New IAEA-Driven Equation Uncovers Widespread Errors in Dietary Self-Reporting
Published recently in Nature Food, the study reveals that approximately one-third of the records in widely used nutritional datasets may be misreported.
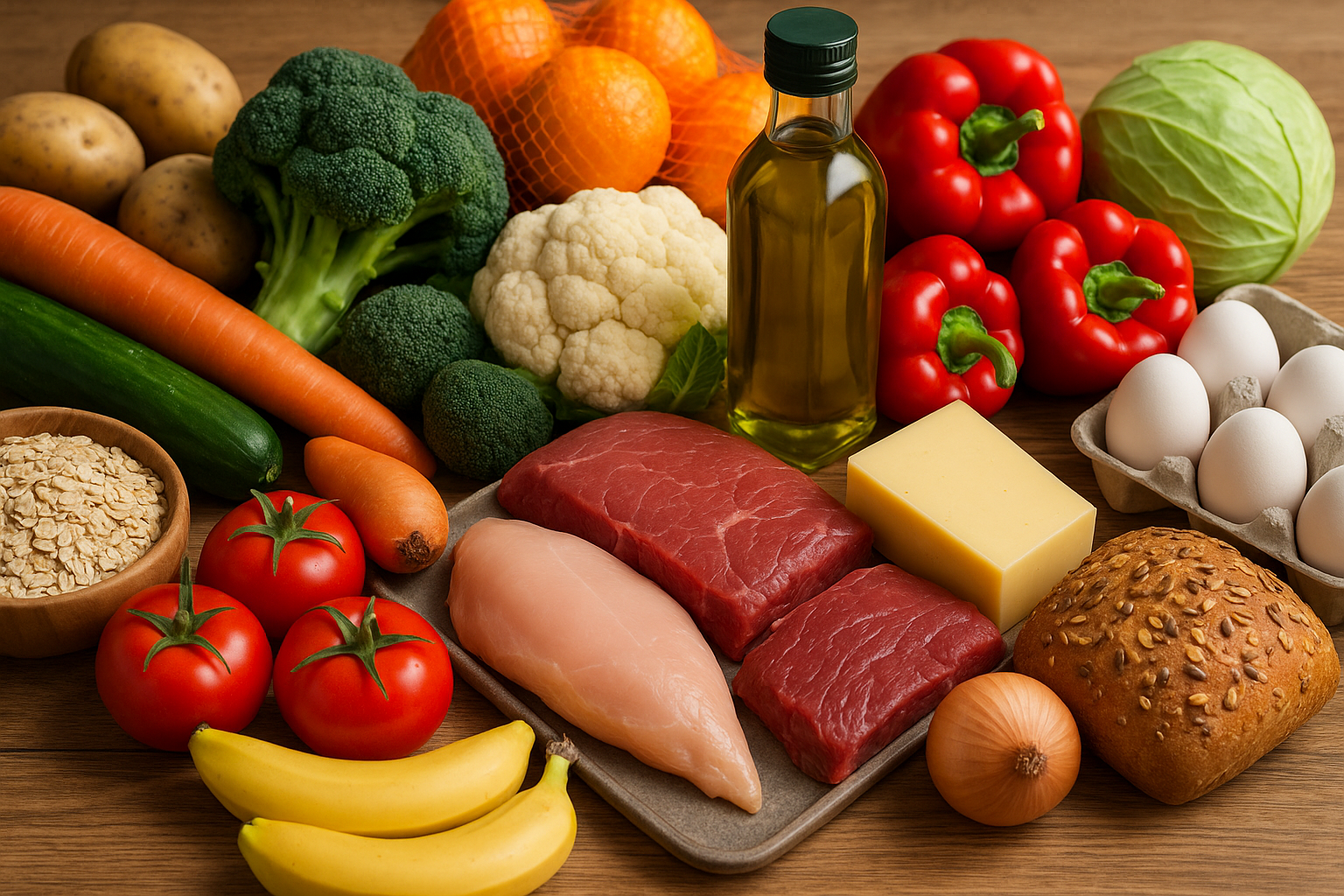
A groundbreaking machine learning-based equation, developed using data from the International Atomic Energy Agency’s (IAEA) extensive nutrition database, is shedding new light on a long-standing issue in nutrition science: the accuracy of self-reported dietary data. This innovation is helping researchers across the globe critically evaluate nutritional records and uncover discrepancies that may be distorting the field of nutritional epidemiology.
