Machine learning becomes backbone of optical communications amid complexity challenges
In more unconventional domains like chaos-based secure optical communication and photonic reservoir computing, ML enables the decoding of highly complex signals and multi-channel synchronization. These are environments where classical models fail due to the nonlinear and chaotic nature of the signal sources. ML’s adaptability and pattern recognition capacity allow it to extract structure from what was previously noise.
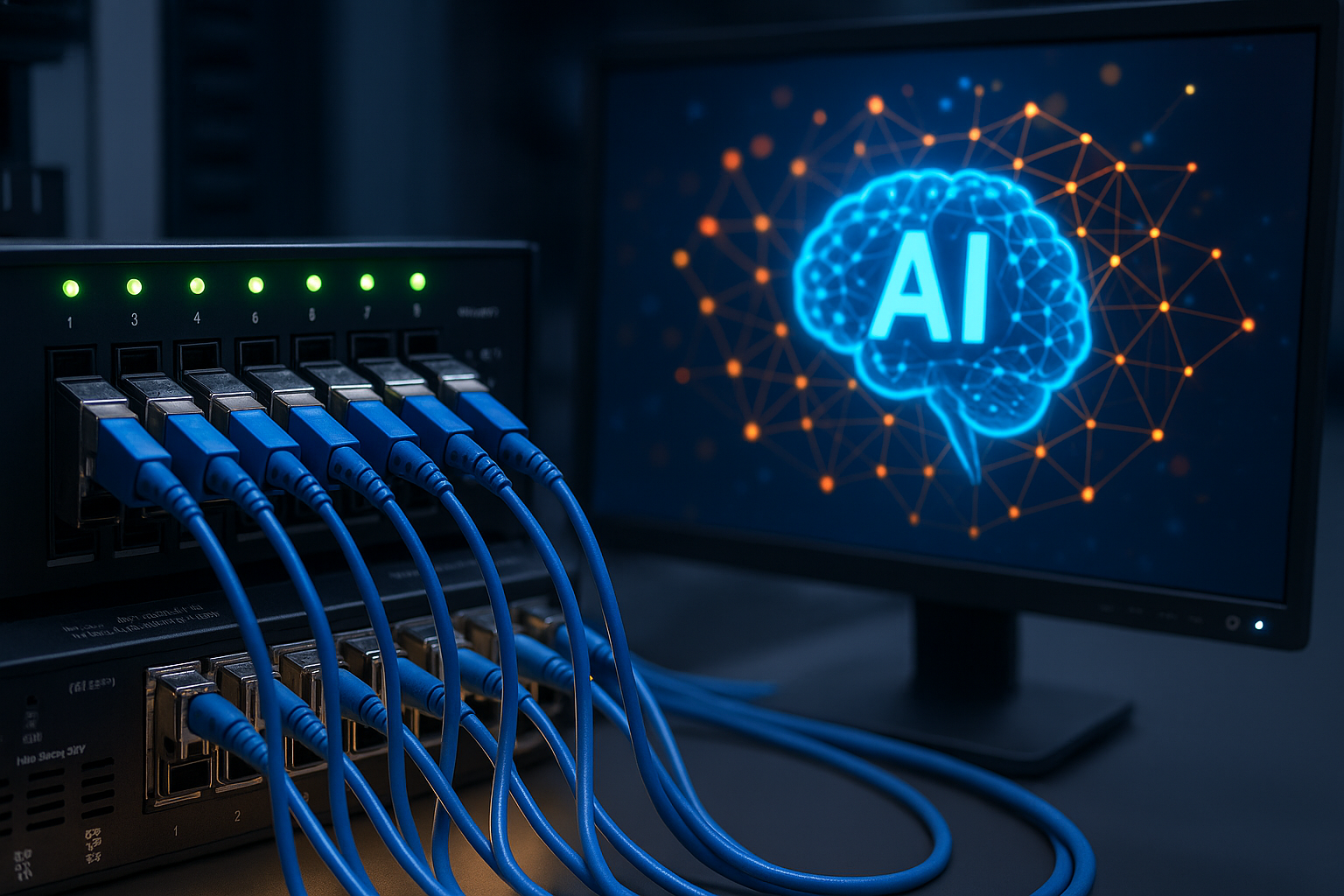
Optical communication systems are under mounting pressure to meet growing demands for speed, bandwidth, and reliability. Traditional rule-based design methodologies are proving insufficient to manage this complexity. A new editorial study, “Machine Learning Applied to Optical Communication Systems”, published in Photonics (2025), explores how machine learning (ML) is becoming indispensable in overcoming these challenges.
