Brain-sensing AI could be the future of road safety
The study’s neuroimaging analysis revealed distinct activation patterns associated with distraction. Using cortical activation maps, researchers found that distraction significantly increased oxygenated blood flow (∆HBO₂) in the dorsolateral prefrontal cortex (DLPFC) and premotor cortex. These areas are responsible for executive functions, attention regulation, and motor planning - key faculties in driving.
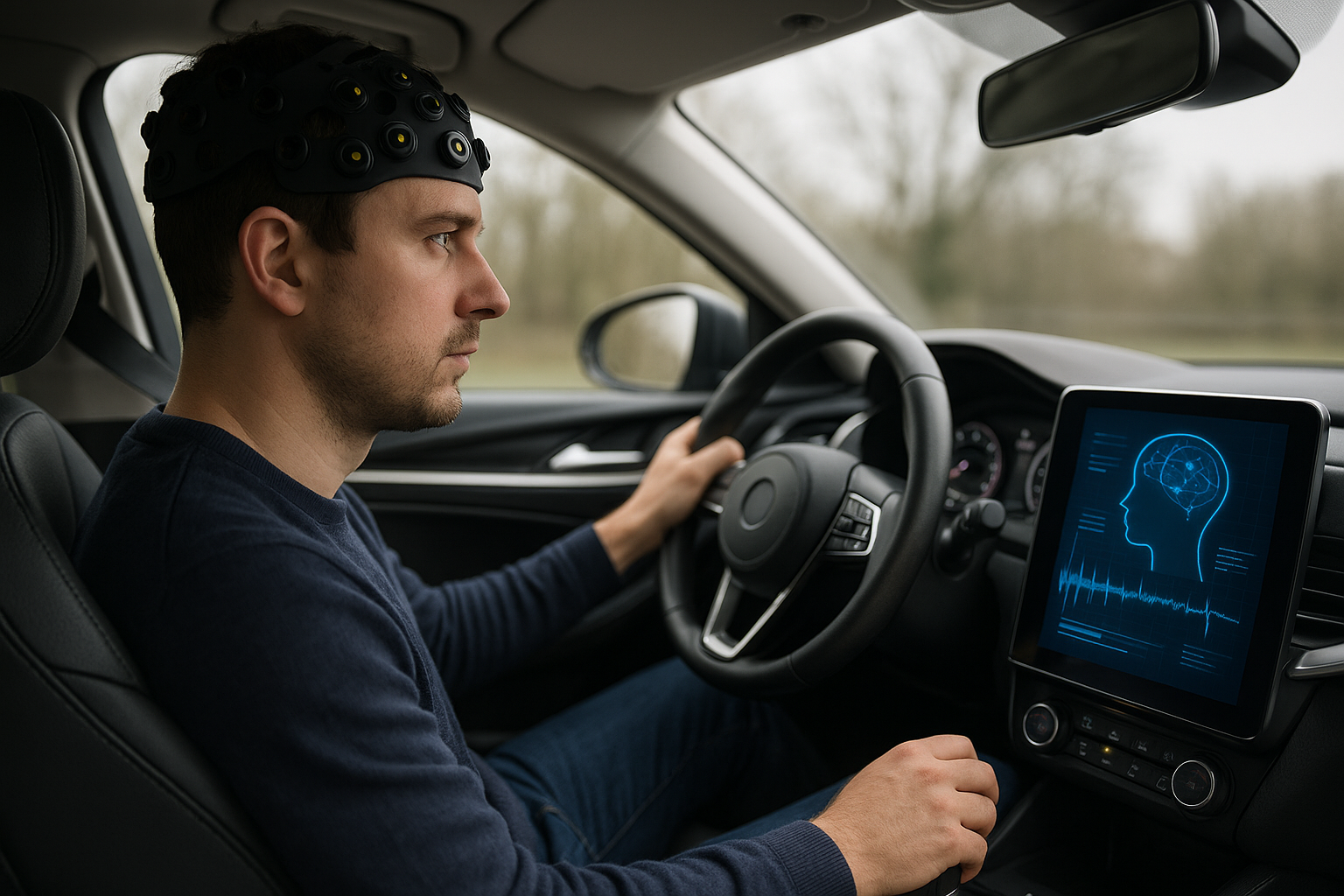
As traffic fatalities from inattention rise globally, researchers have developed a new AI-powered system capable of detecting driver distraction based on real-time brain activity. The study, titled “NeuroSafeDrive: An Intelligent System Using fNIRS for Driver Distraction Recognition”, was published in Sensors in May 2025. Using functional near-infrared spectroscopy (fNIRS) and machine learning, the system classifies drivers’ attention levels by monitoring brain oxygenation, offering a promising tool for the next generation of intelligent transportation systems.
