Deep learning breakthrough enhances crop disease detection across lab and field
Plant diseases remain a major threat to food security and agricultural productivity, especially in resource-constrained settings where expert diagnosis is limited. Traditionally, disease identification relies on visual inspection by specialists, a method prone to human error and limited scalability. Leaf images, often showing early signs of infection, serve as primary indicators in diagnosis. With global agriculture facing increased vulnerability due to climate change, pests, and cross-border infections, the need for automated and accurate disease detection systems is more urgent than ever.
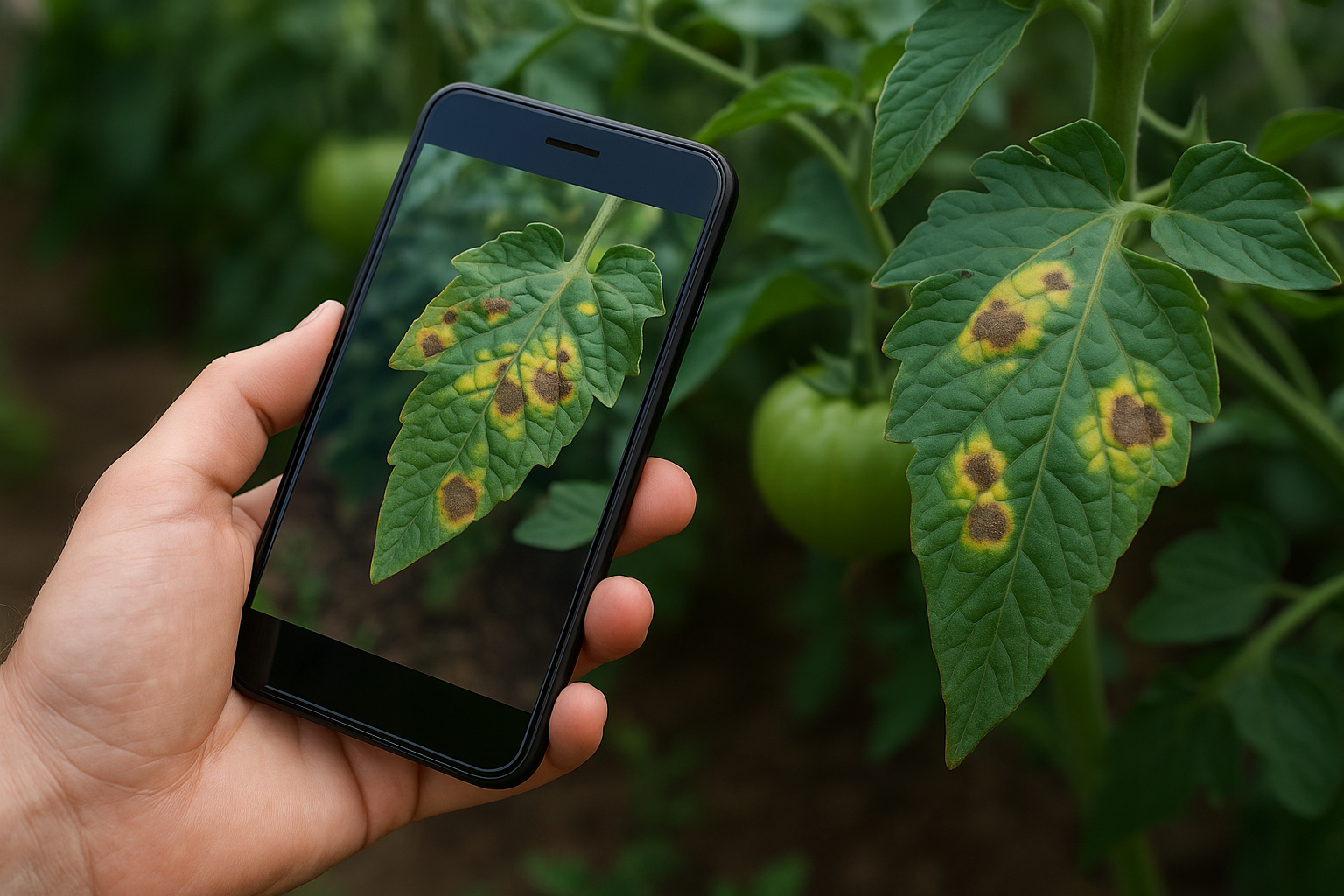
A breakthrough ensemble learning model combining multiple advanced deep learning architectures has achieved state-of-the-art accuracy in identifying plant diseases across diverse agricultural environments. The study, titled “A Robust Ensemble Model for Plant Disease Detection Using Deep Learning Architectures”, was published in the May 2025 issue of AgriEngineering. It demonstrates how integrating InceptionResNetV2, MobileNetV2, and EfficientNetB3 can significantly enhance the reliability and robustness of plant disease classification in both controlled laboratory and real-world field conditions.
