Automation reshapes textile waste management as AI sorting systems advance
Studies leveraging NIR and CNNs report accuracies exceeding 95%, with some achieving perfect classification for pure fibers. However, these results typically apply to controlled laboratory environments using relatively small datasets. One standout example involved converting NIR spectral data into pixelated images and processing them through a model called Textile Recycling Net (Tr-Net), which achieved 96.2% accuracy on nine fiber categories.
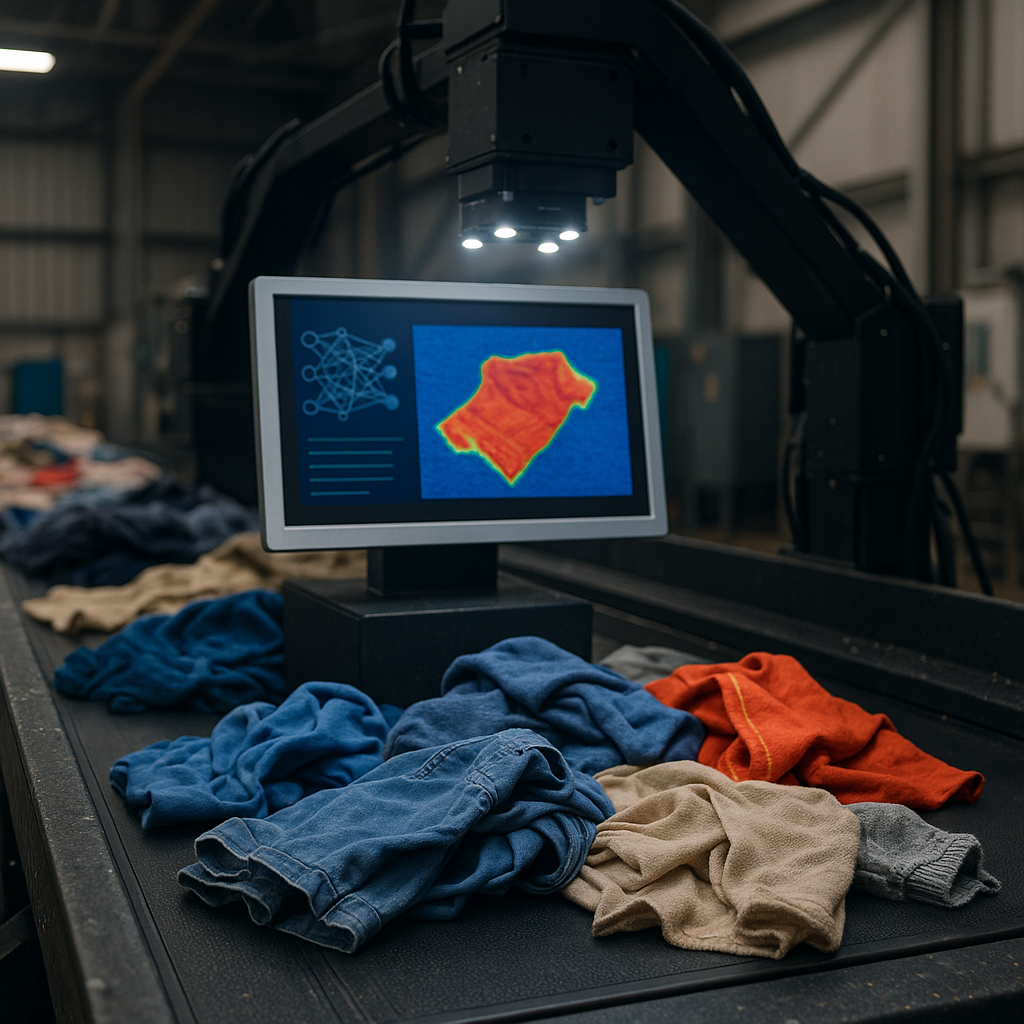
In a significant leap for sustainable manufacturing, artificial intelligence (AI) is increasingly being deployed to transform textile waste sorting - one of the biggest hurdles in closing the loop on garment recycling. A new study, titled “A Systematic Literature Review—AI-Enabled Textile Waste Sorting,” published in Sustainability, provides the most comprehensive analysis to date of how computer-based automation is changing textile waste management. The paper analyzes 18 cutting-edge peer-reviewed studies and identifies core technologies, accuracy benchmarks, current limitations, and future research imperatives for scaling up AI-powered textile sorting systems.
Conducted by researchers from North Carolina State University, the review evaluates three dominant technical methods, spectroscopy, imaging, and hyperspectral imaging (HSI), all integrated with AI frameworks like machine learning, deep learning, and computer vision. The study categorizes these into their industrial capabilities, reviews their impact on classification accuracy, and presents a roadmap for overcoming persistent challenges such as blended fiber identification, material coating interference, and dataset inadequacy.
