LLMs use richer language cues to flag dementia early
The research reveals that human perception of dementia is often inconsistent and heavily reliant on a narrow set of observable cues. Annotators tended to associate short sentences and specific character mentions with cognitive health, sometimes contradicting clinical markers that flagged the same features as signs of dementia. In contrast, LLMs used a much richer set of features spanning linguistic patterns, emotional cues, and inferred social context.
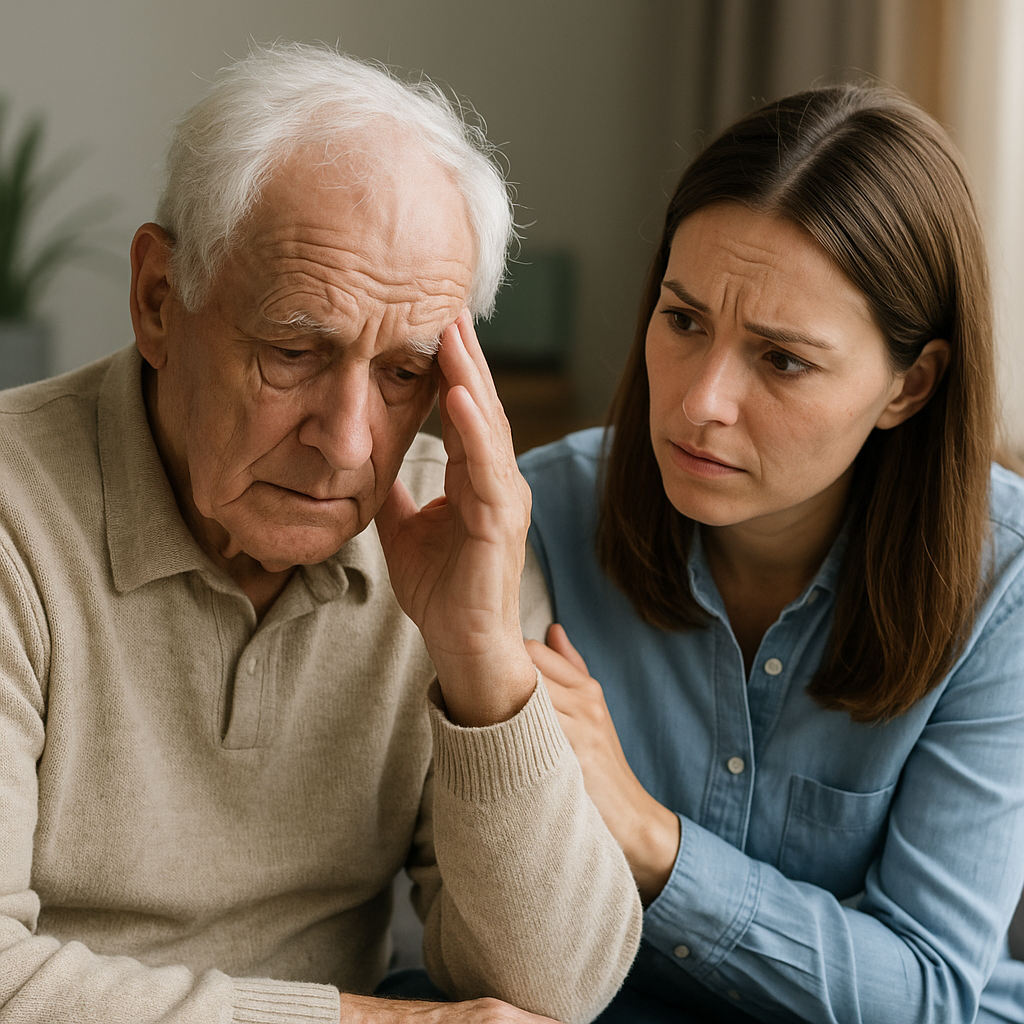
A new study published on arXiv titled “Dementia Through Different Eyes: Explainable Modeling of Human and LLM Perceptions for Early Awareness” investigates how large language models (LLMs) and non-expert humans interpret language signals to detect signs of dementia. By comparing their judgments to clinical diagnoses, the research reveals profound differences in perception accuracy, feature reliance, and explainability, insights that could reshape how society approaches early detection of cognitive decline.
