Edge computing deliver real-time shield against systemic financial shocks
To bridge the digital and economic domains, the authors constructed four composite indicators: transaction efficiency, latency per transaction, utilization-to-efficiency ratio, and decision quality score. These indicators capture the operational reality of edge infrastructures and their influence on financial decisions in real time.
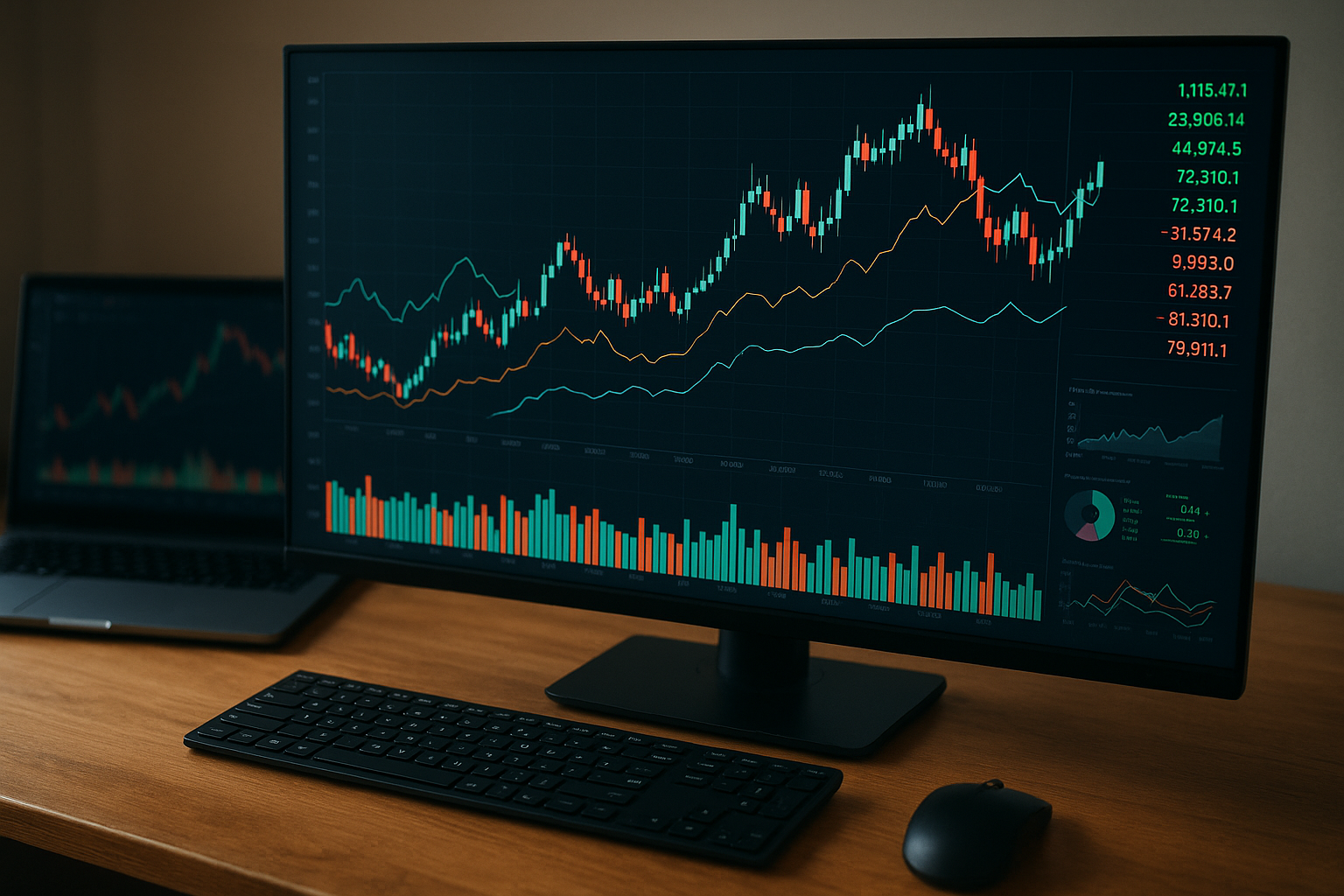
In an era of volatile global markets and increasing systemic risks, cutting-edge digital infrastructure is fast becoming a frontline defense. A new peer-reviewed study titled "Improving Real-Time Economic Decisions Through Edge Computing: Implications for Financial Contagion Risk Management," published in the journal Computers (2025), provides compelling evidence that edge computing can significantly enhance the accuracy and timeliness of economic decision-making, thereby strengthening resilience against financial contagion.