Published recently in Nature Food, the study reveals that approximately one-third of the records in widely used nutritional datasets may be misreported. This alarming finding not only confirms suspicions that have lingered for decades but also equips the research community with a robust tool to identify and mitigate the issue.
The Problem with Self-Reporting in Nutritional Epidemiology
Nutritional epidemiology — the study of how diet influences human health and disease — has long relied on self-reported data collected through tools like food diaries, 24-hour recalls, and dietary frequency questionnaires. While these methods are cost-effective and widely accessible, they are also prone to inaccuracies. Individuals may underreport or overreport their food intake, forget what they ate, misestimate portion sizes, or even alter their eating behavior simply because they are being monitored.
“Many nutritional epidemiology studies that try to link dietary exposure to disease outcomes are based on unreliable data, which can explain why many findings contradict each other,” said Professor John Speakman, one of the paper’s authors and a scientist at both the Shenzhen Institute of Advanced Technology in China and the University of Aberdeen in the UK.
Despite being recognized since the 1980s, the issue of dietary misreporting continues to compromise the quality and reliability of diet-disease relationship studies. Until now, the absence of scalable, accurate alternatives has limited researchers’ ability to address this problem.
Doubly Labelled Water: A Gold Standard for Energy Expenditure
Enter the doubly labelled water (DLW) technique. This non-invasive method measures daily energy expenditure by tracking stable isotopes of hydrogen and oxygen in water consumed by individuals. Widely regarded as the "gold standard" for measuring energy expenditure in real-world conditions, DLW provides an objective and highly accurate benchmark against which self-reported energy intake can be compared.
The IAEA’s Doubly Labelled Water Database aggregates over 12,000 individual records of energy expenditure from people aged from infancy to 90 years across 45 countries. This database has been central to multiple landmark studies in human metabolism, including a 2021 article in Science that gained significant international attention.
Alexia Alford, a nutrition specialist in the IAEA’s Division of Human Health, emphasized the database’s value:
“The IAEA Doubly Labelled Water Database is an unparalleled resource that provides invaluable insights into human energy expenditure across the lifespan, playing a critical role in advancing our understanding of metabolism and health.”
Building the Predictive Equation Using Machine Learning
Nearly 100 scientists collaborated globally to harness this vast trove of DLW data. By employing both classical general linear regression models and advanced machine learning algorithms, they derived a predictive equation capable of estimating daily energy expenditure. Key predictors such as body weight, height, age, sex, and even elevation above sea level were incorporated into the model to enhance accuracy.
This equation was validated using portions of the database not used in its initial training phase — a common practice in machine learning to ensure robustness. Once validated, researchers applied the model to external datasets to assess real-world misreporting rates.
One of the datasets analyzed was the UK’s National Diet and Nutrition Survey, which includes more than 12,000 dietary records. The results were telling: only 66.8% of adult dietary reports matched the predicted energy expenditure range, suggesting that 33.2% were likely misreported. Among children, 83.4% of records aligned with the predictions, pointing to a somewhat lower but still significant misreporting rate.
Similar analyses of the U.S. National Health and Nutrition Examination Survey (NHANES) showed that 32.1% of adult reports and 18.3% of children’s reports likely reflected misreporting.
Implications for the Future of Nutrition Science
The implications of this work are profound. It provides researchers with a valuable screening tool to evaluate the credibility of self-reported dietary data, potentially improving the accuracy of nutrition research and public health policy based on it.
“While new methods of dietary intake reporting are actively being developed, none are ready for large-scale implementation just yet,” noted Dr. Cornelia Loechl, Head of the Nutritional and Health-Related Environmental Studies Section in the IAEA.
“In the meantime, the prediction equation based on DLW data can help researchers estimate the extent of misreporting in their studies.”
The equation is already being seen as a transformative addition to the toolbox of nutrition scientists and public health professionals. It helps identify patterns of over- or under-reporting that might skew data interpretations and supports a more evidence-based approach to nutritional recommendations and interventions.
Towards Better Dietary Assessment
While machine learning cannot yet fully replace self-reporting methods, this new equation marks a step forward in mitigating one of their most critical flaws. With growing access to datasets and computational tools, it is increasingly possible to integrate such predictive models into everyday research workflows.
The IAEA’s initiative exemplifies how international collaboration, data transparency, and innovative science can work hand-in-hand to tackle persistent problems in global health research. As tools like this become more widely adopted, the field of nutritional epidemiology is poised to move closer to a more precise understanding of how what we eat influences how we live — and how we die.
Tags: