ChatGPT’s grammar judgments mirror humans, but with statistical twist
Despite its impressive performance, ChatGPT exhibited systematic deviations in specific areas. It showed difficulty distinguishing between marginally grammatical and fully ungrammatical constructions and occasionally misclassified subtle syntactic phenomena. These patterns suggest that while ChatGPT captures broad patterns of English grammar, it lacks the nuanced, theory-driven filters used by professional linguists. The study concludes that ChatGPT's grammaticality judgments emerge from data-driven exposure rather than from a true competence model of language.
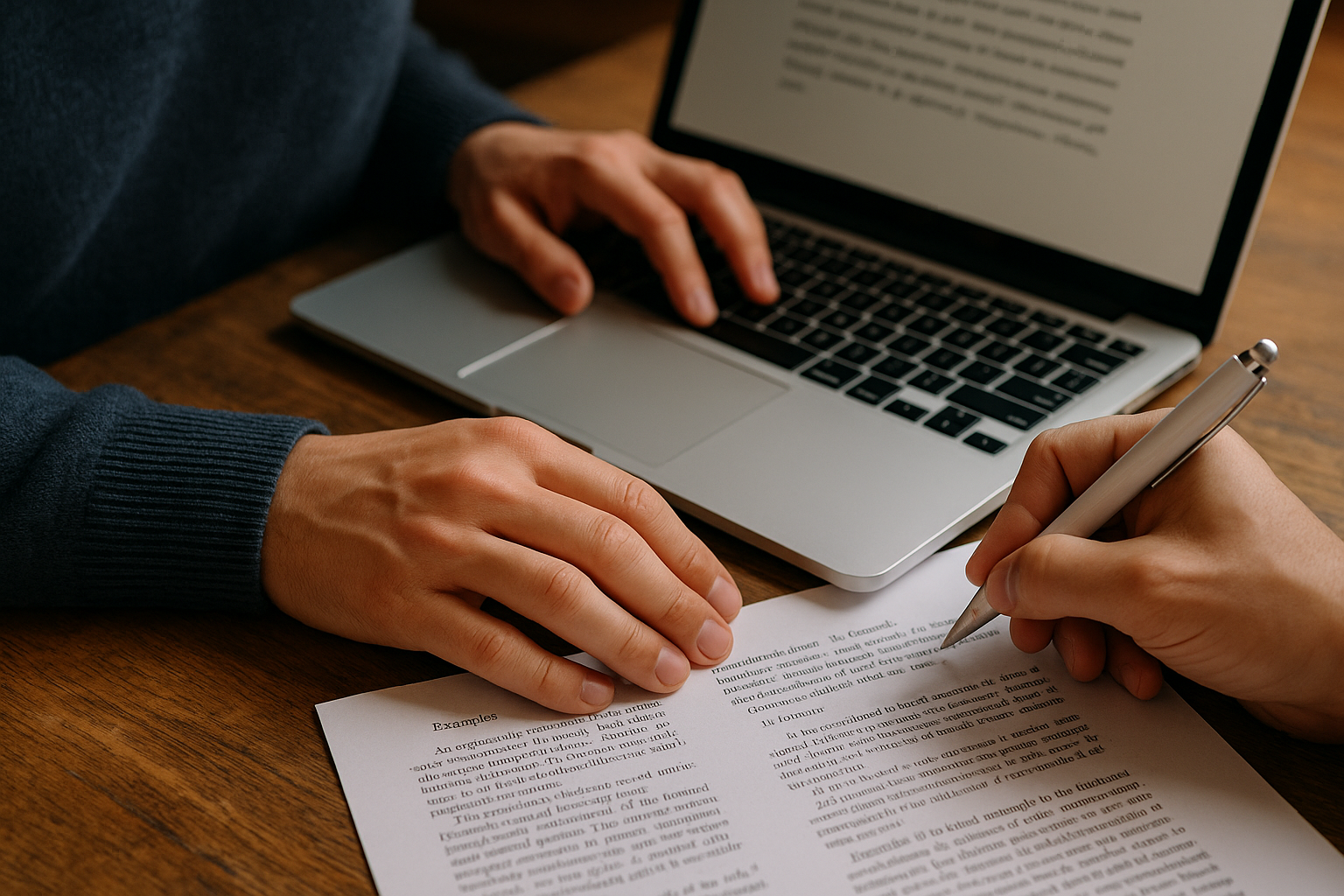
As large language models like ChatGPT become increasingly embedded in tools used by educators, linguists, and the general public, new research has examined how these systems represent and judge grammaticality and how that compares to human intuition.
