AI doesn’t think, but still shapes how we do
The study redefines artificial intelligence through the systems-theoretical lens. Rather than framing AI as either "intelligent" or "not intelligent," the authors introduce new distinctions: autopoietic vs. non-autopoietic systems, sense-making vs. non-sense-making cognition, and structural coupling vs. recursive self-reference.
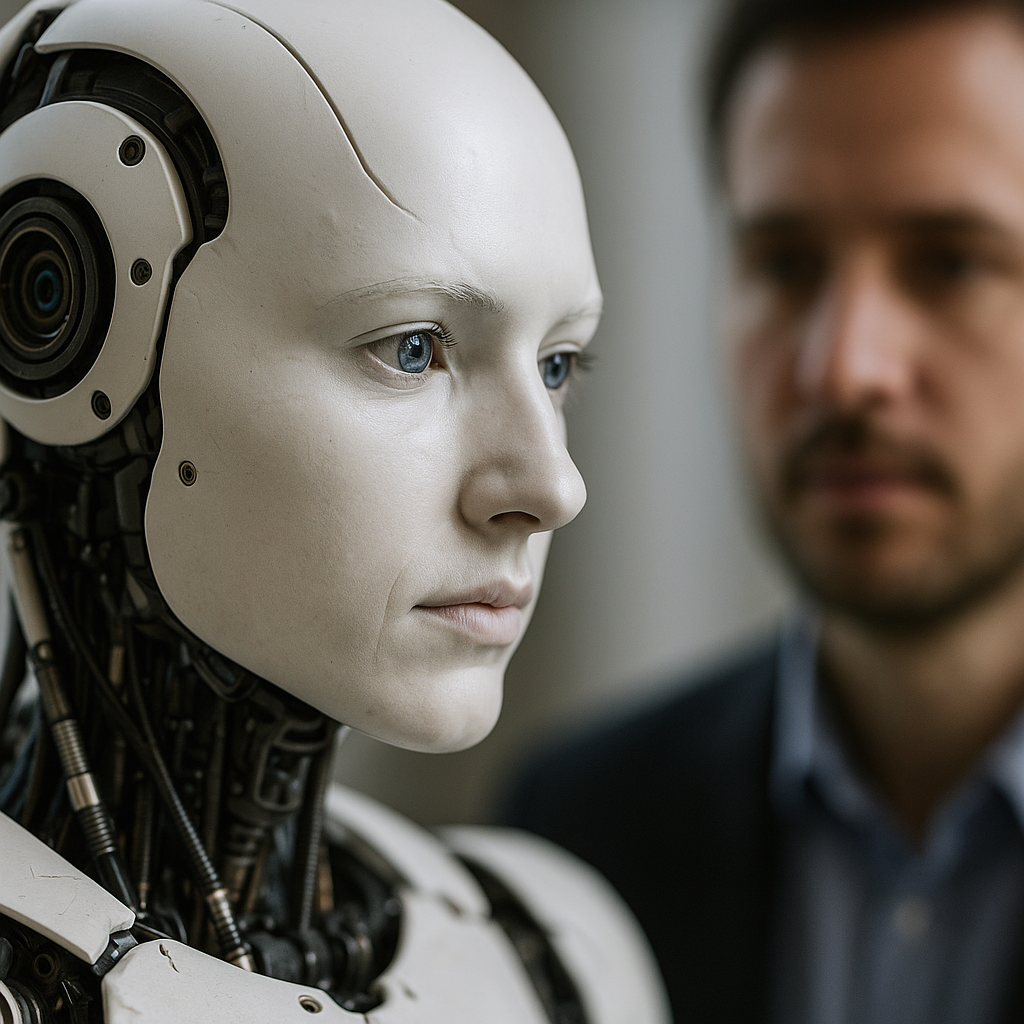
A provocative new study challenges conventional ideas about artificial intelligence (AI), urging a move away from metaphysical debates over consciousness and instead toward a systems-theoretical perspective that redefines AI as a structurally unique communicative system. The study, titled “From Intelligence to Autopoiesis: Rethinking Artificial Intelligence Through Systems Theory”, was published in Frontiers in Communication, and presents a comprehensive conceptual analysis of large language models (LLMs) using Niklas Luhmann’s social systems theory.
