Explainability and validation gaps threaten AI’s biomedical potential
One of the most notable trends highlighted in the study is the shift toward lightweight AI models optimized for execution in real time, on low-power platforms, and outside conventional healthcare environments. These include embedded systems like Jetson modules and Raspberry Pi devices that allow AI-driven tools to function in rural clinics, emergency response teams, and even patients' homes.
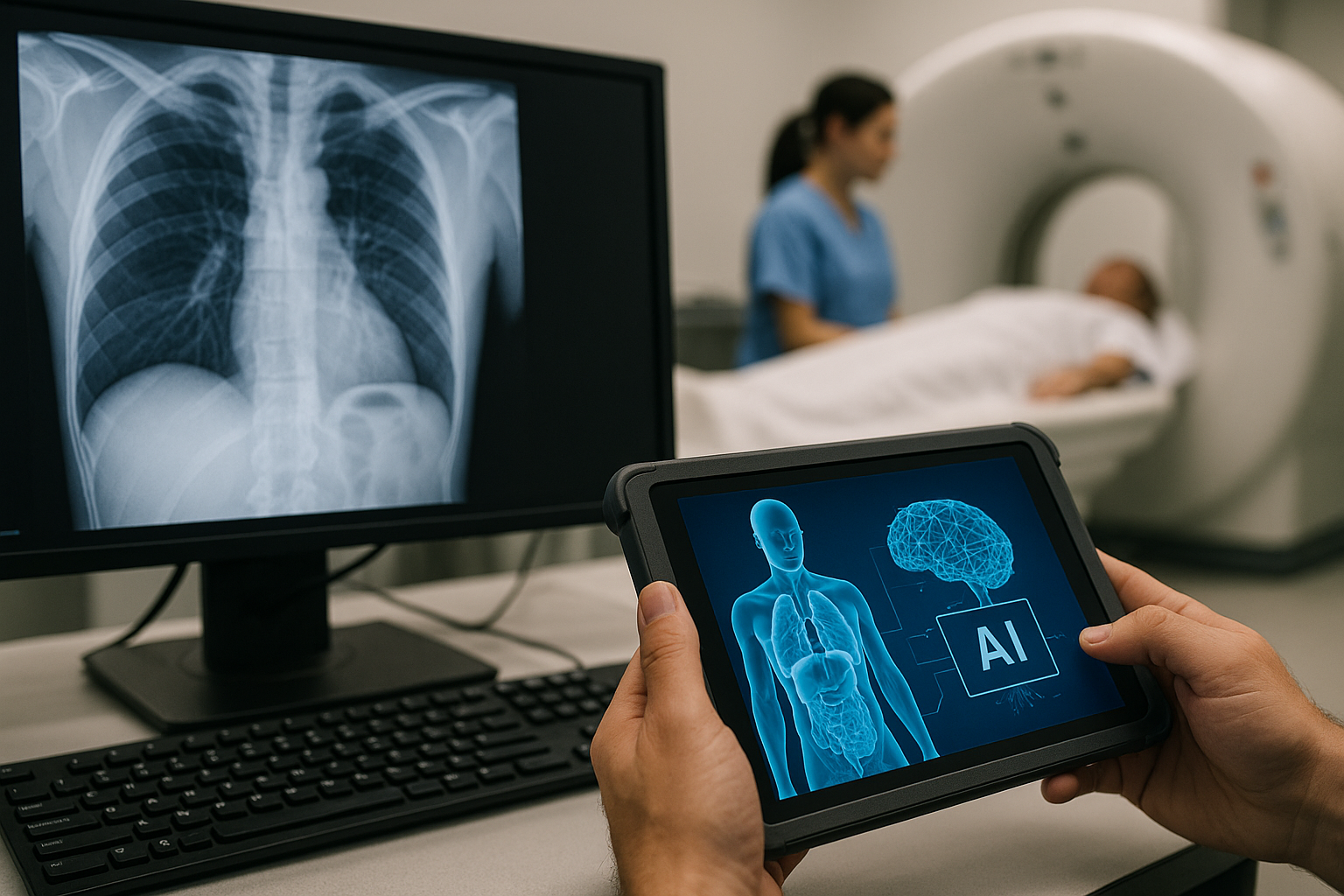
The rapid adoption of artificial intelligence (AI) in biomedical technology is reaching a tipping point. A new study titled “Artificial Intelligence in Biomedical Technology: Advances and Challenges” published in Technologies (Vol. 13, Article 208) explores the cutting-edge progress made in clinical imaging, physiological monitoring, real-time diagnostics, and accessibility solutions. Yet, the authors also issue a clear warning: without improvements in model explainability, clinical validation, data diversity, and system integration, these technologies may fall short of transforming real-world healthcare.
