AI can detect Parkinson’s disease years before symptoms appear
A key barrier to effective PD treatment is the slow, inconsistent patient recruitment process for clinical trials, especially neurosurgical interventions like Deep Brain Stimulation (DBS) and Focused Ultrasound (FUS). The study illustrates how AI algorithms overcome this challenge through automated patient stratification based on symptom severity, neuroimaging profiles, and biomarker expression. This not only accelerates enrollment but also increases the likelihood of therapeutic efficacy.
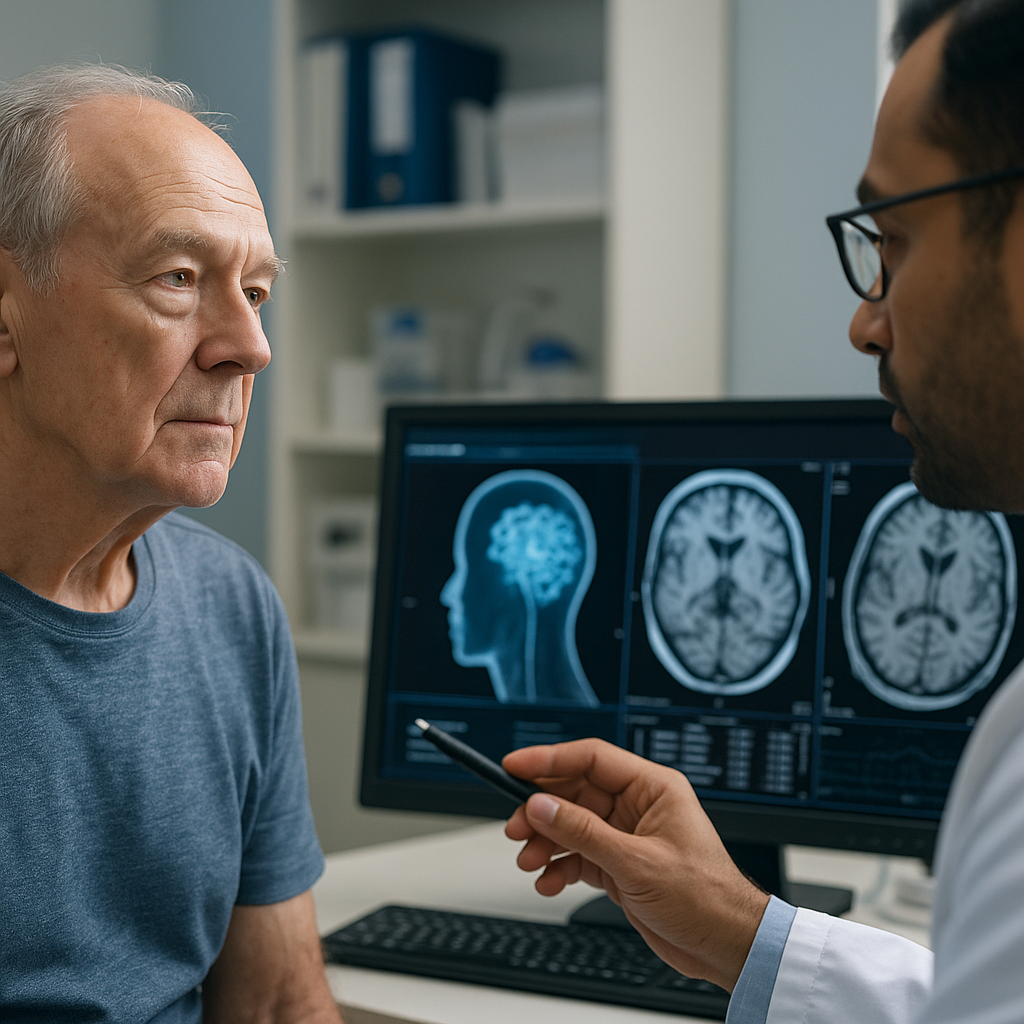
AI-driven platforms are accelerating the transformation of Parkinson’s disease neurosurgery, offering breakthroughs in patient selection, biomarker identification, and surgical precision. These technologies promise faster diagnosis, enhanced treatment personalization, and improved outcomes, according to a newly published study titled “AI-Driven Advances in Parkinson’s Disease Neurosurgery: Enhancing Patient Selection, Trial Efficiency, and Therapeutic Outcomes” in the journal Brain Sciences.