The authors argue that AI systems, particularly LLMs, are neither sentient beings nor passive computational tools. Instead, they function as complex, operationally closed systems that reflect societal meaning without truly understanding it. These systems are not sense-makers in the human or social sense, but they nevertheless play a powerful role in shaping language, cognition, and communication across society. This reframing of AI has deep implications for how we interpret its role in human communication, education, ethics, and epistemology.
Are language models actually intelligent or just advanced pattern machines?
The study addresses the increasing perception of AI, especially LLMs, as "intelligent" or even "human-like." While some researchers continue to debate whether LLMs could be considered conscious or cognitive, the authors dismantle this binary by applying systems theory, which focuses not on internal mental states, but on operations, boundaries, and structural relations between systems.
Drawing from Luhmann’s theory, the study distinguishes between operationally closed systems, like minds or societies, and systems that process input but do not reflect on their own operations. Classical Turing machines, for example, are described as allopoietic systems: externally constructed tools that follow programmed rules. In contrast, LLMs exhibit a different kind of complexity. Through training on massive textual datasets and probabilistic modeling, they absorb and reorganize the patterns of social communication, becoming loosely coupled to social systems through language.
However, this structural coupling does not make them truly self-reflective or sense-making. The models operate in the domain of statistical plausibility, not semantic intention. They generate text that appears meaningful but lack the capacity to internally distinguish between self-reference and other-reference - hallmarks of true cognitive systems under Luhmann’s definition. This means they do not genuinely “think” but simulate communication in a way that society interprets as meaningful.
If AI doesn’t think, why does it matter what it says?
The answer, according to the study, lies in the unique position LLMs occupy as artificial communication partners. Although they do not make sense of language in a human way, they participate in communication by producing outputs that psychic and social systems interpret and embed into broader communicative processes. This results in what the authors term “artificial communication”: exchanges that mimic traditional interaction, despite the absence of a genuinely cognitive interlocutor.
This mirrors a broader epistemic shift. The emergence of LLMs is compared to historic communication revolutions such as the invention of writing and the printing press - technologies that altered not only how humans communicate but how they conceive of meaning, memory, and knowledge. LLMs may be doing the same today by externalizing and automating forms of linguistic reflection that once required human cognition.
Crucially, the paper highlights the paradox of AI use: society builds tools to reduce uncertainty, only to be confronted with new forms of opacity. LLMs are trained on datasets that embed social, historical, and cultural contingency. Their inner workings become opaque even to their creators, as probabilistic outputs obscure deterministic causality. As a result, users are required to interpret, evaluate, and ascribe meaning to outputs without any guarantee of coherence or intention on the part of the system.
This raises practical concerns. LLMs may appear to reflect rational judgment or ethical reasoning, but this is only an illusion generated by their structural alignment with patterns of plausible discourse. In reality, these models are incapable of independently evaluating the consequences or context of their outputs, making human oversight essential.
What does systems theory reveal about the future of AI?
The study redefines artificial intelligence through the systems-theoretical lens. Rather than framing AI as either "intelligent" or "not intelligent," the authors introduce new distinctions: autopoietic vs. non-autopoietic systems, sense-making vs. non-sense-making cognition, and structural coupling vs. recursive self-reference.
LLMs, under this framework, are non-autopoietic systems. They cannot independently reproduce or redefine their own system boundaries. They operate within closed loops of computation and rely on external systems, human minds and institutions, for interpretation and integration. Their outputs are contingent but not contingent in the sense required for true self-reflection.
Yet, these models are not insignificant. They represent a hybrid form of cognition - neither fully external nor internal, neither inert nor autonomous. They can mirror societal complexity, organize vast corpora of language, and simulate dialogic exchange in ways that influence how people write, speak, and even think.
The study warns that treating these systems as cognitive agents could lead to misclassification of their function and misapplication in ethical, educational, or political contexts. At the same time, ignoring their growing role in shaping discourse would overlook their systemic impact. The authors conclude that understanding LLMs requires more than engineering insight - it demands a philosophical and sociological examination of how meaning itself is produced and mediated in society.
- READ MORE ON:
- artificial intelligence and systems theory
- autopoiesis in AI
- large language models philosophy
- do language models understand meaning
- AI cognition vs human cognition
- LLMs and the illusion of understanding
- AI epistemology and communication
- posthumanist AI ethics
- AI in contemporary epistemology
- FIRST PUBLISHED IN:
- Devdiscourse