New AI model boosts rail safety with real-time cyber and energy resilience
The researchers structured the model to meet compliance under the EU Artificial Intelligence Act (EU Directive 2024/1689), which mandates transparency, auditability, and proportionality for high-risk AI systems. The signaling system, being part of critical transport infrastructure, falls under this category. The model maintains transparency by logging performance data, using clear thresholds for alert generation, and enabling easy audits through MAE and R² metrics.
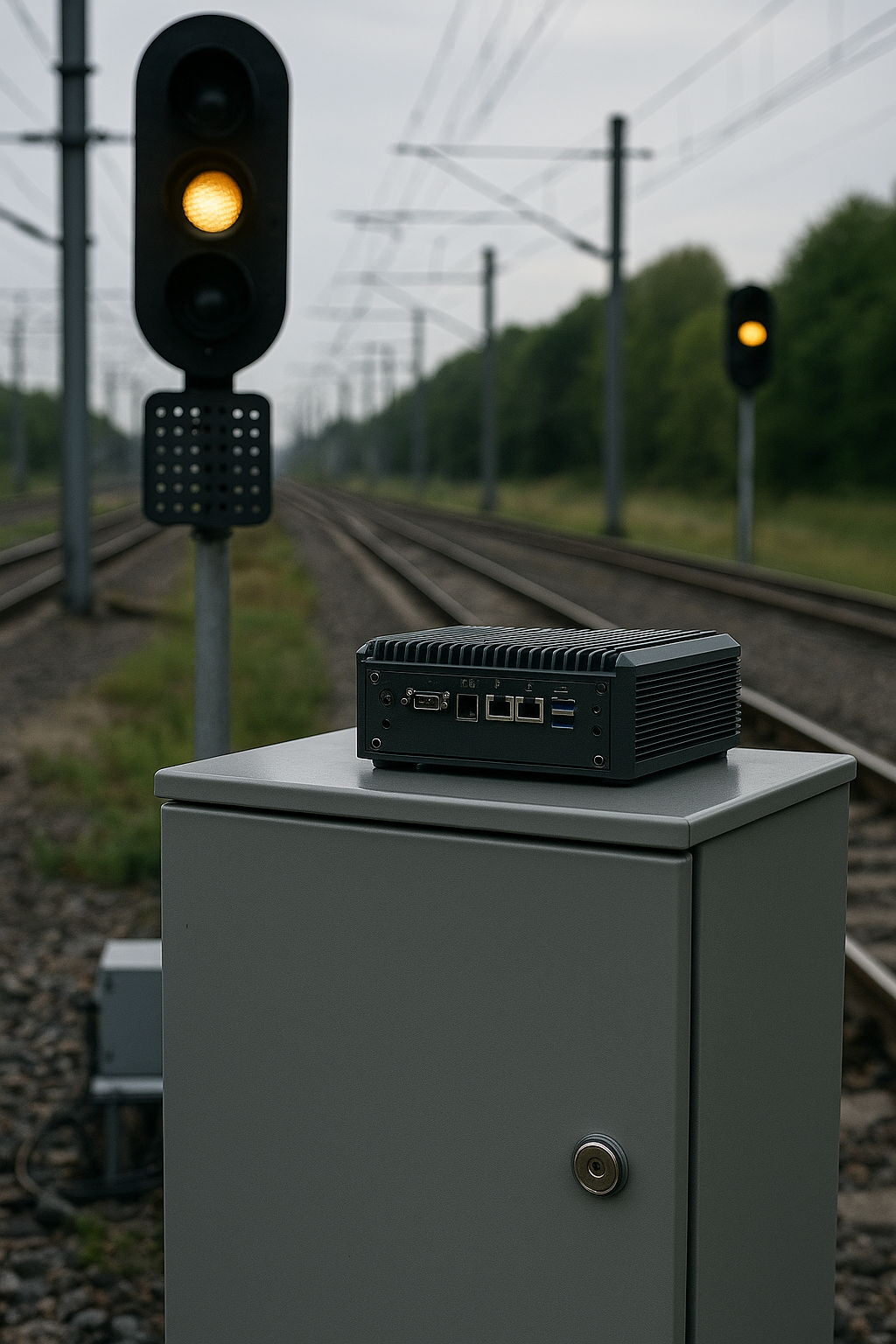
Amidst the rising cyber threats and power instability in rail networks, a new study has proposed a dual-function artificial intelligence model designed to fortify both cybersecurity and energy resilience in railway signaling systems. The study, titled “Future Rail Signaling: Cyber and Energy Resilience Through AI Interoperability”, was published in the May 2025 issue of Sustainability, and presents a simulation-validated model capable of early cyber-attack detection and robust energy sustainability forecasting in real time.
