Automated ML and biomimicry advance sustainable wastewater reclamation
The study demonstrates that machine learning algorithms, inspired by biological morphological evolution, significantly improve the classification of influent characteristics. This allows for precise adjustments to the treatment process, ensuring that the effluent meets the specific quality requirements for various reuse applications. The researchers achieved a robust F1 score of 0.91 for influent classification, which highlights the algorithm's accuracy in predicting wastewater characteristics and optimizing treatment protocols.
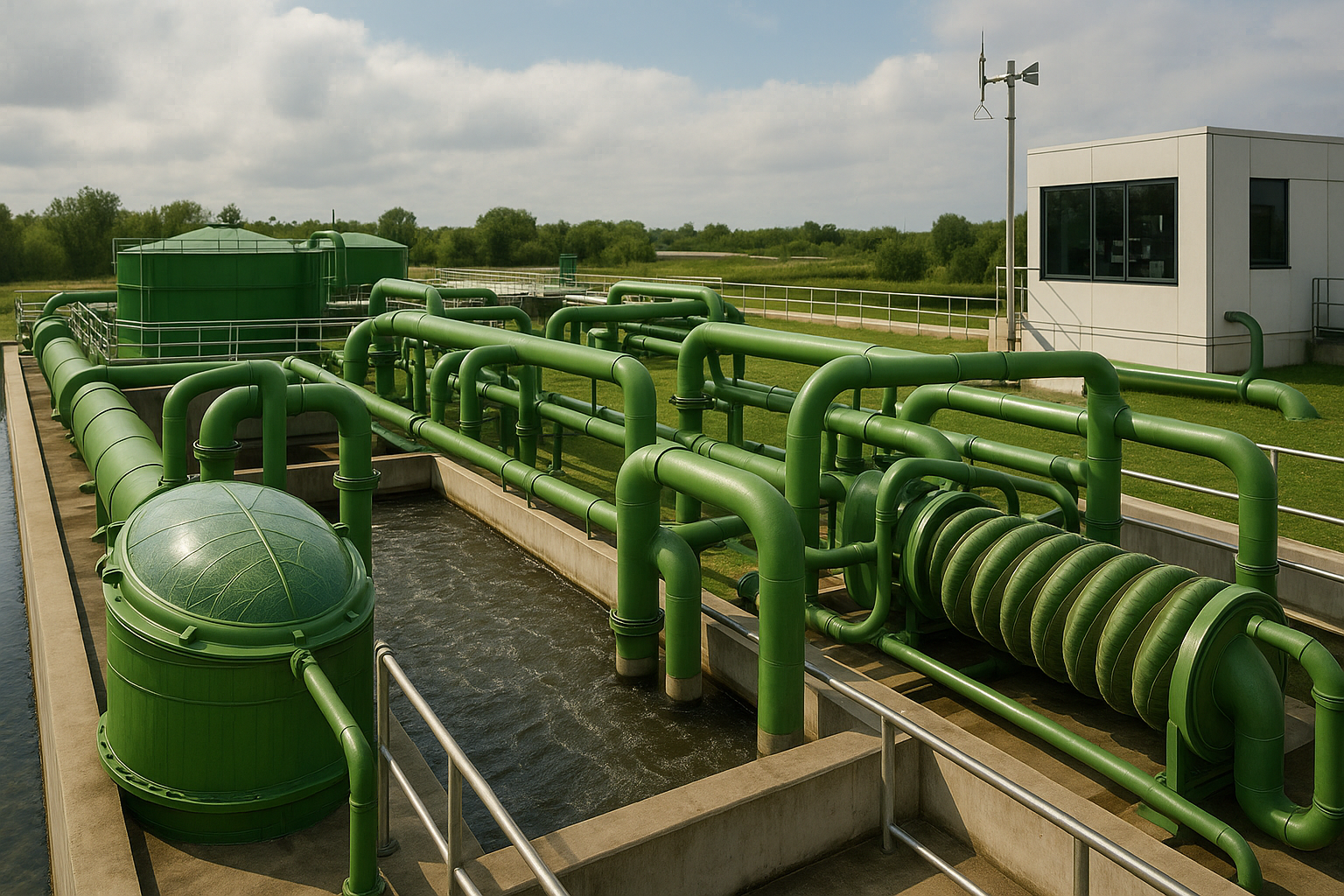
A new study titled "Biomimicry-Inspired Automated Machine Learning Fit-for-Purpose Wastewater Treatment for Sustainable Water Reuse," published in Water in May 2025, proposes an innovative approach to wastewater treatment that could play a crucial role in addressing the growing global concern of freshwater scarcity. The study explores the use of biomimicry-inspired automated machine learning (AML) to adapt wastewater treatment processes to fluctuating influent conditions, ensuring sustainable water reuse in a variety of industrial and agricultural applications.
