Custom Diet Tips from AI: Fuzzy Variables Enhance Clarity and Nutritional Precision
Researchers at the University of Granada developed a generative AI system enhanced with fuzzy logic to deliver personalised, nutritionally accurate food recommendations. The approach significantly improves clarity, trustworthiness, and adherence to dietary guidelines compared to conventional methods.
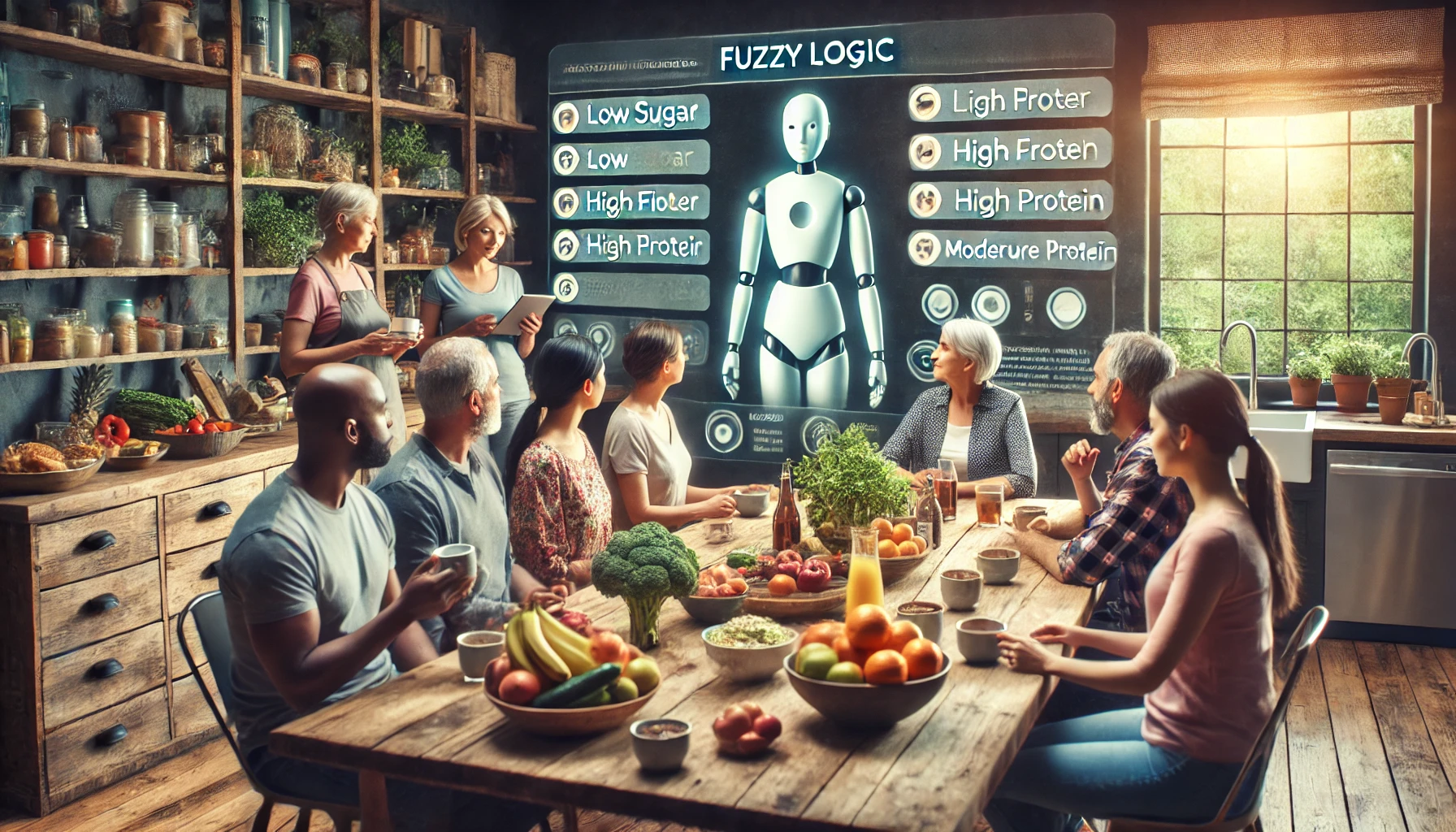
In a groundbreaking study conducted by the Department of Computer Science and Artificial Intelligence at CITIC-UGR (Research Center for Information and Communication Technologies) and the Faculty of Health Sciences at the University of Granada, researchers have introduced a novel fusion of fuzzy logic and generative AI to deliver personalised, health-aware food recommendations. Their work addresses the urgent global issue of diet-related diseases, which account for one in every five deaths worldwide. By developing a recommendation framework rooted in WHO dietary standards and user-specific profiles, the team seeks to empower individuals with understandable, accurate, and scientifically rigorous nutritional advice.
