AI outperforms humans in writing research proposals
Specifically, ChatGPT's proposals were rated as more logically sound, more original, and more meaningful than those of its human counterparts. On a scale with a maximum score of 130, ChatGPT received a median score of 129, while students scored a median of 105. The AI’s experimental procedures were also judged to be more valid and better articulated, often including detailed suggestions such as using statistical software and validated questionnaires - features frequently missing from student responses.
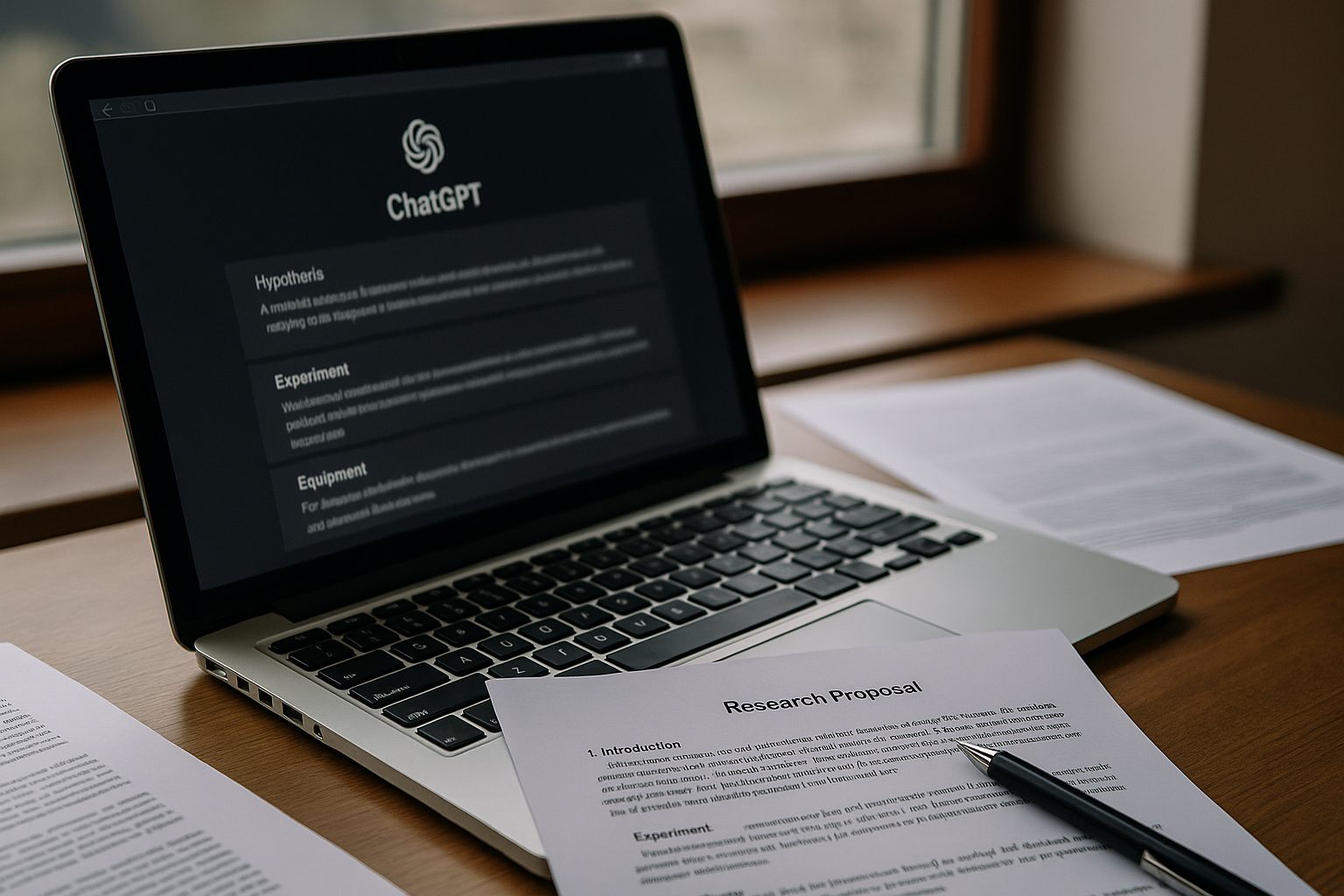
In a development that raises urgent questions about the future of research training, a new study has found that ChatGPT-4 significantly outperforms graduate students in generating original, meaningful, and logically sound scientific proposals. The findings underscore the evolving role of generative AI in academic creativity and challenge assumptions about human dominance in hypothesis formulation and experimental design.