How is AI reshaping Parkinson’s diagnosis and biomarker discovery?
Parkinson’s disease (PD) remains one of the most challenging neurodegenerative conditions, largely due to its multifactorial origins and the absence of definitive early diagnostic tools. The study shows that AI is bridging this gap by enabling predictive modeling of molecular and physiological processes. Machine learning algorithms now integrate data from neuroimaging, cerebrospinal fluid (CSF) analysis, and genomic sequencing to detect early-stage Parkinson’s with unprecedented accuracy.
A notable advancement is the identification of eight blood biomarkers (e.g., GRN, MASP2, HSPA5) via proteomics and regression-based ML classifiers, offering 100% accuracy in distinguishing de novo PD patients from healthy individuals. These biomarker profiles are capable of detecting the disease up to seven years before motor symptoms emerge, which is a transformative leap in preclinical intervention.
Further, EEG-based diagnostics, analyzed through Brain Network Analytics and AI classification, have isolated 15 ERP features linked to early cognitive dysfunction in PD. This offers a scalable, non-invasive avenue for identifying neurological anomalies during the prodromal phase. The integration of AI with CSF analysis also reveals neurochemical changes, such as shifts in iron and ferritin levels, supporting longitudinal tracking of neurodegeneration.
What role does AI play in enhancing clinical trials and neurosurgical planning?
A key barrier to effective PD treatment is the slow, inconsistent patient recruitment process for clinical trials, especially neurosurgical interventions like Deep Brain Stimulation (DBS) and Focused Ultrasound (FUS). The study illustrates how AI algorithms overcome this challenge through automated patient stratification based on symptom severity, neuroimaging profiles, and biomarker expression. This not only accelerates enrollment but also increases the likelihood of therapeutic efficacy.
AI-enhanced imaging techniques such as 18F-FDG PET and high-resolution fMRI are now capable of differentiating Parkinson’s from atypical parkinsonian syndromes. Machine learning classifiers, like support vector machines and convolutional neural networks, interpret these imaging outputs to recommend optimal DBS target regions. For example, STN stimulation has shown long-term efficacy in tremor-dominant and akinetic-rigid subtypes of PD, while VIM stimulation remains preferable in older patients with unilateral symptoms.
Beyond diagnostics, AI aids in real-time modulation of DBS parameters through feedback from wearable biosensors. Deep learning platforms analyze motor and non-motor fluctuations, allowing clinicians to fine-tune neuromodulation strategies dynamically. These closed-loop systems reduce side effects and improve quality of life without repeated in-clinic visits. The FDA’s recent approval of AI-guided DBS planning tools underscores the regulatory momentum behind such innovations.
What are the challenges and future directions for AI in Parkinson’s neurosurgery?
Despite its promising utility, the study emphasizes that integrating AI into PD neurosurgery is not without hurdles. Key challenges include data standardization across cohorts, algorithmic transparency, and ensuring equitable model performance across diverse demographics. Current AI systems are highly sensitive to cohort-specific training data, which can lead to center bias or misclassification when applied in different settings.
To overcome these obstacles, the authors advocate for international collaborations among neurosurgeons, AI engineers, ethicists, and regulatory bodies. Establishing transparent frameworks and rigorous validation protocols will be critical to ensuring ethical deployment. Additionally, future research should explore AI’s capability in genotype-guided intervention strategies and the use of explainable AI (XAI) for clinician trust and patient education.
AI’s ability to process high-dimensional neurobiological data also opens up new possibilities for personalized therapy. For instance, advanced ML pipelines are being designed to classify PD subtypes using voice patterns, gait anomalies, and sleep-related biomarkers. These tools can preemptively tailor interventions based on projected disease trajectories.
- READ MORE ON:
- AI in Parkinson’s disease neurosurgery
- artificial intelligence for Parkinson’s diagnosis
- Parkinson’s disease early detection AI
- artificial intelligence in neurodegenerative disease
- how AI improves Parkinson’s disease surgical outcomes
- Parkinson’s diagnosis using blood and EEG biomarkers
- AI-enhanced clinical decision-making in Parkinson’s treatment
- FIRST PUBLISHED IN:
- Devdiscourse