Performance and transparency drive trust in generative AI
The study identifies five key antecedents of cognitive trust in generative AI: performance expectancy, perceived transparency, social influence, perceived anthropomorphism, and trust propensity. Each of these factors plays a statistically significant role in shaping how users perceive and cognitively evaluate the reliability and trustworthiness of AI-generated content.
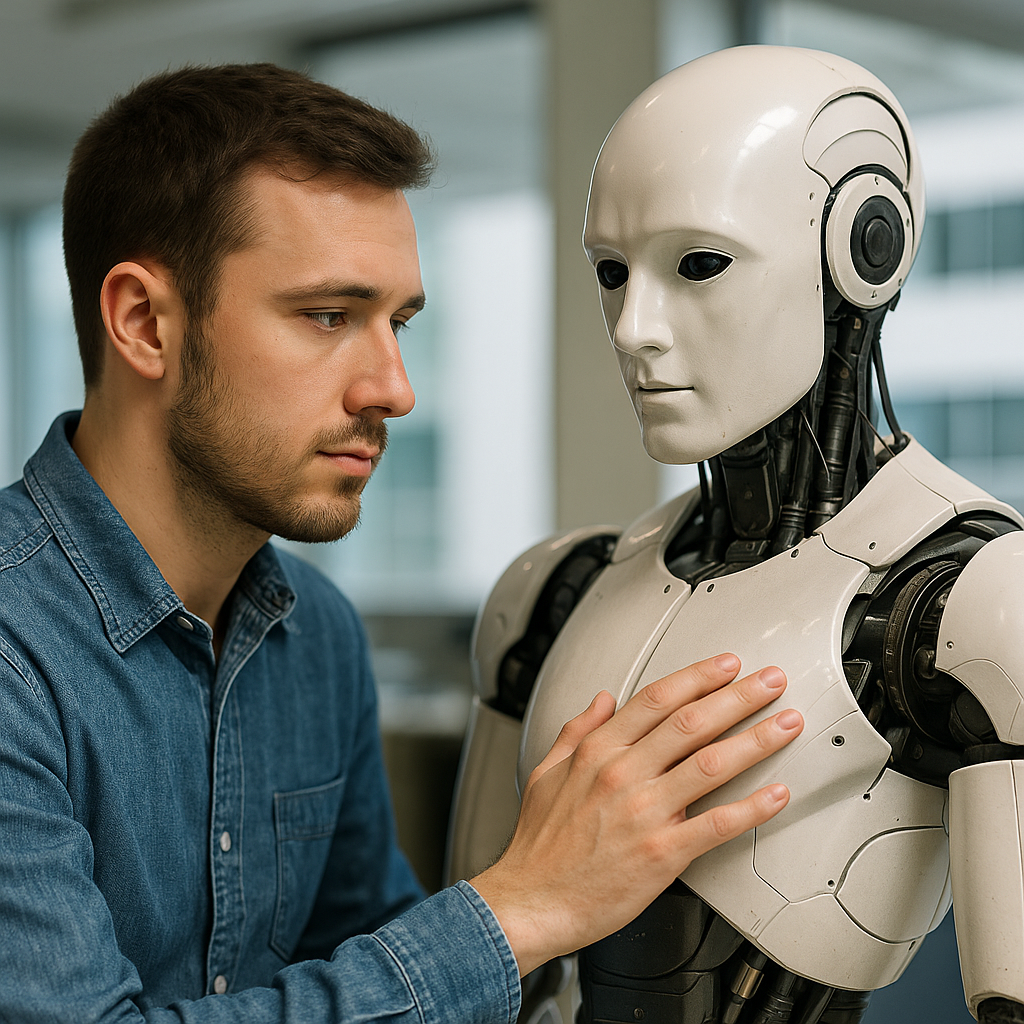
The formation of trust in emerging technologies like generative artificial intelligence has emerged as a decisive factor in determining long-term user engagement. A new peer-reviewed study titled “In generative artificial intelligence we trust: unpacking determinants and outcomes for cognitive trust”, published in AI & Society, offers one of the first empirically validated models to explain how users build trust in generative AI and how that trust influences behavior.
The study addresses a central knowledge gap in the accelerating deployment of tools like ChatGPT, DALL·E, and other text-to-image or text-to-text AI systems: What determines users’ cognitive trust in generative AI, and how does this trust translate into user satisfaction and continued usage? In tackling these questions, authors Minh‑Tay Huynh and Thomas Aichner apply a rigorous structural equation modeling (SEM) analysis to data from 303 respondents, constructing and testing a theoretical model grounded in established technology acceptance and trust theories.
The study identifies five key antecedents of cognitive trust in generative AI: performance expectancy, perceived transparency, social influence, perceived anthropomorphism, and trust propensity. Each of these factors plays a statistically significant role in shaping how users perceive and cognitively evaluate the reliability and trustworthiness of AI-generated content.
Among these, performance expectancy, users’ belief that the system will fulfill its intended function effectively, was found to be the strongest predictor of trust. This reflects a core expectation that generative AI must provide accurate, relevant, and high-quality outputs to gain and retain user confidence. The research demonstrates that trust hinges not on novelty or entertainment, but on consistent, goal-aligned performance.
Perceived transparency, users’ sense of clarity about how the AI operates, was also shown to have a significant impact on cognitive trust. When users understand or believe they understand how a model arrives at its output, their trust increases. The authors argue that transparency is not only an ethical or regulatory consideration, but also a psychological catalyst for trust formation. The implication is that design choices involving explainability features, algorithmic openness, and data source disclosures directly influence trust dynamics.
Social influence, the extent to which users are affected by the opinions and usage behavior of others, also plays a meaningful role. The study finds that peer recommendations, societal discourse, and community-level validation contribute to a collective sense of AI trustworthiness. Trust, in this case, is partly a socially conditioned phenomenon, affected by what others believe and do rather than just personal experience.
The authors also include perceived anthropomorphism as a variable, reflecting whether users view generative AI systems as human-like in communication, style, or behavior. While this was found to be a less dominant factor, it still contributes to trust formation by enhancing perceived relational affinity. However, the study cautions against over-reliance on anthropomorphic cues, which may create misleading impressions of agency or competence.
Finally, trust propensity, a user’s general disposition to trust technology or systems, acts as a background moderator. Individuals who are more inclined to trust in general are also more likely to develop cognitive trust in GAI. While this factor is intrinsic and difficult to influence directly, its role highlights the psychological baseline that users bring into their interaction with AI tools.
The study goes further by exploring the outcomes of cognitive trust. Specifically, it finds that cognitive trust significantly predicts both user satisfaction and the intention to continue using generative AI. These relationships are robust and statistically validated, reinforcing the practical importance of trust in shaping long-term adoption patterns. Satisfaction is not merely a function of output quality but is mediated by trust in the system’s reliability and integrity.
To construct their model, Huynh and Aichner employed partial least squares structural equation modeling (PLS-SEM) and validated all constructs for reliability, convergent validity, and discriminant validity. The analysis confirms that each hypothesized path, except one, was statistically significant. The exception was the direct relationship between anthropomorphism and satisfaction, which did not meet the threshold for significance, indicating that human-like traits alone do not necessarily drive satisfaction if trust is absent.
The theoretical contribution of the study is twofold: it refines our understanding of cognitive trust as distinct from affective or emotional trust, and it offers a testable model for future research and industry application. The model integrates and extends constructs from the Unified Theory of Acceptance and Use of Technology (UTAUT), trust literature, and emerging AI-specific research, making it a foundational reference point for studies on human-AI interaction.
For policymakers, designers, and developers, the implications are immediate. Trust cannot be treated as an abstract ideal, it is shaped by tangible system attributes and user experience. Enhancing perceived performance and transparency, enabling positive social validation, and avoiding manipulative anthropomorphism are practical interventions that can improve trust levels and ensure ethical adoption.
The authors recommend that future research explore longitudinal dynamics of trust, potential cultural differences in trust determinants, and the impacts of negative user experiences such as misinformation, hallucination, or bias in AI outputs. These dimensions, while beyond the scope of the current study, represent critical frontiers in the responsible deployment of generative AI.
- FIRST PUBLISHED IN:
- Devdiscourse