The study, titled “When ChatGPT Writes Your Research Proposal: Scientific Creativity in the Age of Generative AI”, was published in the Journal of Intelligence. Conducted by researchers at the Center for Cognitive Science at the University of Kaiserslautern-Landau, it systematically compared proposals written by graduate students to those generated by ChatGPT in response to a structured research scenario.
Can generative AI really think like a scientist?
To evaluate whether generative AI can perform tasks associated with scientific creativity, researchers designed an experiment mimicking a key component of real academic work: writing a brief research proposal. Ten cognitive science graduate students and ChatGPT-4 were given identical prompts. Participants were asked to generate a testable hypothesis, outline a valid experimental procedure, list necessary equipment, and justify the scientific rationale behind their design.
All responses were evaluated blindly by two senior researchers using a standardized rating tool covering seven creativity criteria: clarity of hypothesis, falsifiability, validity of design, logical reasoning, adequacy of explanation, originality, and meaningfulness. ChatGPT-4 scored significantly higher than the human sample in five of the seven metrics, including overall scientific creativity.
Specifically, ChatGPT's proposals were rated as more logically sound, more original, and more meaningful than those of its human counterparts. On a scale with a maximum score of 130, ChatGPT received a median score of 129, while students scored a median of 105. The AI’s experimental procedures were also judged to be more valid and better articulated, often including detailed suggestions such as using statistical software and validated questionnaires - features frequently missing from student responses.
Where do students still compete and what are the limits of AI creativity?
Despite ChatGPT’s superior quantitative performance, the study reveals subtle qualitative distinctions between human and AI-generated ideas. While ChatGPT maintained a consistent five-step structure in every experiment, often concluding with statistical analysis, it sometimes introduced logical inconsistencies. For example, it included procedural elements like blood sampling that were neither analyzed nor integrated into its conclusions. Such inconsistencies were often overlooked by reviewers due to ChatGPT’s eloquent and technically rich language.
In contrast, human students demonstrated greater procedural variety, including the use of diverse methodologies such as food diaries, blind taste tests, and cross-cultural comparisons. These approaches suggested a broader conceptual scope, even if they were not as precisely framed or articulated as those of the AI.
Furthermore, the study noted a lack of variance in AI-generated answers, raising questions about creativity in the strict sense of producing statistically rare or novel ideas. Because ChatGPT relies on large-scale pattern recognition rather than cognitive intuition, its responses tend to echo high-probability patterns from its training data. This architecture favors repetition over true ideational divergence.
The study also raises the issue of potential plagiarism. While ChatGPT’s answers appear creative, they may in fact be recombinations of previously published scientific ideas, especially given the prevalence of related research in online databases. This challenges the legitimacy of labeling AI-generated outputs as “original” and highlights the importance of transparency regarding training data in generative models.
What does this mean for the future of scientific training?
The implications of this study are profound for education, research policy, and the future of academic assessment. The authors argue that if generative AI can convincingly draft research proposals, universities may need to rethink what constitutes creativity and originality in scientific training. Rather than focusing solely on output fluency, flexibility, or even originality, metrics AI can now excel in, future assessment may need to emphasize ethical reasoning, domain-specific judgment, and the pursuit-worthiness of ideas.
There is also a call for integrating AI into the research training pipeline. Co-creative models, where students collaborate with AI tools, may become a viable path forward. Such integration could allow AI to enhance operational efficiency while humans retain control over vision, ethics, and exploratory intuition.
However, the authors caution against over-reliance. Despite its strengths, ChatGPT lacks self-awareness, ethical judgment, and the capacity to assess the long-term value or potential consequences of its proposals. These limitations make human oversight indispensable, especially in high-stakes or ethically complex research domains.
- READ MORE ON:
- ChatGPT scientific creativity
- ChatGPT research proposal
- AI in scientific research
- artificial intelligence academic writing
- ChatGPT hypothesis generation
- AI-written research proposals
- ChatGPT vs human researchers
- AI for academic proposal writing
- AI in cognitive science research
- AI-generated academic content
- FIRST PUBLISHED IN:
- Devdiscourse