Conducted by a research team at the Universidad Autónoma de Querétaro and Tecnológico Nacional de México, the study reviews recent literature and technological implementations, mapping the evolution of biomedical AI from hospital-based diagnostics to decentralized applications, such as wearable devices and community-focused tools.
How is biomedical AI expanding beyond traditional hospital settings?
One of the most notable trends highlighted in the study is the shift toward lightweight AI models optimized for execution in real time, on low-power platforms, and outside conventional healthcare environments. These include embedded systems like Jetson modules and Raspberry Pi devices that allow AI-driven tools to function in rural clinics, emergency response teams, and even patients' homes.
This transition is driven by the need for decentralized and accessible care. Real-time AI processing now supports mobile diagnostics, remote ultrasound analysis, and wearable systems for fall detection or ergonomic posture monitoring. Local computation reduces reliance on internet connectivity, making these tools valuable in low-resource settings, humanitarian missions, and public health campaigns.
In addition to performance optimization, there is an increasing use of AI in non-diagnostic contexts such as automatic sign language recognition, workplace safety monitoring, and well-being interventions. These applications reflect an expansion of AI's functional reach beyond strictly clinical domains, signaling a socially responsive evolution of the technology.
What technical innovations are enhancing AI accuracy and utility?
The study outlines several key innovations accelerating the usability and adaptability of biomedical AI systems:
-
Synthetic Data Generation: One major advancement is the use of synthetic datasets, created via generative adversarial networks (GANs) and diffusion models, to compensate for limited or imbalanced clinical datasets. These synthetic images and signal patterns can be used to train AI algorithms for rare disease detection or minority population representation, boosting model robustness and reducing bias risks.
-
Explainable AI (XAI): Explainability is now recognized as essential rather than optional. Techniques such as activation maps, variable importance analysis, and filter visualization are being incorporated into clinical decision-support systems. These tools help medical professionals understand AI predictions, trace model logic, and detect potential misclassifications or biases prior to patient-facing deployment.
-
Compact Model Architectures: Developers are prioritizing architectures that support on-device inference, minimizing power consumption and maximizing computational speed. These models are increasingly compatible with edge computing, IoT platforms, and mobile healthcare apps, making them viable even in digitally underserved regions.
Taken together, these technologies enable real-time, interpretable, and scalable AI solutions that are being tested in both urban hospitals and peripheral healthcare environments. Their adoption represents a shift toward AI systems that are both technically sophisticated and socially relevant.
What barriers still limit AI’s clinical integration and impact?
Despite encouraging advances, the study identifies persistent obstacles that threaten the effective translation of AI from lab prototypes to hospital workflows.
First among them is limited real-world validation. Most models are tested in idealized lab conditions or with highly curated datasets that do not reflect the variability of actual clinical populations. As a result, AI tools often fail to generalize, particularly when deployed across diverse ethnicities, age groups, or regional healthcare systems.
Second, interoperability remains a critical concern. Many AI tools are not designed to integrate with electronic health records (EHRs), hospital databases, or national health information platforms. They often lack support for clinical data standards such as HL7 FHIR, making large-scale implementation technically and administratively challenging.
Third, explainability and transparency lag behind deployment ambitions. Many systems still function as “black boxes,” providing accurate predictions without clear justifications. This undermines trust among clinicians, impedes regulatory approval, and increases the risk of bias or unanticipated failure in sensitive applications like cancer screening or cardiac diagnostics.
Additionally, bias in training data is a structural issue. Many AI models are built using data from homogeneous populations in high-income countries, leaving them poorly suited to resource-limited settings or underrepresented demographic groups. Although synthetic data can help address these gaps, its reliability is not yet fully validated in clinical trials.
- FIRST PUBLISHED IN:
- Devdiscourse