The study aggregates insights from 14 cutting-edge contributions, including three comprehensive reviews and eleven original research papers. It offers a wide-ranging analysis of how ML technologies, spanning neural networks, generative models, convolutional architectures, and probabilistic learning, are being deployed across every layer of optical communication, from long-haul fiber networks to visible light communication and optical wireless systems.
How is machine learning transforming traditional optical communication?
At the heart of the editorial is a recognition that ML is fundamentally altering how optical systems are designed, operated, and optimized. In long-haul coherent optical networks, used for transmitting data over hundreds of kilometers, ML is now employed to correct nonlinear impairments, polarization effects, and signal distortions. Neural networks, including deep learning models, have demonstrated superior performance over conventional Volterra filters and digital backpropagation methods, particularly in dynamic environments where traditional algorithms struggle to adapt.
Short-reach and direct-detection systems, common in data centers and consumer electronics, are also undergoing transformation. ML algorithms, including support vector machines and neural networks, have significantly improved equalization and bit error rates in scenarios involving low-cost hardware such as directly modulated lasers (DMLs), vertical-cavity surface-emitting lasers (VCSELs), and silicon photonics.
In more unconventional domains like chaos-based secure optical communication and photonic reservoir computing, ML enables the decoding of highly complex signals and multi-channel synchronization. These are environments where classical models fail due to the nonlinear and chaotic nature of the signal sources. ML’s adaptability and pattern recognition capacity allow it to extract structure from what was previously noise.
What are the key innovations presented in the special issue?
Each contribution in the Photonics special issue offers a targeted application of ML to a unique challenge within optical systems. A few standout examples include:
-
DeepChaos+ by Vu et al., a deep generative model for chaos signal detection in WDM systems, which improves bit error rate performance by three orders of magnitude.
-
Gradient-based Equalizers for VCSEL transceivers introduced by Srinivasan et al., enabling more robust performance under thermal drift, especially vital for automotive and data center networks.
-
End-to-End Learning Models like those developed by Luna-Rivera et al. for visible light communication (VLC) systems, which integrate autoencoders to enhance signal robustness in 5G and IoT deployments.
-
Photonic Neural Networks proposed by Hung et al., which utilize Mach–Zehnder interferometers for signal regeneration in silicon micro-ring modulator systems, demonstrating parity with digital counterparts at higher speeds.
-
ML-based Resilience Mechanisms in next-generation Ethernet passive optical networks (NG-EPONs), where AI supports fault detection and proactive recovery, key to the reliability of the tactile Internet.
Other contributions explore indoor positioning through attention-based CNNs, high-speed optical wireless communication via neural equalizers, and secure communication through photonic reservoir computing backed by quantum dot spin VCSELs.
The review articles provide essential context, comparing network architectures, summarizing time-series forecasting strategies, and assessing computational trade-offs. One particularly important review addresses the challenges of integrating ML into self-coherent optical systems, highlighting improvements in signal recovery and cost-effective detection.
What are the broader implications and future directions?
The collected works illustrate that ML is no longer a peripheral tool in optical communication - it is central to enabling the next generation of network capabilities. From enhancing physical-layer performance to enabling autonomous network management, ML allows systems to move beyond deterministic rules into adaptive, self-optimizing behavior.
Notably, ML is playing a growing role in network orchestration, performance monitoring, and predictive maintenance. Techniques like long short-term memory (LSTM) networks and convolutional neural networks are being used to forecast optical signal-to-noise ratios (OSNR), detect faults, and identify optimal modulation formats on-the-fly. This capability aligns with broader trends toward software-defined networking and real-time system reconfiguration.
The study also sheds light on some key challenges including the high computational cost of training and deploying ML models, especially in low-power environments; the need for real-time adaptability without sacrificing accuracy; and the integration of ML models into existing hardware and software infrastructure. The risk of overfitting and the interpretability of models, especially those making high-impact decisions in critical infrastructure, also remain open areas for exploration.
- READ MORE ON:
- machine learning in optical communication
- AI in optical networks
- intelligent optical systems
- next-generation optical communication
- machine learning optical systems
- ML-enabled optical infrastructure
- real-time machine learning in optical systems
- autonomous optical network management
- AI for 5G optical backhaul systems
- FIRST PUBLISHED IN:
- Devdiscourse