The researchers tested the model on 21 participants in a controlled driving simulator using real-world distraction scenarios, including music selection, text reading, and virtual conversation. By measuring haemodynamic responses across the prefrontal cortex, the system achieved nearly 78% accuracy in detecting transitions between relaxed and distracted driving states. This breakthrough could support proactive safety interventions in semi-autonomous vehicles and improve public road safety.
How does the NeuroSafeDrive system detect distraction?
The NeuroSafeDrive framework combines neuroimaging and AI by leveraging fNIRS technology, which measures changes in blood oxygenation to assess cognitive workload. Participants wore a 24-channel fNIRS headcap while driving in a simulated environment across three road types, urban, urban with intersections, and highways, under both normal and distracted conditions.
Three types of secondary tasks were used to simulate distractions: answering audio-based true/false questions (cognitive), selecting songs from a music playlist (visual-manual), and reading SMS messages (visual-cognitive). These tasks were randomly presented during different road scenarios to evaluate brain responses under varied conditions.
The researchers focused on three brain signal types:
-
∆HBO₂ (oxygenated haemoglobin)
-
∆HHB (deoxygenated haemoglobin)
-
∆HBO₂HHB (combined signal)
Each signal was segmented into multiple time windows (1–60 seconds) and processed using a joint mutual information (JMI) algorithm for feature selection. Machine learning classifiers, Support Vector Machines (SVM), K-Nearest Neighbors (KNN), and Decision Trees (DT), were then trained to differentiate between distraction levels.
The results showed that ∆HBO₂HHB was most effective for detecting distracted driving when compared to a resting state, while ∆HBO₂ alone was better at distinguishing between normal and distracted driving sessions. Among all models tested, SVM consistently outperformed others, achieving 77.9% accuracy for distracted vs. relaxed states.
What brain regions and signals indicate driver inattention?
The study’s neuroimaging analysis revealed distinct activation patterns associated with distraction. Using cortical activation maps, researchers found that distraction significantly increased oxygenated blood flow (∆HBO₂) in the dorsolateral prefrontal cortex (DLPFC) and premotor cortex. These areas are responsible for executive functions, attention regulation, and motor planning - key faculties in driving.
In contrast, undistracted driving showed relatively low activation in these regions, suggesting reduced cognitive load and more efficient attentional control. Moreover, the medial prefrontal cortex (mPFC) showed consistent involvement in all driving conditions, indicating its role in sustained attention and decision-making under cognitive demand.
The spatial mapping also identified specific fNIRS channels, especially channels 11 and 16, as the most responsive during distraction, capturing elevated haemodynamic activity. These findings are supported by earlier studies that link the mPFC to multitasking and self-monitoring in demanding environments like driving.
Notably, the combined haemodynamic signal (∆HBO₂HHB) yielded superior classification results when comparing baseline (resting) to distracted conditions, while ∆HBO₂ was more sensitive in differentiating subtle shifts during active driving. These results confirm that oxygen-based neurovascular signals are reliable indicators of attentional changes on the road.
Can this system improve road safety in real-world vehicles?
While the study was conducted in a simulator, it lays essential groundwork for real-time integration into vehicles. NeuroSafeDrive’s non-invasive, wearable fNIRS sensor system, coupled with compact machine learning classifiers, makes it feasible for use in driver-assistance technologies. The model can be trained to alert drivers or even engage autonomous intervention during high-risk distraction phases.
However, the researchers acknowledge that real-world implementation will require further development. The study’s sample size was limited to 21 participants, and the driving scenarios, though diverse, were simulated. The model’s robustness must be tested with larger datasets, different demographics, and varied real-world distractions.
Additionally, environmental noise, motion artifacts, and sensor placement variability can affect data fidelity. Future models may benefit from hybrid sensor systems, combining fNIRS with eye tracking, EEG, or vehicle telemetry to build a multi-modal monitoring system. The authors also recommend expanding secondary task categories to reflect everyday driving challenges more comprehensively.
- READ MORE ON:
- driver distraction detection
- AI road safety system
- AI driver attention system
- intelligent transportation systems
- real-time driver brain analysis
- how AI detects driver distraction using brain signals
- brain-based driver monitoring systems with fNIRS
- wearable brain-sensing technology for smart vehicles
- FIRST PUBLISHED IN:
- Devdiscourse