How do current AI technologies support automated textile waste sorting?
AI-powered textile waste sorting uses computer-driven analysis techniques to classify garments by fiber composition, which is a critical first step for effective recycling. The review outlines spectroscopy-based systems as the most mature and widely studied category. Near-infrared (NIR), Fourier-transform infrared (FTIR), short-wave infrared (SWIR), and Raman spectroscopy are frequently used, often in tandem with models like convolutional neural networks (CNNs), support vector machines (SVMs), and artificial neural networks (ANNs).
Studies leveraging NIR and CNNs report accuracies exceeding 95%, with some achieving perfect classification for pure fibers. However, these results typically apply to controlled laboratory environments using relatively small datasets. One standout example involved converting NIR spectral data into pixelated images and processing them through a model called Textile Recycling Net (Tr-Net), which achieved 96.2% accuracy on nine fiber categories. Yet, performance dropped significantly when the systems encountered blended textiles or aged and coated fabrics, which are common in real-world waste streams.
Imaging-based methods, which use RGB cameras and standard computer vision algorithms, are more cost-effective but generally less reliable. They focus primarily on garment type, shape, or color rather than fiber composition. One study using AlexNet achieved only 68.2% accuracy overall, underscoring the gap between visual classification and material-level identification. Another advanced method incorporating attention mechanisms into CNN architectures reached over 90% accuracy, showing that imaging can improve with enhanced feature recognition.
HSI methods, by contrast, capture both spectral and spatial information, creating three-dimensional data cubes that enable highly precise classification. These systems showed average accuracies nearing 99% and excelled in identifying polyester content in blends. However, their practical adoption is challenged by data processing requirements, cost, and variability introduced by surface features like wrinkles or coatings.
What are the limitations holding back AI-driven sorting systems?
Despite promising developments, key limitations hinder AI’s full-scale deployment in textile waste sorting. The review highlights the failure to accurately classify blended fabrics as the most pressing technical challenge. Even sophisticated models struggle to parse fibers when materials are tightly intertwined, especially when surface-level spectroscopy cannot penetrate to inner layers. This is exacerbated by the structural complexity of some textiles, such as core-sheath yarns, which conceal essential identifying features.
Another constraint is data scarcity. Most models are trained on relatively small and narrow datasets that do not reflect the diverse, contaminated, and unpredictable nature of post-consumer textile waste. Without standardized, expansive, and annotated datasets, models risk overfitting or misclassification, limiting industrial scalability.
Environmental factors like moisture, coatings, and fabric aging further disrupt spectral signals and reduce accuracy. Although some models incorporate preprocessing techniques like external parameter orthogonalization (EPO) or standard normal variate (SNV) normalization to reduce distortion, these interventions are not sufficient to fully mitigate real-world variability.
Moreover, the cost and complexity of hyperspectral systems and industrial-scale spectroscopy equipment remain barriers for many recycling facilities. Imaging methods, while cheaper, are limited in their inability to detect fiber composition—undermining their utility in high-fidelity recycling systems.
What is the path forward for scaling AI in circular textile economies?
The study concludes with a set of focused recommendations aimed at strengthening the robustness and applicability of AI-enabled textile sorting systems. Foremost is the need for standardized, open-access datasets featuring a wide range of fiber types, blend ratios, surface treatments, and wear conditions. Larger datasets would enable the use of more efficient deep learning architectures like self-supervised learning or graph neural networks, which can improve accuracy while reducing dependence on manual labeling.
Hybrid systems that combine multiple data input methods such as spectroscopy for chemical composition and imaging for structural or visual cues are also encouraged. For example, some studies suggest integrating hyperspectral data with machine vision and thermal signatures to improve classification accuracy in complex or coated textiles.
Researchers also emphasize the importance of expanding the fiber coverage in AI models. While polyester and cotton dominate textile production and research focus, fibers like nylon and man-made cellulose are underrepresented despite their prevalence in garments. Accurate classification of these fibers is essential for comprehensive recycling systems.
The study calls for stronger collaborations between academia, industry, and policymakers to standardize protocols and facilitate commercial-scale implementation. In particular, embedding traceable identifiers like photonic crystal fibers during manufacturing could significantly improve downstream sorting efficiency and counterfeit detection.
- READ MORE ON:
- textile waste automation
- automated textile sorting
- AI in textile waste management
- textile waste handling automation
- industrial automation in recycling
- smart waste management systems
- sustainable textile waste solutions
- how automation is changing textile waste management
- AI and robotics for automated textile waste sorting
- automation trends in circular textile economy
- FIRST PUBLISHED IN:
- Devdiscourse