AI and wireless infrastructure lead the next phase of digital transformation in education
For decades, educational institutions have relied on siloed administrative systems plagued by outdated timetables, classroom overbooking, and reactive maintenance strategies. The framework introduced in this study tackles these inefficiencies by fusing data from heterogeneous sensors with AI-driven analytics that process the information in real-time. Modules such as predictive maintenance, asset tracking, and adaptive scheduling work together to enable proactive management across campuses.
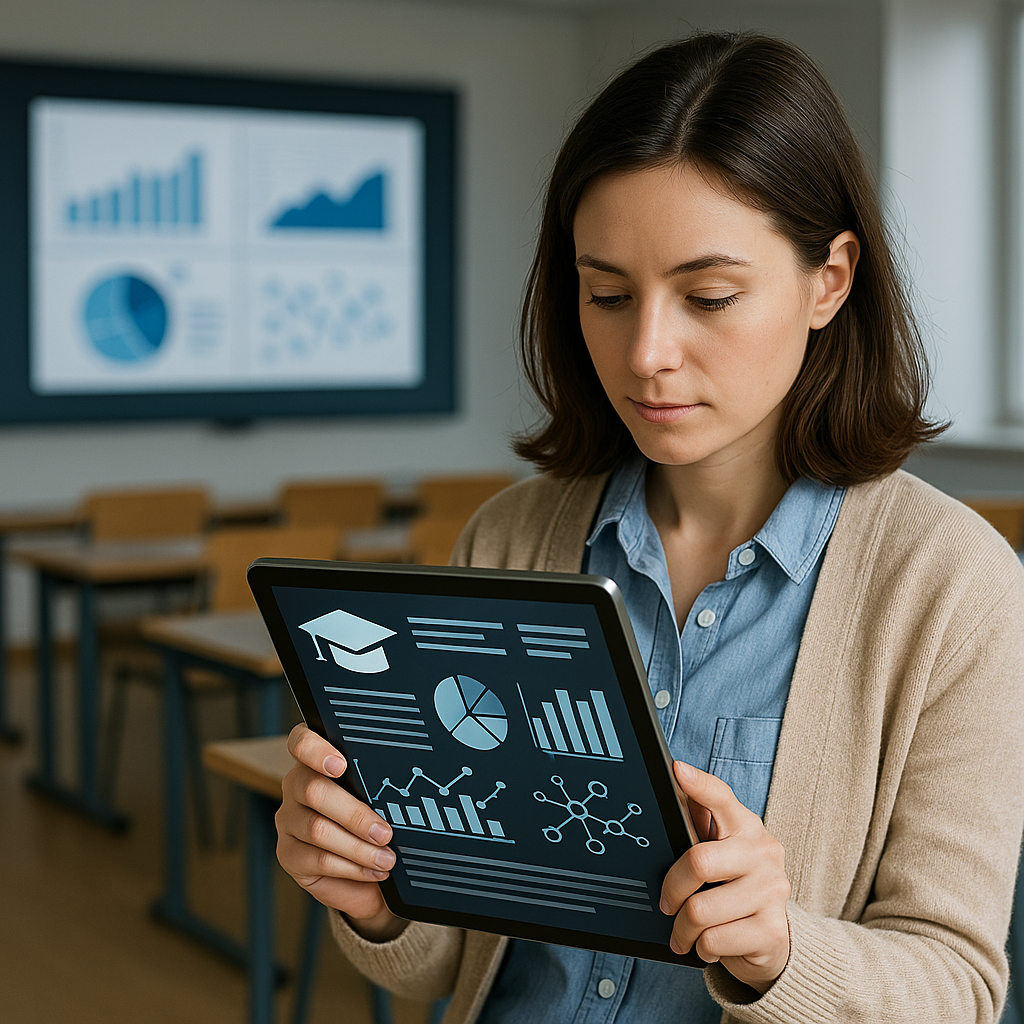
A new conceptual framework is redefining how educational institutions manage resources and make decisions, as the convergence of artificial intelligence (AI), 5G wireless networks, and the Internet of Things (IoT) takes shape across global campuses. Published in Applied Sciences under the title “Revolutionising Educational Management with AI and Wireless Networks: A Framework for Smart Resource Allocation and Decision-Making”, the study presents a modular architecture that promises to modernize timetabling, predictive maintenance, energy optimization, and data security on campuses through intelligent automation and seamless interconnectivity.
