Machine learning can’t detect misinformation - Here's why
The core argument of the paper is that what counts as misinformation is not an objective fact but a relative judgment anchored in the epistemic and non-epistemic values of specific communities. These values evolve over time, creating what Yee identifies as “distribution shifts” in the data environments that machine learning models are trained on versus the real-world environments they are deployed in.
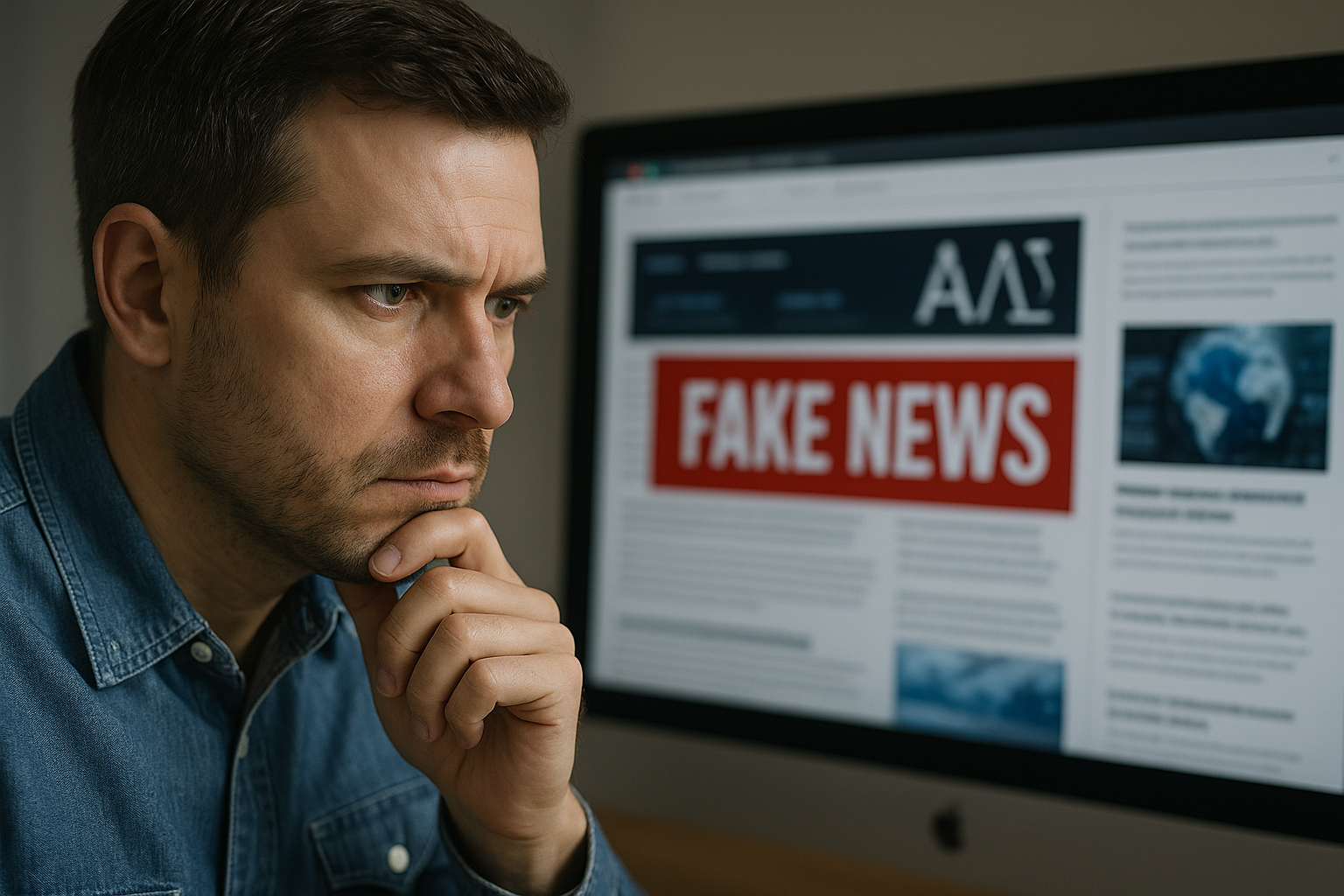
The effectiveness of machine learning models in identifying and curbing misinformation remains deeply contested. In a detailed and philosophically grounded critique, Adrian K. Yee of Lingnan University argues that machine learning models of misinformation (MMMs) are fundamentally ill-equipped to deliver on their promise. The peer-reviewed study, titled "The Limits of Machine Learning Models of Misinformation", published in the journal AI & Society, reveals deep methodological and ethical problems in how misinformation is defined, labeled, and operationalized in machine learning systems.