The study proposes a transparent, explainable AI pipeline to evaluate the alignment, or misalignment, between non-expert perception and expert clinical insight, using real diagnostic speech data from the widely studied Pitt corpus. It examines how different evaluators - 27 human annotators and three prominent LLMs (LLaMA 3, GPT-4o, and Gemini 1.5 Pro) - label transcribed speech as either “healthy” or “dementia-affected,” based solely on textual cues.
How do humans and LLMs perceive dementia differently?
The research reveals that human perception of dementia is often inconsistent and heavily reliant on a narrow set of observable cues. Annotators tended to associate short sentences and specific character mentions with cognitive health, sometimes contradicting clinical markers that flagged the same features as signs of dementia. In contrast, LLMs used a much richer set of features spanning linguistic patterns, emotional cues, and inferred social context.
Key features such as disfluencies and non-specific language, strong predictors of dementia in clinical literature, were significantly weighted by LLMs. For example, in the logistic regression model, the presence of disfluencies increased the likelihood of a “dementia” label nearly ninefold. Human annotators often overlooked such signals or misinterpreted them.
Despite LLMs’ broader perceptual base, both groups shared a critical weakness: a tendency toward false negatives. LLMs especially were likely to judge a speaker as cognitively healthy if overt linguistic dysfunctions were absent, potentially missing subtler signals. Human annotators showed similar errors, but their judgments were more subjective and less explainable, with poor inter-annotator agreement (Fleiss’ κ = 0.28).
What linguistic cues matter most - and are they understood?
To determine which cues guided decisions, the researchers extracted 38 expert-guided binary features from each transcription using GPT-4o, spanning five categories: linguistic, objective interpretation, subjective interpretation, human experience, and interview context. These were then modeled using logistic regression to evaluate their influence on perception and diagnosis.
The most predictive features for clinical diagnosis included disfluencies, actions over objects, short sentences, and weather references. Interestingly, LLMs also captured subjective and emotional cues, such as sad or lighthearted language, theory of mind references, and self-limitations, which were not typically used by clinicians but may offer valuable context when interpreting signs of decline.
Humans, however, tended to rely on simpler cues such as whether specific characters (like the girl or mother) were mentioned. In misperceived cases, where annotators wrongly labeled dementia patients as healthy, they often used “rich vocabulary” or “outside references” as false signs of cognitive normality. In contrast, LLMs rarely made such errors, suggesting more consistent interpretation across features.
Figure 3 from the study visually compares the weight and direction of significant features for all three perception types. LLMs had stronger alignment with clinical diagnosis than humans, supported by their McFadden’s R² scores: 0.527 for LLM perception vs. 0.058 for human perception, and 0.209 for clinical diagnosis.
Can AI improve early dementia detection and how should it be used?
The study offers a compelling case for integrating LLMs into early dementia awareness frameworks, not as standalone diagnostic tools, but as augmentative systems that guide or challenge human intuition. Given that detection often begins with family members or caregivers, not clinicians, equipping them with AI-assisted tools could bridge the perceptual gap between lay insight and medical expertise.
Moreover, the explainable modeling approach used in this research could be extended to other sensitive domains where interpretability is crucial. The authors argue that, in high-risk settings like dementia care, it is not enough to rely on opaque neural networks. Instead, using transparent methods like logistic regression with interpretable features can foster trust and enable actionable insights.
One key limitation of the study is that it uses binary classification, simplifying the complex spectrum of cognitive decline. This may obscure distinctions between mild cognitive impairment and more advanced dementia. Additionally, while the LLMs demonstrated strong performance, they too exhibited false negative patterns that warrant further refinement, especially in cases where overt dysfunctions are not linguistically obvious.
The researchers propose that future work should expand to include longitudinal analysis and explore whether LLMs, when used continuously, can detect subtle shifts in a person’s language use over time, potentially providing the earliest warnings of neurodegeneration before clinical intervention is even sought.
- FIRST PUBLISHED IN:
- Devdiscourse