How digital twin technology is transforming precision farming
One of the main advantages of digital twins is their capacity for predictive modeling. For instance, using weather, soil, and crop data, a DT can forecast optimal irrigation schedules or predict disease outbreaks. This not only boosts productivity but also minimizes environmental impact through more efficient resource use. Moreover, DTs allow for scenario testing without real-world risk, enabling farmers to explore outcomes of different crop management strategies before implementation.
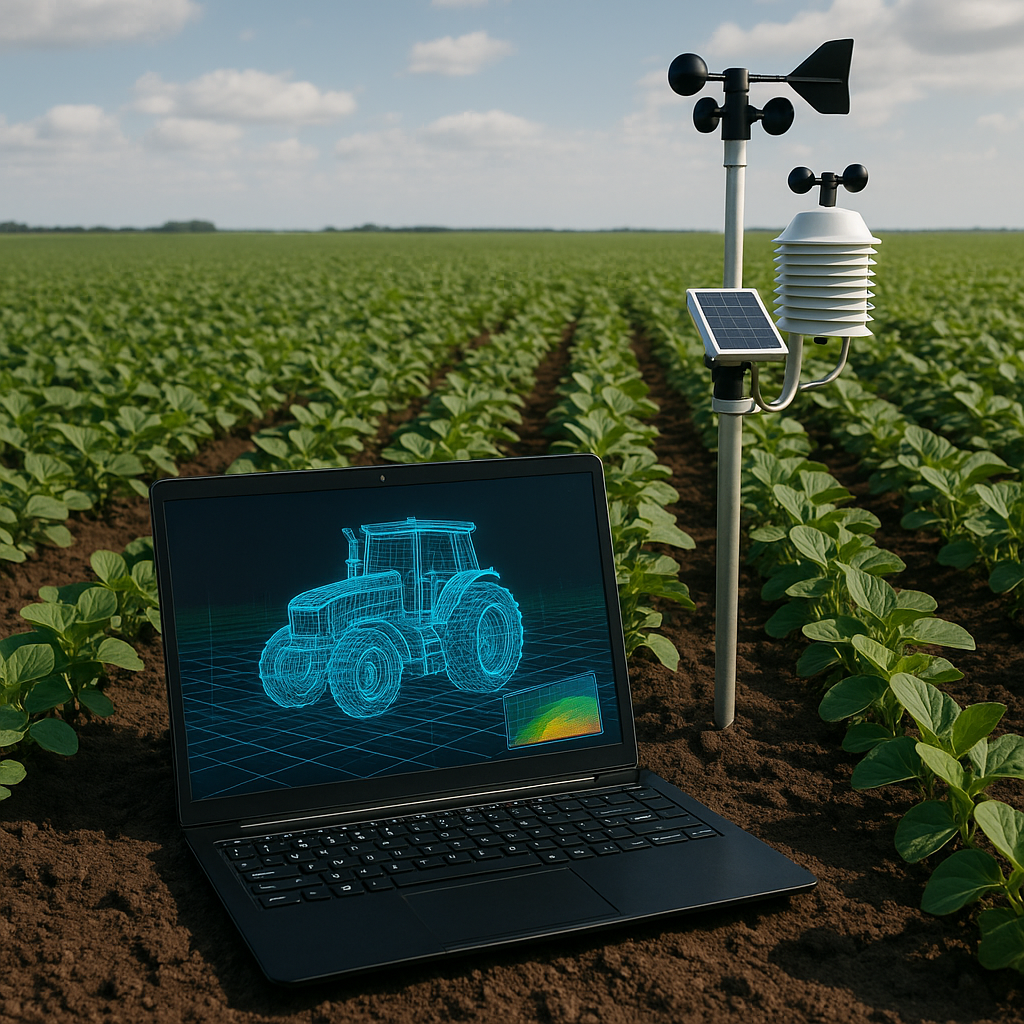
Digital twin technology is fast becoming a cornerstone in the modernization of agriculture, offering real-time synchronization between physical farming assets and their virtual counterparts. This technological evolution is transforming agricultural management into a more data-driven, predictive, and adaptive process.
A recent study titled "A Comprehensive Review of Digital Twins Technology in Agriculture," published in Agriculture, provides an in-depth analysis of how digital twins (DTs) are reshaping the agricultural sector. The study consolidates existing research and use cases to explore DTs' structure, enabling technologies, applications, and future prospects in smart farming.
What makes digital twins a transformative force in agriculture?
At their core, digital twins are dynamic digital replicas of physical entities, continuously updated with real-time data from sensors, drones, IoT devices, and other monitoring systems. These twins offer a bidirectional flow of information: they not only reflect the current state of the physical system but also simulate and predict future scenarios through modeling and analytics.