Despite growing interest in automated solutions to misinformation by governments and platforms alike, Yee's research contends that misinformation judgments are inherently value-laden, context-dependent, and subject to temporal shifts, making them unsuitable for fixed algorithmic classification. The study challenges prevailing assumptions and offers a reframing of MMMs not as truth-detection tools but as recommender systems reflecting communal values.
How are judgments of misinformation fundamentally unstable?
The core argument of the paper is that what counts as misinformation is not an objective fact but a relative judgment anchored in the epistemic and non-epistemic values of specific communities. These values evolve over time, creating what Yee identifies as “distribution shifts” in the data environments that machine learning models are trained on versus the real-world environments they are deployed in.
Yee illustrates this instability through historical and contemporary examples. For instance, state-driven propaganda in wartime Japan, public health misinformation in varying cultural contexts, and even online memes, all reveal the highly situational nature of misinformation. Whether a statement is deemed false or misleading often hinges not just on factual accuracy but on social values like political acceptability, perceived harm, and group identity.
These inconsistencies complicate the training of MMMs. Standard definitions, such as the alethic model that defines misinformation strictly as false information, prove too narrow and inflexible. They cannot account for legitimate disagreements (e.g., religious or political) or acceptable forms of misleading content (e.g., satire or rhetorical exaggeration). The study suggests that any attempt to automate misinformation detection without accounting for these contextual variables is methodologically flawed.
What makes machine learning models empirically inadequate?
Yee identifies five distinct types of distribution shifts that compromise the empirical adequacy of MMMs:
-
Covariate Shift A (CSA): Changes in input features (e.g., language style) while label distributions remain constant.
-
Covariate Shift B (CSB): Shifts in how features relate to labels, even if label distributions appear stable.
-
Label Shift (LS): The same input features receive different misinformation labels over time.
-
Concept Shift (CS): Definitions of misinformation evolve, even if input features are stable.
-
Radical Shift (RS): Simultaneous changes in input features, label distributions, and labeling functions.
Each shift undermines the assumptions of stationary data distributions foundational to machine learning. Yee provides empirical evidence from recent MMMs, including models applied to Twitter data, image classification using GANs, and cross-national news classifiers, to show how these shifts lead to decreased model performance and inconsistencies in label assignments.
In one example, classifiers trained on American media underperformed on UK datasets despite linguistic similarities, highlighting CSA. In another, supervised learning models assessed Russian state media as “reliable” by standard metrics, contradicting broad international assessments and exposing LS. These cases demonstrate how MMMs trained on one data distribution cannot generalize across different times, contexts, or communities.
The consequence is stark: no fixed training set or labeling strategy can capture the fluidity of real-world misinformation judgments. As a result, MMMs cannot be reliable detectors of falsehood but are instead reflections of the specific communities that define and label the training data.
Are there viable solutions to improve machine learning models of misinformation?
Yee examines three proposed strategies to address the problem of distribution shifts: larger static training sets, social engineering, and dynamic sampling. Each is rigorously evaluated for both feasibility and ethical implications.
1. Larger Static Datasets: While successful in other domains like language modeling, static datasets fail for MMMs due to the intrinsic volatility of misinformation judgments. No amount of data volume can compensate for changing informational norms. For example, the same statement about vaccine efficacy may be considered misinformation or not depending on evolving scientific consensus and public risk tolerance. Static datasets can only encode past judgments, not future shifts.
2. Social Engineering: This approach proposes manipulating the informational environment, through education, censorship, or nudging, to create more consistent standards. Yee warns against this strategy, citing ethical concerns about free speech, policymaker bias, and historical abuses. Examples like the Myanmar government’s use of Facebook to promote anti-Rohingya misinformation highlight the dangers of centralized control over information norms. Even well-intentioned nudges risk reinforcing the biases of elites, leading to informational epistocracies where only certain voices are legitimized.
3. Dynamic Sampling: Yee ultimately endorses dynamic sampling as the least problematic path forward. This method involves continually updating MMMs based on evolving stakeholder preferences, similar to how recommender systems function. By tracking user interactions and periodically surveying users about their judgments, MMMs can better reflect current informational values. However, this reframes MMMs not as truth detectors but as systems that mirror community-defined standards. The implications are significant: rather than identifying “fake news,” MMMs merely highlight what a specific community currently finds untrustworthy.
This reconceptualization aligns with findings from recent industry surveys indicating that existing MMMs are more adept at generating misinformation than detecting it. The study thus calls for a humbler, more socially aware approach to machine learning in the misinformation space, one that recognizes the subjective and evolving nature of truth judgments.
- READ MORE ON:
- machine learning misinformation
- AI misinformation detection
- AI and fake news
- why machine learning fails at detecting misinformation
- problems with automated fake news detection
- AI models and misinformation labeling
- misinformation and social media
- epistemic values in misinformation detection
- FIRST PUBLISHED IN:
- Devdiscourse