Developed and tested using three datasets, PlantVillage, PlantDoc, and FieldPlant, the proposed model achieved a remarkable 99.69% accuracy on the lab-based PlantVillage dataset, 83% on the field-acquired FieldPlant dataset, and 60% on the complex, semi-structured PlantDoc dataset. The results confirm the ensemble model’s ability to generalize effectively across image types and environments, offering scalable solutions for disease surveillance in modern agriculture.
Why do we need AI-driven plant disease detection?
Plant diseases remain a major threat to food security and agricultural productivity, especially in resource-constrained settings where expert diagnosis is limited. Traditionally, disease identification relies on visual inspection by specialists, a method prone to human error and limited scalability. Leaf images, often showing early signs of infection, serve as primary indicators in diagnosis. With global agriculture facing increased vulnerability due to climate change, pests, and cross-border infections, the need for automated and accurate disease detection systems is more urgent than ever.
This research addresses that need by offering a deep learning-powered model capable of accurately identifying multiple types of plant diseases using visual data. The approach reduces reliance on human experts, minimizes the cost of manual inspections, and enables large-scale monitoring using readily available digital images.
How does the ensemble model improve detection accuracy?
The study’s core innovation lies in its ensemble methodology, which integrates three high-performing convolutional neural networks (CNNs), each selected for complementary strengths. InceptionResNetV2 contributes deep feature extraction and residual learning for complex disease patterns; MobileNetV2 adds lightweight, mobile-friendly inference capabilities; and EfficientNetB3 brings scalable accuracy with fewer parameters.
Each network was pre-trained on the ImageNet dataset and fine-tuned using plant-specific datasets. The models were partially unfrozen to allow transfer learning, enabling the ensemble to adapt to plant disease identification without sacrificing learned general features. Data augmentation—such as random rotations, flips, and zooming—was extensively applied to increase the robustness of the training process.
The final model concatenates features from each network, processes them through dense layers with batch normalization and dropout, and outputs class probabilities via a softmax layer. This combined architecture proved significantly more accurate than any individual model tested. For instance, the ensemble outperformed MobileNet (82.9%), VGG16 (80.54%), and InceptionV3 (82.54%) on the FieldPlant dataset, achieving 83% accuracy.
How did the model perform across real-world agricultural scenarios?
The model’s performance varied according to dataset complexity. PlantVillage, a clean, well-labeled dataset, allowed near-perfect accuracy. However, FieldPlant and PlantDoc presented greater challenges due to image noise, class imbalance, and variations in lighting and background. On FieldPlant, real-world leaf images with manual annotations posed difficulties for traditional models, but the ensemble achieved the highest comparative accuracy at 83%.
On PlantDoc, which features varied crops and field images from uncontrolled environments, the ensemble achieved 60% accuracy. While this lagged behind the model's lab performance, it surpassed other approaches like MobileNetV2 (40%) and EfficientNetV2 (28%) tested under the same conditions. Moreover, the ensemble model excelled in precision and recall for dominant disease classes such as Cassava Mosaic and Tomato Brown Spots, demonstrating its ability to distinguish complex symptoms from healthy tissue.
Ablation studies revealed that the inclusion of all three CNN architectures and additional regularization layers (dense, batch normalization, dropout) produced the best accuracy. Individual models or pairwise combinations underperformed, with EfficientNetB3 alone achieving only 12.2% accuracy on PlantDoc.
Confusion matrices and confidence scores provided further insights. The ensemble reliably predicted most disease classes with high certainty, though struggled with visually similar categories like Cassava Healthy vs. Cassava Brown Leaf Spot. Such results highlight areas where enhanced image preprocessing or multimodal data integration (e.g., temperature, humidity) could reduce false positives and improve diagnostic clarity.
What does this mean for the future of smart agriculture?
The model holds significant promise for smart farming systems, especially in regions where expert agricultural support is sparse. Its mobile-friendly architecture, combined with transfer learning efficiency, positions it well for integration into low-power edge devices like smartphones and agricultural drones. By empowering farmers with automated diagnostics, such tools could reduce yield losses, optimize pesticide use, and improve food supply chain stability.
Nonetheless, the study acknowledges challenges in practical deployment. Field datasets are inherently noisy and often unbalanced, which affects generalization. Also, training such ensemble models demands significant GPU resources and memory—potentially limiting accessibility for small-scale operations. Future directions include developing lighter versions of the model for real-time use, improving generalization through GAN-generated synthetic images, and exploring explainable AI methods to make predictions more transparent for end-users.
Moreover, localization of datasets is crucial. The authors propose creating region-specific disease databases, such as those focused on crops in Qatar, to enhance the model’s contextual accuracy. Incorporating environmental variables and enabling multi-label classification would further increase its utility in real-world farming.
- FIRST PUBLISHED IN:
- Devdiscourse