The study outlines five key components of a digital twin in agriculture: the physical entity (e.g., crops, machinery, livestock), the virtual entity (its digital model), data acquisition systems, data processing and analytics, and services or applications such as visualization, decision support, and automation. This integration facilitates precision agriculture by enabling farmers to make informed decisions on irrigation, fertilization, harvesting, and disease management.
One of the main advantages of digital twins is their capacity for predictive modeling. For instance, using weather, soil, and crop data, a DT can forecast optimal irrigation schedules or predict disease outbreaks. This not only boosts productivity but also minimizes environmental impact through more efficient resource use. Moreover, DTs allow for scenario testing without real-world risk, enabling farmers to explore outcomes of different crop management strategies before implementation.
The use of DTs in greenhouse farming, aquaponics, livestock monitoring, and agricultural robotics demonstrates their wide applicability. In controlled environments like greenhouses, DTs adjust lighting, temperature, and humidity based on crop needs. In livestock management, they monitor animal health, behavior, and welfare in real-time, reducing the risk of disease and improving yields.
How are enabling technologies shaping the development of digital twins?
The effectiveness of digital twins in agriculture depends heavily on supporting technologies. The study identifies the Internet of Things (IoT) as a foundational element. IoT sensors capture vast amounts of real-time data on temperature, moisture, nutrient levels, and other variables crucial for plant and animal health. This data feeds into the digital model, keeping it updated and reflective of physical reality.
Cloud computing and edge computing also play vital roles. Cloud platforms offer scalable storage and computing resources needed to process and analyze large datasets, while edge computing allows for local processing near the data source. This hybrid approach ensures both speed and scalability, essential for time-sensitive agricultural operations.
Artificial intelligence (AI) and machine learning (ML) are indispensable for processing data and generating actionable insights. These algorithms enable pattern recognition, anomaly detection, predictive analytics, and automation. For example, AI can detect subtle changes in plant coloration from drone imagery, signaling early disease onset before it becomes visible to the human eye.
Digital twin modeling also benefits from advanced visualization tools such as augmented reality (AR) and virtual reality (VR), which allow farmers and stakeholders to interact with the digital environment in immersive ways. Blockchain is another emerging tool for enhancing transparency and traceability in the agricultural supply chain, although its integration with DTs is still in the exploratory phase.
Despite these advances, the study notes that interoperability remains a major challenge. Agricultural systems often involve heterogeneous devices and data standards, complicating seamless integration. To address this, open-source platforms and standardization frameworks are being developed, but widespread adoption is still a work in progress.
What barriers must be overcome to fully deploy digital twins in agriculture?
The study acknowledges that while digital twins offer tremendous promise, several barriers hinder their widespread adoption. Chief among these is the high cost of implementation. Building a functional digital twin requires investment in hardware, software, skilled personnel, and maintenance. For smallholder and resource-constrained farmers, these costs can be prohibitive.
Data-related challenges also loom large. Many agricultural regions lack the infrastructure for reliable internet connectivity, limiting real-time data transmission. Moreover, issues around data privacy, security, and ownership are increasingly pressing as more farm operations become digitized. Farmers may hesitate to adopt DTs without clear legal frameworks and data governance policies in place.
Another critical concern is the complexity of model development and validation. Creating accurate digital models that reflect real-world behavior involves sophisticated simulation and calibration techniques. Errors in modeling can lead to flawed predictions, potentially harming crops or livestock. The study urges collaboration between domain experts and technology developers to ensure agricultural validity in digital twin design.
Furthermore, the study calls for more real-world case studies and pilot projects to validate the effectiveness of digital twins across various agricultural domains and climatic regions. Most current implementations are experimental or localized, with limited scalability. Expanding these efforts will help build a more robust evidence base and drive adoption.
To advance digital twin technology in agriculture, the authors recommend a multi-pronged approach: increased public and private investment, development of modular and scalable DT platforms, integration with decision support systems, and policy interventions to ensure equitable access and data protection. Training programs for farmers and extension workers will also be crucial to bridge the digital literacy gap.
- READ MORE ON:
- Digital twins in agriculture
- Smart farming technology
- Precision agriculture 2025
- Agricultural digital twin systems
- Agriculture 4.0 innovations
- IoT in smart farming
- Farming with digital twins
- Cloud and edge computing in agriculture
- How digital twins improve farm productivity
- real-time data in agriculture
- Future of agriculture with digital twins and AI
- FIRST PUBLISHED IN:
- Devdiscourse