Focusing on the critical infrastructure of the Vilnius–Klaipėda double-track railway corridor in Lithuania - a key freight link to the Baltic Sea for Ukrainian exports - the study models a proactive signaling AI framework. The model integrates a feedforward neural network capable of tracking vital operational parameters and issuing early warnings of ransomware-based disruptions. The system not only predicts imminent cyber intrusions up to 20 minutes before they occur but also maintains the accuracy of energy consumption monitoring even during coordinated attack phases.
How does AI enhance cyber and energy resilience in rail signaling?
The model designed by the authors utilizes a multilayer perceptron (MLP) neural network trained on operational and simulated data from a real-world railway environment. This includes information such as voltage levels, train speeds, gradient data, and anomaly signals. The AI model is calibrated using MATLAB simulations to detect ransomware-induced anomalies and energy instability with minimal mean absolute error (MAE = 0.0331) and a coefficient of determination (R² ≈ 0.94), signaling high predictive accuracy.
In the simulated scenario, a ransomware attack begins at minute 60 of a 120-minute session. However, the AI model identifies precursors, such as increased port scanning and delay in heartbeat packets, 20 minutes in advance. This early detection window gives system operators critical time to take corrective measures before a complete system shutdown. The same model also tracks the energy consumption profile of the signaling network, ensuring operational safety and power conservation under both normal and compromised conditions.
The network architecture consists of two hidden layers (64 and 32 neurons respectively) and two output neurons, one responsible for energy resilience metrics and the other for cyber-attack probability. This streamlined structure ensures quick processing with minimal computing resources, a vital requirement for deployment in field-based railway environments with limited hardware overhead.
How is the AI system designed to meet legal and operational standards?
The researchers structured the model to meet compliance under the EU Artificial Intelligence Act (EU Directive 2024/1689), which mandates transparency, auditability, and proportionality for high-risk AI systems. The signaling system, being part of critical transport infrastructure, falls under this category. The model maintains transparency by logging performance data, using clear thresholds for alert generation, and enabling easy audits through MAE and R² metrics.
Moreover, the study cross-references technical interoperability standards from Directive 2016/797, which requires that AI components in railway signaling systems meet safety, reachability, and serviceability criteria. The modular AI model meets these by ensuring predictive capability across various operating conditions, easy retraining for new device configurations, and compliance with Common Safety Methods (CSMs) outlined in EU Directive 2016/798.
The simulation-based validation includes real-world considerations such as mixed traffic speeds (ranging from 70–150 km/h), slope variability, and attack conditions to ensure functional applicability. The system is further designed to be easily upgradable and scalable across different railway lines with minor model retraining, enhancing its feasibility for wide deployment.
What are the implementation challenges and future prospects?
Despite its effectiveness, the study acknowledges key limitations in the model's current form. The system presently functions as a binary classifier, capable of indicating whether a cyber threat exists but not distinguishing between different types of attacks. In high-complexity scenarios with multiple simultaneous threats, this could limit situational granularity.
To address this, the authors propose expanding the output layer for multiclass threat detection and incorporating recurrent neural networks or ensemble learning methods. These upgrades would enable the model to analyze sequential attack patterns and handle multi-vector failures more effectively. Additionally, integrating the system into a digital twin framework could allow rail operators to simulate large-scale scenarios without risking live infrastructure.
From a practical standpoint, the authors recommend phased deployment of the AI system. Starting with a “shadow mode” implementation allows operators to monitor the system’s outputs without integrating them into control decisions, enabling fine-tuning and reducing the risk of false positives. Other critical steps include enhancing sensor infrastructure, investing in network segmentation, and ensuring rigorous training for dispatch personnel to interpret AI outputs in real-time.
The model is particularly valuable in regions with heightened geopolitical risk or outdated signaling infrastructure. Recent cyber-attacks on railway systems in Poland and France underscore the vulnerability of critical rail infrastructure to digital sabotage. By deploying this AI-driven architecture, rail operators could preempt such disruptions and ensure continuity in freight and passenger operations under extreme conditions.
- FIRST PUBLISHED IN:
- Devdiscourse