Tackling Poor Nutrition with Intelligent Technology
The traditional landscape of food recommendation systems has largely been limited to catering for user preferences, often at the expense of nutritional value and personalised health considerations. At a time when large language models (LLMs) like ChatGPT are increasingly used to answer health-related questions, the lack of contextual and scientific depth in these outputs poses real risks. The study’s authors argue that current AI-generated dietary suggestions often overlook critical variables such as individual energy needs, gender-sensitive nutrition requirements, and evidence-based dietary thresholds. To bridge this gap, the proposed system integrates fuzzy linguistic modelling, enabling AI to assign human-readable labels like “low,” “medium,” or “high” to the content of key nutrients in food items.
Fuzzy Logic Meets AI Language Models
At the heart of the research is a computational pipeline that calculates personalised dietary guidelines for users based on their weight, height, and age. This data is used to compute the Basal Metabolic Rate (BMR), a foundation for estimating individual nutrient limits. Four critical nutrients, fat, saturated fat, sugar, and salt, are then analysed using fuzzy sets that transform raw numeric values into linguistic categories. These fuzzy variables are embedded into prompts that instruct the AI on how to frame nutritional recommendations in simple, non-technical language. For example, a food item high in salt but low in fat and sugar might be categorised as suitable for occasional consumption. The model can then explain this to users using natural language tailored to their understanding.
Three types of prompt strategies were tested: a fuzzy prompt (providing pre-classified fuzzy variables), a crisp prompt (using raw nutritional values), and a crisp2fuzzy prompt (asking the model to infer fuzzy values from numerical inputs). Additionally, four AI-generated personas were used to vary the tone and approach of the explanations: a professional nutritionist, a chef with nutritional knowledge, a university professor, and a layperson with no expertise. These combinations were tested using LLaMA3, a state-of-the-art generative AI model, to evaluate the best strategies for delivering clear, accurate, and engaging dietary advice.
Extensive Testing Across Cultures and Food Types
To ensure cultural and dietary diversity, the researchers applied their framework to two well-established nutritional datasets: the UK’s CoFID (Composition of Foods Integrated Dataset) and Spain’s BEDCA (Base de Datos Española de Composición de Alimentos). Together, these databases cover over 3800 food items, including fresh produce, packaged goods, and traditional regional dishes. The system was tasked with generating frequency-of-consumption recommendations for each food item and explaining the rationale behind those suggestions.
The results were impressive. Fuzzy prompt strategies consistently outperformed the other two in terms of clarity, semantic richness, and nutritional rigour. Recommendations written in the voice of a professor of nutrition emerged as the most coherent and trustworthy, with the nutritionist close behind. Readability metrics like the SMOG index and Dale-Chall score showed that these prompts were more accessible to general audiences. Sentiment analysis revealed that the best-performing outputs maintained a neutral tone and low subjectivity, essential qualities for public health communications. Moreover, the fuzzy-enhanced responses were statistically more aligned with WHO dietary recommendations, minimising hallucinations and ambiguous statements.
Real Impact Confirmed by Human Evaluations
To assess real-world applicability, the team conducted a human-centred evaluation involving 15 diverse annotators, ranging in age, gender, and nutritional literacy. Participants reviewed AI-generated dietary advice and rated it based on clarity, usefulness, and trustworthiness. The fuzzy strategy earned the highest scores across the board, while explanations generated by non-expert personas ranked the lowest. More importantly, users were asked to rate how often a food item should be consumed both before and after reading the AI’s recommendation. The post-explanation ratings were significantly more aligned with expert guidance, indicating that the model had a measurable impact on users’ dietary awareness and decision-making.
Future Horizons for Smarter, Safer Nutrition
Though the current system focuses on four core nutrients, the researchers see vast potential for expansion. Future iterations could incorporate fibre, vitamins, and micronutrients to provide a more holistic dietary overview. The flexible nature of fuzzy logic also allows the system to adapt to different geographic nutritional standards and cultural preferences, making it suitable for global deployment. Beyond nutrition, the methodology could be extended to other expert-driven domains like healthcare advice, financial planning, and educational tutoring, anywhere explainable AI is needed to support informed decisions. Despite some limitations, such as the reliance on complete food databases and static user profiles, this study represents a powerful step forward in making AI-generated dietary recommendations both trustworthy and deeply personalised.
- FIRST PUBLISHED IN:
- Devdiscourse
ALSO READ
Hand Hygiene Key to Mpox Prevention: WHO Outlines Urgent Global Strategy
Health for All: WHO Outlines 40 Actions to Ensure Disability-Inclusive Care
Waqf Act: CJI Khanna, who retires on May 13, says he does not want to reserve order on pleas challenging validity of law.
Reinforcing Hand Hygiene: WHO Urges Global Action Beyond Glove Use
Zero Hepatitis B in Children: Maldives and Sri Lanka Reach WHO Control Milestone