Machine learning model closes weather forecast gaps in India's remote regions
The machine learning model, built using logistic regression, marked a substantial improvement, with 87.35% overall accuracy. Yet it struggled with rain event detection, recording a recall rate of just 4.02%, suggesting it missed most actual rainfall occurrences despite high precision. This revealed the model’s bias toward the more frequent "no rain" outcome, a common problem in imbalanced datasets.
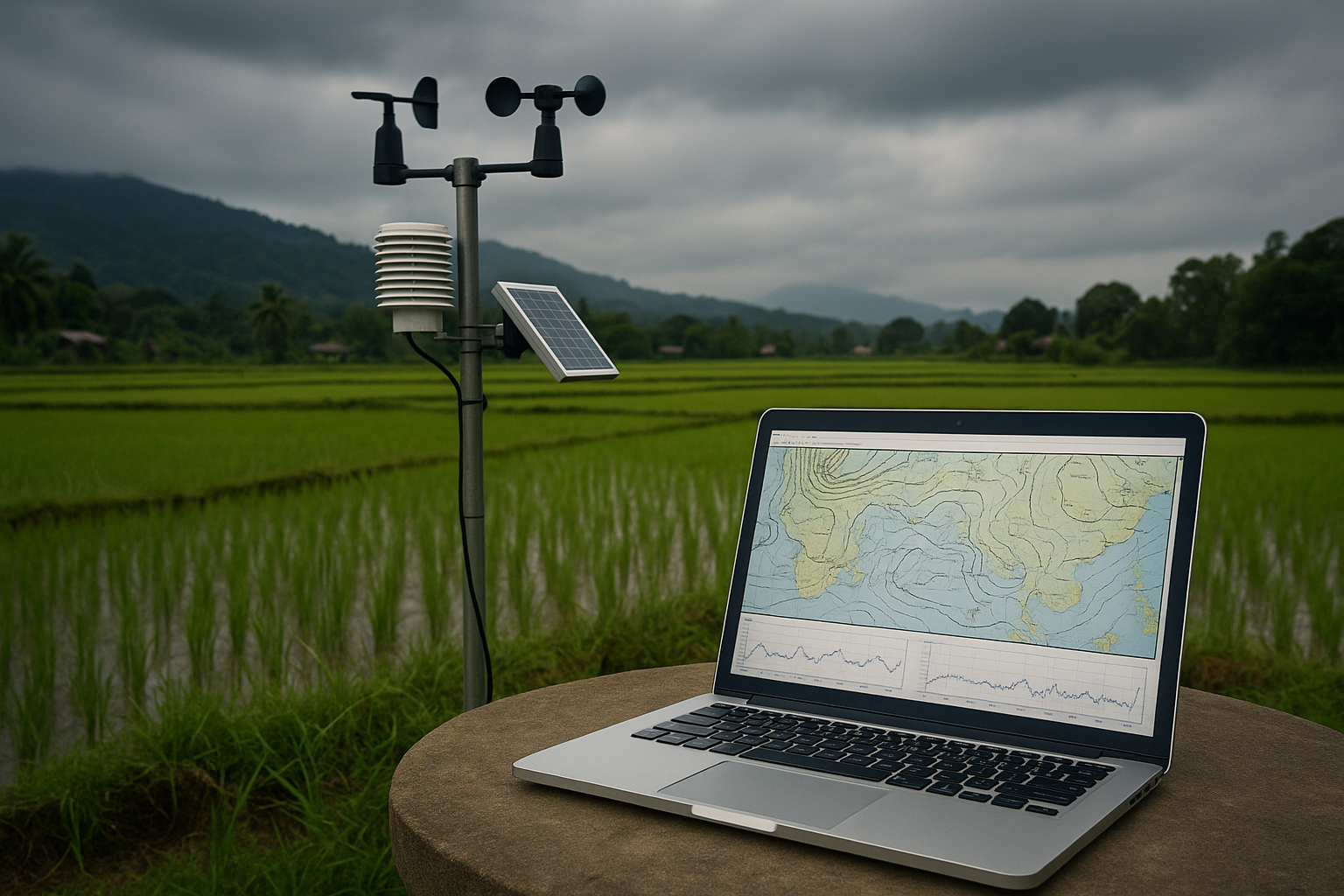
India’s rural and remote regions, home to millions dependent on accurate climate prediction, continue to suffer from unreliable weather forecasts due to poor data coverage. Traditional meteorological models have long struggled in these areas, with sparse observational records leaving large portions of the country vulnerable to extreme weather with minimal warning. A new study points to artificial intelligence as the breakthrough technology to overcome these longstanding data gaps.
