AI predicts chronic disease patterns using electronic health records
AI tools are transforming EHR datasets into actionable clinical insights by identifying disease patterns, predicting comorbidities, and stratifying patient risk. The reviewed studies predominantly used clustering techniques, XGBoost, random forest, and neural networks to uncover multimorbidity networks and forecast disease trajectories.
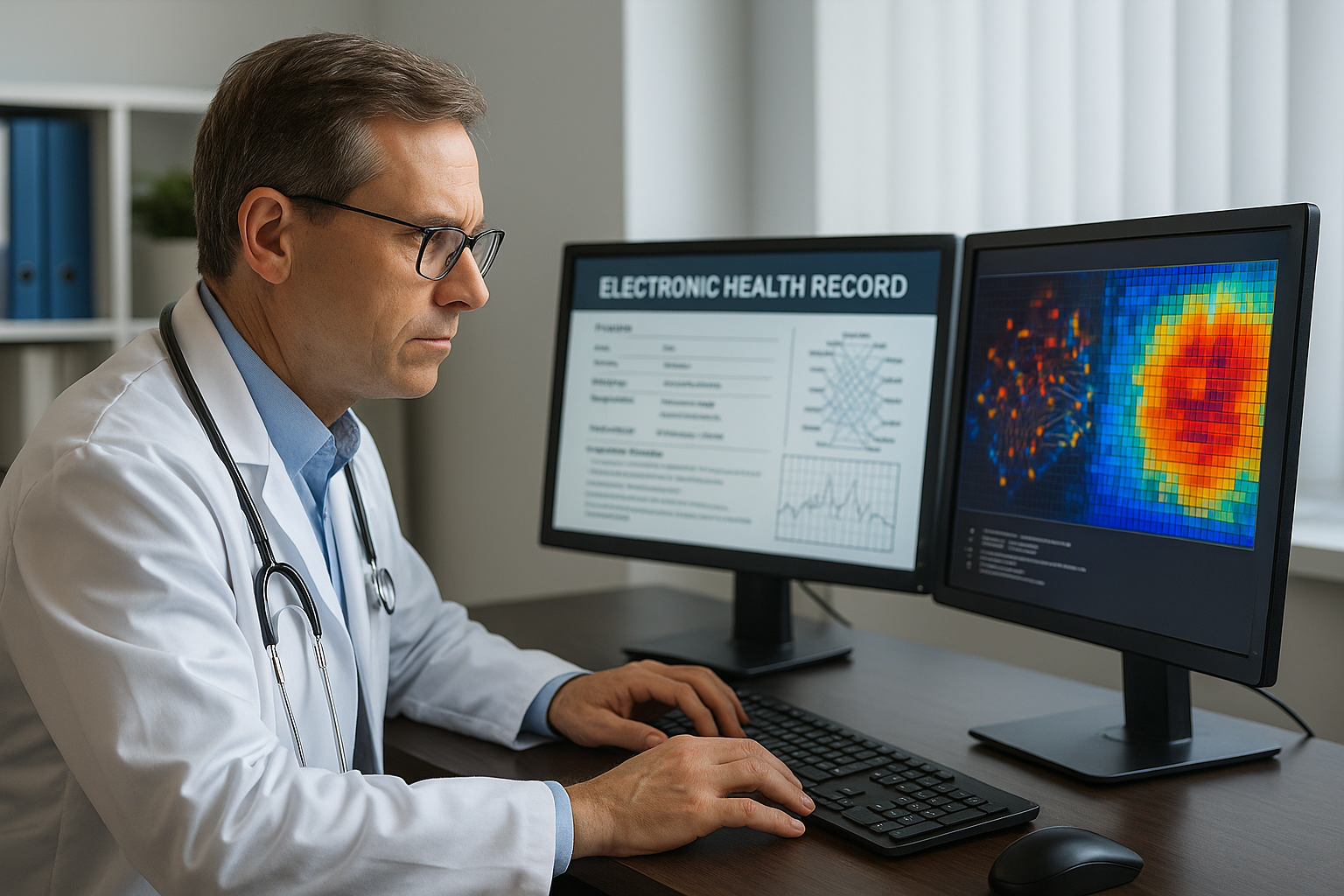
As healthcare systems worldwide confront the escalating challenges of managing patients with multiple chronic conditions, artificial intelligence (AI) has emerged as a pivotal tool for improving clinical insight, operational efficiency, and personalized care. A landmark study has now synthesized the field’s progress by systematically reviewing AI applications that leverage electronic health records (EHRs) to address multimorbidity.