Developed through design-science methodology, the framework enables dynamic resource scheduling, real-time infrastructure monitoring, and AI-enhanced decision support, all within a privacy-compliant, secure, and scalable ecosystem. It also addresses critical implementation gaps such as the lack of cross-layered integration, technical inaccessibility for administrators, and fragmented data governance. By deploying sensors, edge computing, and AI models across layered system components, the framework provides a roadmap for educational institutions to evolve into fully connected “smart campuses.”
How does the framework solve long-standing operational inefficiencies in education?
For decades, educational institutions have relied on siloed administrative systems plagued by outdated timetables, classroom overbooking, and reactive maintenance strategies. The framework introduced in this study tackles these inefficiencies by fusing data from heterogeneous sensors with AI-driven analytics that process the information in real-time. Modules such as predictive maintenance, asset tracking, and adaptive scheduling work together to enable proactive management across campuses.
For example, HVAC systems, projectors, and servers are continuously monitored by IoT devices that detect anomalies such as overheating or vibration spikes. AI algorithms, including LSTM and isolation forests, process this data to predict failures before they disrupt operations. When a failure is imminent, the system auto-generates a work order, reschedules affected classes, and optimizes technician dispatch timing, integrating physical space and human availability constraints.
Likewise, the scheduling module employs meta-heuristic algorithms like tabu search and hill climbing to construct and continuously refine conflict-free timetables. The system autonomously resolves disruptions, such as instructor illness or room unavailability, by reassigning classes with minimal timetable perturbation. Notifications are dispatched instantly via integrated mobile platforms, reducing administrative burden and enhancing agility.
The resource allocation engine ensures optimal utility usage by adjusting lighting, HVAC, and digital resource provisioning based on real-time occupancy and system demand. For example, empty classrooms have their power consumption reduced, while crowded labs receive additional bandwidth or energy allocation to maintain comfort and functionality.
What makes this framework secure, scalable, and inclusive?
Security and scalability are foundational pillars of the proposed smart campus architecture. Recognizing the growing cybersecurity risks posed by interconnected IoT devices, the framework incorporates multilayered defense strategies, including end-to-end encryption, device authentication, AI-driven intrusion detection systems (IDS), and secure federated learning. These protections ensure data integrity and compliance with global standards like GDPR and FERPA.
The IDS system detects anomalies such as unusual data spikes or unauthorized access attempts, and autonomously triggers responses such as node quarantining. Role-based access control governs data visibility, ensuring that only authorized personnel can view or act upon sensitive information. Blockchain technology is proposed as a future enhancement for immutable record-keeping of transactions like maintenance logs and resource reallocations.
The framework’s modular design supports incremental implementation. Institutions with limited budgets or technical maturity can adopt specific components, such as the scheduling engine or maintenance dashboard, without a complete system overhaul. As readiness improves, additional modules, such as dynamic energy management or sentiment-based scheduling from social media inputs, can be layered in. Real-world case studies, such as those from James Madison University and Politecnico di Torino, have demonstrated the feasibility and impact of such technologies.
Furthermore, the framework emphasizes accessibility. Its explainable dashboards and user-friendly interfaces are tailored for non-specialist administrators, avoiding the need for deep IT expertise. Visual aids and interactive AI outputs empower human decision-makers to adjust recommendations, ensuring the human-in-the-loop principle remains intact.
What are the long-term implications for educational institutions?
The study posits that the long-term benefits of this smart campus architecture extend well beyond efficiency. By transforming manual operations into anticipatory, data-informed processes, educational institutions can achieve greater resilience, sustainability, and responsiveness. Predictive maintenance reduces operational downtime and costs. Adaptive scheduling improves student experience by reducing class conflicts and supporting learning continuity during disruptions. Intelligent energy management reduces carbon footprints, aligning campuses with global climate targets.
Strategically, the system supports “what-if” scenario testing using digital twins. Institutions can simulate policy changes or infrastructure upgrades in virtual environments, assessing impact before physical implementation. This capability aligns with global trends in smart governance, risk mitigation, and inclusive planning.
Challenges including ensuring consistent data quality, overcoming resistance to algorithmic decision-making, and navigating the high initial investment required for full-scale rollout, remain. However, the study’s roadmap anticipates these obstacles by offering modular entry points, open APIs for system integration, and federated learning for privacy-preserving analytics.
Future research could focus on integrating extended reality (XR), quantum-safe cryptography, and multi-model load forecasting into the framework, the authors suggest.
- FIRST PUBLISHED IN:
- Devdiscourse