Published in Atmosphere under the title “Improving Weather Forecasting in Remote Regions Through Machine Learning,” the study by Kaushlendra Yadav, Saket Malviya, and Arvind Kumar Tiwari introduces a machine learning framework tailored to compensate for missing meteorological data. The study not only presents a rigorous analysis of data scarcity challenges but also provides a tested, scalable AI model capable of improving rainfall prediction accuracy in less-documented Indian territories.
How sparse data undermines forecasting in remote areas
Traditional weather prediction models rely on comprehensive and continuous historical data inputs, often gathered through dense networks of ground-based sensors. However, much of India, especially interior and high-elevation regions, lacks this infrastructure. The authors reviewed the Meteorological Data Supply Portal of the India Meteorological Department and found substantial data availability gaps, particularly in non-urban zones where weather forecasting is most urgently needed for agriculture, disaster preparedness, and daily life.
To bridge this data divide, the study proposes training AI models on well-documented, data-rich cities and transferring the predictive insights to similar but data-sparse regions. This approach hinges on the hypothesis that machine learning algorithms can generalize weather dynamics based on geographical, climatic, and socio-environmental similarities.
Using datasets from 27 global cities, including several in India, the researchers enriched conventional weather variables such as temperature, humidity, and pressure with over 20 city-specific features. These included elevation, proximity to oceans or deserts, population density, industrial activity, and even solar radiation. By integrating these diverse indicators, the model could detect subtle climate correlations often ignored by traditional systems.
Can machine learning and deep learning close the accuracy gap?
To test the efficacy of artificial intelligence in forecasting under low-data conditions, the study evaluated three approaches: a rule-based system, a logistic regression model, and a deep learning neural network.
The rule-based model, based on threshold values for humidity and pressure, achieved an accuracy of only 53.69% in predicting rainfall. Its simplicity could not capture nonlinear dependencies, and it performed poorly in identifying actual rain events.
The machine learning model, built using logistic regression, marked a substantial improvement, with 87.35% overall accuracy. Yet it struggled with rain event detection, recording a recall rate of just 4.02%, suggesting it missed most actual rainfall occurrences despite high precision. This revealed the model’s bias toward the more frequent "no rain" outcome, a common problem in imbalanced datasets.
The deep learning model delivered the most balanced performance. Trained on the enriched dataset, it achieved an overall accuracy of 83%, a 40% precision score for rain prediction, and a recall rate of 68%. The area under the ROC curve stood at 0.86, indicating a strong ability to distinguish between rainy and non-rainy days. The model's capacity to detect true rainfall instances represents a vital advancement for regions prone to sudden monsoons or flash floods, where early warning can save lives.
The study’s neural network employed dense layers with ReLU activation functions and binary cross-entropy loss, revealing improved adaptability to nonlinear and sparse data conditions. The model's robustness was further visualized through ROC and Precision-Recall curves, underlining its suitability for real-world forecasting in uncertain environments.
What are the broader implications for Indian meteorology and policy?
Beyond the algorithmic results, the research makes a compelling case for policy intervention and modernization of India’s forecasting ecosystem. While AI can bridge gaps temporarily, long-term resilience will require systematic investment in data collection infrastructure, particularly in climate-sensitive rural and coastal regions.
The authors advocate for integrating AI-based forecasts into state and national disaster preparedness protocols. Better rainfall prediction directly supports agriculture planning, flood management, and public health initiatives. Furthermore, by incorporating features such as air quality index (AQI) and industrial activity, these models can link environmental and climate insights with urban planning and public policy, opening pathways for climate-resilient development strategies.
Importantly, the study highlights the adaptability of AI frameworks across regions. By training on datasets from documented cities, the model can be deployed in structurally similar but under-monitored regions. This creates opportunities for AI-assisted forecasting in other data-deficient countries facing similar challenges in Africa, Southeast Asia, and Latin America.
Despite its success, the study acknowledges certain limitations. False positives in rain prediction remain a concern, and model performance is still dependent on the quality and granularity of the training data. Future improvements could include the integration of real-time data via IoT sensors, enhanced transfer learning techniques, and dynamic adjustment to climate change–induced weather pattern shifts.
- FIRST PUBLISHED IN:
- Devdiscourse