The research, conducted by Ștefan Ionescu, Camelia Delcea, and Ionuț Nica of the Bucharest University of Economic Studies, evaluates the impact of edge infrastructure performance on real-time financial decisions. Using a synthetic dataset simulating live economic environments, the study leverages machine learning models to classify decision outcomes based on computational metrics such as latency, resource utilization, and workload balance. The findings indicate that high-performing edge systems correlate strongly with accurate economic decisions and can serve as early warning indicators of systemic vulnerabilities.
How can composite indicators reflect the link between edge performance and economic decisions?
To bridge the digital and economic domains, the authors constructed four composite indicators: transaction efficiency, latency per transaction, utilization-to-efficiency ratio, and decision quality score. These indicators capture the operational reality of edge infrastructures and their influence on financial decisions in real time.
The transaction efficiency metric assesses the system’s capacity to process data-intensive economic activity with minimal delays. Latency per transaction quantifies responsiveness by calculating average delay per transaction, crucial in high-speed financial systems where even milliseconds can dictate gains or losses. The utilization-to-efficiency ratio signals whether the system’s computational resources are being overused or under-orchestrated. Lastly, the decision quality score combines system accuracy with outcome polarity, providing a nuanced measure of performance-sensitive decision effectiveness.
Descriptive analysis showed that edge latency had the highest impact on decision quality, dominating the performance composite index. Correlation studies revealed that as transaction volumes increased, latency and inefficiency also rose, suggesting bottlenecks that degrade decision-making capabilities under load. Principal Component Analysis (PCA) helped distill the multicollinearity among edge metrics, confirming that latency remains the most influential factor across decision scenarios.
What relationships exist between edge performance and economic decision outcomes?
The study uncovered significant empirical relationships linking technological performance and decision quality. Real-time indicators such as system throughput and latency showed consistent correlation with positive decision outcomes. Specifically, lower latency and balanced resource distribution were associated with more accurate and favorable economic decisions.
Through comprehensive data visualization and statistical testing, including Pearson and Kendall correlation matrices, the researchers demonstrated that efficient edge operations are not just technological conveniences but integral to robust economic decision architectures. The decision quality score was particularly well-aligned with positive outcomes, validating its utility as a diagnostic indicator. Notably, edge systems exhibiting uneven workload distribution or saturation were prone to increased decision errors, elevating the risk of cascading financial shocks during market turbulence.
Moreover, the study emphasizes that these relationships extend beyond abstract modeling. In practical terms, suboptimal decisions made under high-latency conditions can trigger chain reactions across financial institutions, especially during periods of systemic stress. By embedding performance-sensitive indicators into decision-support frameworks, financial platforms can proactively manage contagion threats, thereby reinforcing institutional stability.
Which machine learning models best predict decision outcomes in edge-enhanced settings?
Five machine learning classifiers were tested to evaluate their ability to predict binary economic decision outcomes: Logistic Regression (LR), Random Forest (RF), Support Vector Machines (SVM-RBF), Neural Networks (NN), and Extreme Gradient Boosting (XGBoost). Among these, XGBoost emerged as the most effective, achieving a 97% accuracy rate, perfect precision, and a near-perfect ROC-AUC score of 0.998.
XGBoost outperformed all competitors in handling non-linearities and feature interactions inherent in complex economic data. While Random Forest followed closely, achieving a 95% accuracy rate and similarly high recall, XGBoost’s ability to eliminate false positives while minimizing false negatives gave it a critical edge, especially in financial risk contexts where undetected failures can have catastrophic outcomes. Neural Networks showed decent performance but lacked the interpretability and precision of XGBoost. Logistic Regression and SVMs lagged in accuracy and robustness, underscoring the need for more sophisticated models in real-time, high-stakes environments.
The authors caution, however, that high-performing models like XGBoost may trade off interpretability for performance. For regulatory settings and transparent auditing, this poses a challenge. Nonetheless, they argue that with appropriate safeguards, such as explainable AI techniques and human-in-the-loop oversight, these tools can be harnessed effectively without compromising ethical standards or stakeholder trust.
Strategic Implications and Recommendations
The study’s findings carry far-reaching implications for the design of financial infrastructures and public policy. By integrating edge monitoring into financial regulation frameworks, institutions can treat digital performance metrics as predictive signals for systemic instability. Stress-testing digital architectures under simulated contagion scenarios could reveal hidden vulnerabilities long before they manifest in market crashes.
Moreover, standardized edge performance benchmarks and interoperable data protocols are necessary to build trust and consistency across platforms. For regions with underdeveloped financial systems, such as rural cooperatives or peripheral economies, edge computing may offer a leapfrogging opportunity to build real-time, adaptive decision-support systems that promote resilience and inclusion.
The authors lastly call for further research into deploying lightweight, explainable models for use on constrained edge devices, as well as exploring the applicability of their framework in real-world, multiclass economic decision environments. While the current dataset is synthetic, the results form a compelling case for integrating computational responsiveness into economic risk management at scale.
- FIRST PUBLISHED IN:
- Devdiscourse