A study titled "Grammaticality representation in ChatGPT as compared to linguists and laypeople," published in Humanities and Social Sciences Communications, provides the first large-scale investigation of ChatGPT's grammatical judgment capabilities. The findings offer important insights into whether ChatGPT's internal representations align more closely with linguistic experts or with everyday language users.
How well does ChatGPT replicate human grammatical judgment?
The study evaluated ChatGPT-3.5 across three different grammaticality judgment tasks using a dataset of 148 sentence constructions previously rated by linguists and laypeople. In Experiment 1, ChatGPT was asked to rate grammaticality based on a reference sentence. In Experiment 2, it used a 7-point acceptability scale. In Experiment 3, it was asked to select the more grammatical sentence from a pair. Across all tasks, ChatGPT showed high convergence with linguist judgments, with similarity rates ranging from 73% to 95% and an overall estimate of 89%.
Interestingly, the model's performance also correlated with laypeople's responses, although the strength of this correlation varied depending on the task. This dual alignment suggests that ChatGPT integrates both formal syntactic patterns found in written language and probabilistic cues derived from colloquial usage. In other words, it seems to blend academic grammar and public intuition, sometimes aligning with experts, and sometimes with everyday speakers.
Despite its impressive performance, ChatGPT exhibited systematic deviations in specific areas. It showed difficulty distinguishing between marginally grammatical and fully ungrammatical constructions and occasionally misclassified subtle syntactic phenomena. These patterns suggest that while ChatGPT captures broad patterns of English grammar, it lacks the nuanced, theory-driven filters used by professional linguists. The study concludes that ChatGPT's grammaticality judgments emerge from data-driven exposure rather than from a true competence model of language.
Does ChatGPT lean toward expert norms or everyday language use?
One of the study’s most revealing findings is that ChatGPT occupies a middle ground between the strictness of linguists and the variability of laypeople. The authors attribute this to the psychometric nature of the judgment tasks and to the way large language models process language. Unlike humans, who rely on cognitive and theoretical representations, ChatGPT’s grammaticality ratings are shaped by token-level probability distributions informed by large-scale internet training data.
This means that when ChatGPT finds a sentence likely in its training corpus, it tends to judge it as more acceptable, even if it would be rejected by linguists applying stricter syntactic rules. Conversely, rare but structurally valid sentences may be misjudged as ungrammatical. The model does not possess an abstract representation of grammar rules but instead relies on statistical regularities in surface patterns.
The authors also observe that task structure influences performance. For example, when ChatGPT is asked to choose between two sentences, it performs better than when it must assign absolute scores. This mirrors findings in human cognition, where comparative judgments are often more reliable than scalar ratings. These observations have implications for how we design evaluation tasks to probe the capabilities of AI language models.
Moreover, the study underscores that ChatGPT's performance may reflect an implicit blend of prescriptive and descriptive norms. In some cases, the model appears to "hedge" by avoiding extreme scores, which could reflect both caution in uncertain cases and a lack of deep syntactic parsing. These tendencies, while not errors per se, indicate that LLMs like ChatGPT operate with a fundamentally different logic than human grammatical competence.
What do the findings mean for linguistics and AI development?
The study opens new avenues for understanding how artificial systems acquire and represent linguistic knowledge. The authors argue that ChatGPT's performance supports the idea that grammaticality can emerge from usage-based exposure rather than from built-in grammatical rules. This challenges traditional generative models of syntax, which posit that grammar is an abstract, innate system separate from actual language use.
For linguists, the results suggest that language models may serve as useful proxies for exploring patterns of acceptability across diverse speaker populations. ChatGPT’s responses can help reveal which constructions are robustly learned from data and which require explicit instruction or theoretical grounding. The model’s hybrid alignment with both experts and laypeople may also be leveraged to develop educational tools, corpus analyses, and hypothesis testing frameworks.
From a computational perspective, the research suggests that while LLMs can match human performance on many syntactic tasks, they remain probabilistic systems without true grammatical competence. This limits their explanatory power but enhances their utility in applied domains. For example, in writing assistance, conversational agents, or second-language instruction, a model that reflects both formal correctness and common usage may be more useful than one that rigidly enforces grammatical rules.
The authors call for further interdisciplinary collaboration to refine how linguistic theory and AI systems interact. By systematically testing models like ChatGPT against curated linguistic benchmarks, researchers can better understand both human cognition and artificial generalization. Additionally, future research could explore how newer models such as GPT-4 perform on similar tasks, especially with targeted prompting or fine-tuning.
- FIRST PUBLISHED IN:
- Devdiscourse