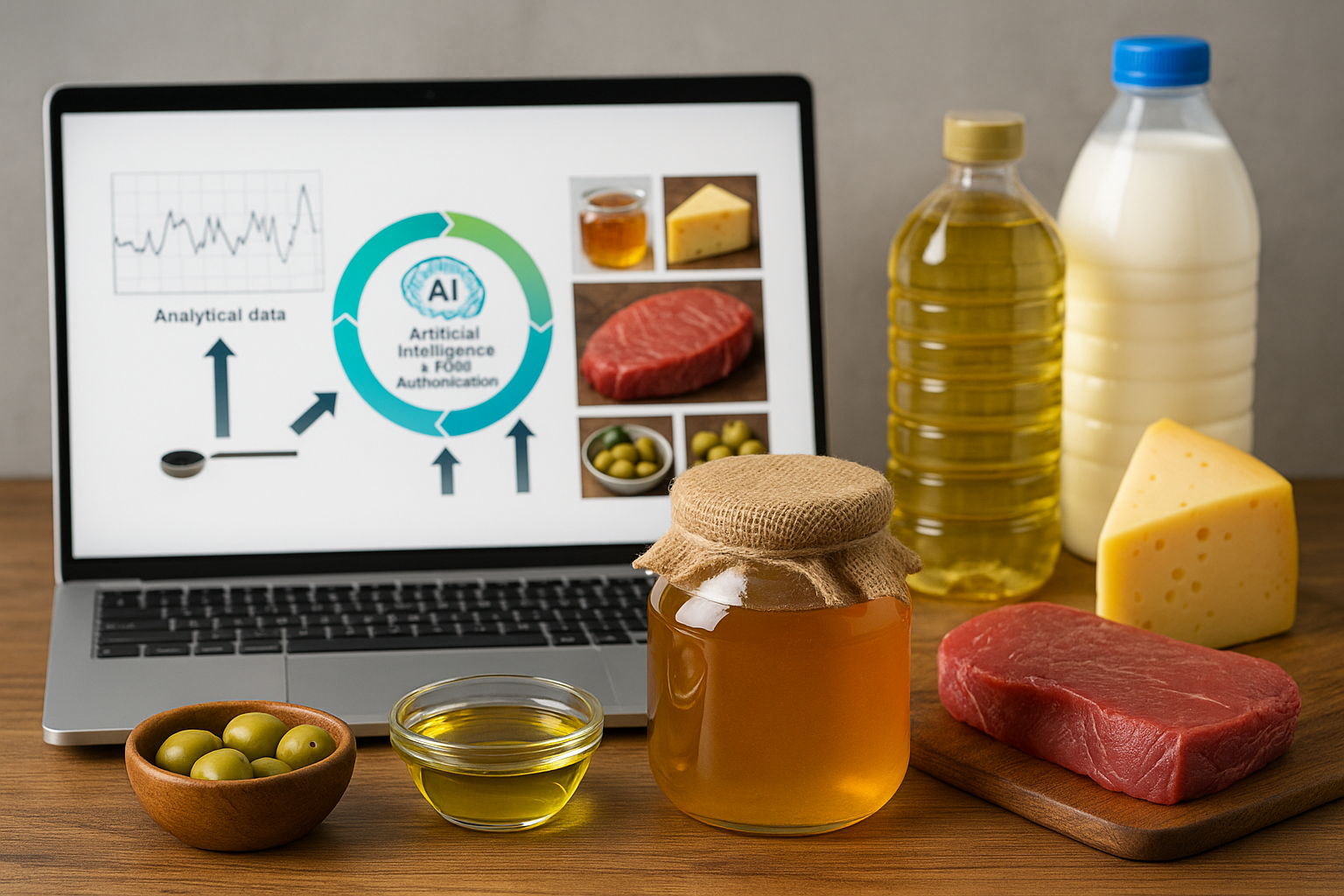
Artificial intelligence is revolutionizing food authentication practices, offering faster, more accurate, and cost-effective tools to detect fraud across global food supply chains. According to the peer-reviewed study “The Journey of Artificial Intelligence in Food Authentication: From Label Attribute to Fraud Detection” published in Foods, AI methods are increasingly deployed to verify origin labels, detect adulteration, and build portable, user-friendly recognition systems that minimize reliance on traditional lab analysis.