The researchers have developed a novel system that utilizes decentralized swarm intelligence, known as the Respected Parametric Insecta Swarm (RPIS), to manage the variability in wastewater qualities. RPIS mimics the natural processes seen in biological systems, where local agents interact without a central control, using feedback mechanisms to adjust and optimize the treatment process in real time. This approach is grounded in the principles of biomimicry, leveraging the adaptive and self-regulating behaviors observed in nature.
A key feature of the RPIS system is its bio-inspired oscillatory control algorithms, which maintain stability in effluent quality despite fluctuations in influent conditions. The system’s ability to respond dynamically to changes in real-time is enhanced by adaptive sensor feedback, which optimizes operational parameters without requiring centralized oversight. This decentralized approach not only improves the system's efficiency but also reduces the need for continuous manual intervention, making it a cost-effective solution for sustainable water management.
The study demonstrates that machine learning algorithms, inspired by biological morphological evolution, significantly improve the classification of influent characteristics. This allows for precise adjustments to the treatment process, ensuring that the effluent meets the specific quality requirements for various reuse applications. The researchers achieved a robust F1 score of 0.91 for influent classification, which highlights the algorithm's accuracy in predicting wastewater characteristics and optimizing treatment protocols.
The researchers also explored the environmental benefits of their approach, particularly in terms of reducing resource consumption. The implementation of bio-inspired membranes with selective permeability was shown to minimize fouling, reducing chemical consumption by approximately 11%. Additionally, the study found that the RPIS-based system reduced energy consumption by 14%, demonstrating a clear advantage over traditional methods in terms of operational efficiency and sustainability.
The study's findings have significant implications for wastewater treatment processes, particularly in the context of water reuse in irrigation, cooling towers, and aquifer recharge. The fit-for-purpose nature of the RPIS system allows for tailored treatment solutions, optimizing effluent quality for specific reuse needs. For instance, irrigation applications require moderate nutrient removal and stringent pathogen reduction, while industrial reuse applications demand clearer effluent with lower nutrient levels. The system’s flexibility and adaptive capacity make it suitable for a wide range of water reuse scenarios.
One of the notable advantages of this approach is its scalability. The researchers used machine learning to optimize chemical dosing and energy input based on real-time data, significantly reducing the overall carbon footprint of wastewater treatment facilities. Furthermore, the system's ability to predict and prevent equipment failures through predictive maintenance ensures the longevity of the treatment infrastructure, reducing downtime and maintenance costs.
Despite these promising results, the study highlights several challenges that must be addressed before this technology can be widely implemented. The researchers acknowledge that the system’s performance can be impacted by sensor data ambiguity and the complexity of scaling decentralized swarm intelligence algorithms. These issues need to be further explored to ensure that the system can operate efficiently in large-scale applications.
Another area for future research is the validation of bio-inspired membranes. While the study demonstrated that these membranes exhibit minimal fouling over extended periods, further experimental data is needed to confirm their long-term performance in real-world conditions. Similarly, while the RPIS system has shown reductions in energy and chemical usage, pilot trials are needed to confirm the predicted savings and assess the system’s overall cost-effectiveness.
- READ MORE ON:
- Biomimicry-inspired wastewater treatment
- Automated machine learning for wastewater
- Sustainable water reuse technologies
- Bio-inspired membranes for wastewater treatment
- Machine learning in water reclamation
- AI and biomimicry for sustainable water management
- Wastewater treatment system energy savings
- FIRST PUBLISHED IN:
- Devdiscourse