Published in the Journal of Clinical Medicine, the study titled "Electronic Health Records: A Gateway to AI-Driven Multimorbidity Solutions—A Comprehensive Systematic Review" examines 44 peer-reviewed studies using real-world data to explore disease prediction, treatment optimization, and healthcare service utilization for patients with two or more chronic conditions.
How is AI being used to predict and classify multimorbidity?
AI tools are transforming EHR datasets into actionable clinical insights by identifying disease patterns, predicting comorbidities, and stratifying patient risk. The reviewed studies predominantly used clustering techniques, XGBoost, random forest, and neural networks to uncover multimorbidity networks and forecast disease trajectories.
Clustering models grouped patients by clinical similarities, uncovering patterns of co-occurring conditions such as cardiovascular and renal disease. XGBoost and random forest models delivered accurate predictions of future health events, including hospital readmissions, drug side effects, and mortality. These models were especially effective in handling unstructured or imbalanced datasets common in clinical records.
Neural networks, particularly deep learning architectures, demonstrated superior ability in parsing complex datasets and supporting longitudinal analysis of patient outcomes. For instance, denoising autoencoders and convolutional networks were employed to predict disease progression and derive latent patient features from noisy data.
Despite promising accuracy metrics, some models reporting over 90% in precision and recall, the study highlights a major gap in consistent evaluation. Only half of the included studies provided comprehensive performance metrics such as F1-scores or AUC, limiting reproducibility and comparative analysis across models.
What role does AI play in drug safety and prescription optimization?
Polypharmacy is a critical concern for patients with multimorbidity, often leading to adverse drug reactions and complex therapeutic regimens. The review identifies drug recommendation systems as a growing AI application area, offering clinicians evidence-driven support for safer prescribing.
Using EHR-integrated AI models, researchers were able to identify high-risk drug combinations, flag inappropriate medications, and predict emergency admissions caused by adverse interactions. For example, random forest algorithms showed capacity to forecast adverse drug reactions based on patient profiles, while reinforcement learning systems dynamically adapted treatment recommendations to minimize drug-related harm.
These systems also contribute to improved prescription appropriateness by customizing drug regimens to individual patient needs. They go beyond rule-based alerts, leveraging machine learning to assess historical health trajectories, current diagnoses, and medication histories.
However, model implementation faces practical constraints. The studies included in the review often relied on retrospective data and were limited by inconsistent documentation across EHR systems. Moreover, few AI models underwent prospective validation or clinical trials, underscoring the need for translational research before widespread deployment in clinical settings.
How can AI improve healthcare delivery and policy for multimorbidity?
Beyond clinical predictions, AI’s utility extends into system-level improvements in healthcare delivery. The review outlines how AI tools extract psychosocial factors from EHRs to predict healthcare utilization, such as emergency visits or psychiatric readmissions. This is particularly crucial in high-risk populations like patients with schizophrenia or severe mental illness.
AI models also support the development of dynamic risk scores, overcoming the rigidity of traditional clinical scoring systems. By training on large, diverse datasets, machine learning models produce adaptable risk profiles for patients with multiple conditions - far more representative than one-size-fits-all diagnostic tools.
From a policy standpoint, AI-powered multimorbidity research offers a foundation for data-driven public health planning. Identifying high-risk clusters and disease trajectories enables better resource allocation, preventive interventions, and care pathway design. The study notes that predictive analytics will likely become one of the most transformative technologies in public health over the next two decades.
Nevertheless, several barriers remain. The review points to wide methodological variability across studies, a lack of standard definitions for multimorbidity, and insufficient transparency in model performance reporting. These limitations currently prevent meaningful meta-analyses and pose challenges for clinical adoption.
Future research must prioritize standardization, validation, and transparent reporting. The integration of AI into healthcare systems must also be accompanied by strong governance, interdisciplinary collaboration, and rigorous evaluation to ensure equitable and effective deployment.
- READ MORE ON:
- electronic health records
- AI for chronic disease management
- EHR data analysis
- AI in multimorbidity care
- how AI uses EHRs to predict chronic conditions
- artificial intelligence in managing multiple diseases
- machine learning models for multimorbidity
- real-world data in AI health applications
- FIRST PUBLISHED IN:
- Devdiscourse