The review, authored by researchers from the National Institute for Research and Development of Isotopic and Molecular Technologies and Babeș-Bolyai University in Romania, synthesizes AI’s expanding role in food authenticity control. It presents compelling evidence of AI’s superiority in processing complex datasets, particularly those involving image, spectral, and chemical signals, to detect fraudulent practices like ingredient substitution and mislabeling in products such as honey, oil, dairy, and meat.
How is AI replacing traditional authentication in food systems?
The study outlines how artificial intelligence has matured beyond its early role in classification tasks, where it worked alongside statistical models to verify label attributes like geographical or botanical origin. Over time, AI’s ability to manage large, multivariate datasets and detect nuanced patterns, especially in image and spectral data, has empowered the food industry to adopt it as a primary tool for quality control.
Machine learning (ML) and deep learning (DL) algorithms now enable robust, non-invasive authentication. Tools such as convolutional neural networks (CNNs), support vector machines (SVMs), and random forests (RFs) are commonly applied to datasets derived from spectroscopic analysis, chromatographic profiles, and digital images. For example, CNN-based systems have achieved over 97% accuracy in identifying adulteration in red meat using hyperspectral imaging (HSI).
Importantly, AI removes the subjectivity associated with human visual assessment and reduces dependence on expert operators. The models can automatically extract features from raw data, digital images, infrared spectra, or thermal readings, without handcrafted inputs. These capabilities have positioned AI as a logical successor to chemometrics in many authentication scenarios.
What kinds of food fraud can AI detect?
AI systems are now capable of detecting both overt and subtle fraud across a wide range of food products. The study categorizes applications into three pillars: classification based on label attributes, adulteration detection through substitution or addition of foreign substances, and rapid recognition via image-based systems.
In honey, CNN models paired with thermographic imaging can detect rice syrup adulteration at concentrations as low as 1%, with over 92% quantification accuracy. Similarly, Raman spectroscopy paired with AI models can identify complex syrup mixtures, achieving nearly 98% classification success. In edible oils, CNN and SVM models trained on microscope and spectroscopic images have achieved 97–100% accuracy in identifying adulterants like refined or pomace oils mixed into premium extra virgin olive oil (EVOO).
Dairy products such as milk and cheese have also benefited from AI applications. Portable AI-based sensors using RGB and UV-Vis data have accurately classified milk purity and detected adulterants with over 98% accuracy. In grated cheese, SVM and RF models based on digital images could distinguish between authentic and additive-laced samples with over 80% accuracy.
In meat, a combination of image analysis and ML methods like CNNs and SVMs have enabled detection of species substitution, offal inclusion, or plant-based adulteration in minced products. These systems not only flag fraudulent items but also quantify adulterant levels with up to 98% precision.
What are the challenges and future opportunities for AI in food authentication?
Despite its advantages, the study cautions that AI's widespread implementation is not without obstacles. One primary challenge is the lack of standardization in data acquisition protocols, which can reduce the reproducibility and robustness of models across different settings. Additionally, while DL methods eliminate the need for expert feature engineering, they demand large, well-labeled datasets for training—something that remains difficult to obtain for diverse food matrices.
Furthermore, although AI-based tools outperform classical chemometric approaches in several domains, their use is recommended primarily as screening tools. Confirmatory testing still relies on validated chemical and physical methods, especially for regulatory or legal enforcement. The study emphasizes that AI systems must be used with caution due to the natural variability of food commodities, which can affect model generalization.
Nonetheless, the potential for real-time, low-cost, and portable devices based on AI is vast. Future directions include mobile applications that integrate image acquisition and AI-powered analysis for on-the-go authentication, especially in remote or low-resource settings. Additionally, research is ongoing into federated learning and edge computing models to ensure data privacy while maintaining model performance across decentralized systems.
The development of hybrid models that combine the strengths of DL and traditional analytical chemistry is also a promising area. For instance, AI can help pre-select suspicious samples for deeper analysis, increasing throughput and reducing costs in quality control laboratories.
- READ MORE ON:
- Artificial intelligence in food authentication
- AI food fraud detection
- Deep learning in food quality control
- AI in food industry
- Food adulteration detection using AI
- Machine learning food analysis
- How AI detects food adulteration
- Artificial intelligence tools for food quality
- AI vs chemometrics in food fraud detection
- FIRST PUBLISHED IN:
- Devdiscourse