Trust or ignore? New tools quantify user confidence in artificial intelligence
The study identifies a major gap in the AI-human interaction research landscape: while dozens of trust measures exist, few have undergone rigorous validation across diverse AI contexts. The researchers addressed this by putting the 12-item TIAS, a widely cited yet under-validated scale, through comprehensive psychometric testing.
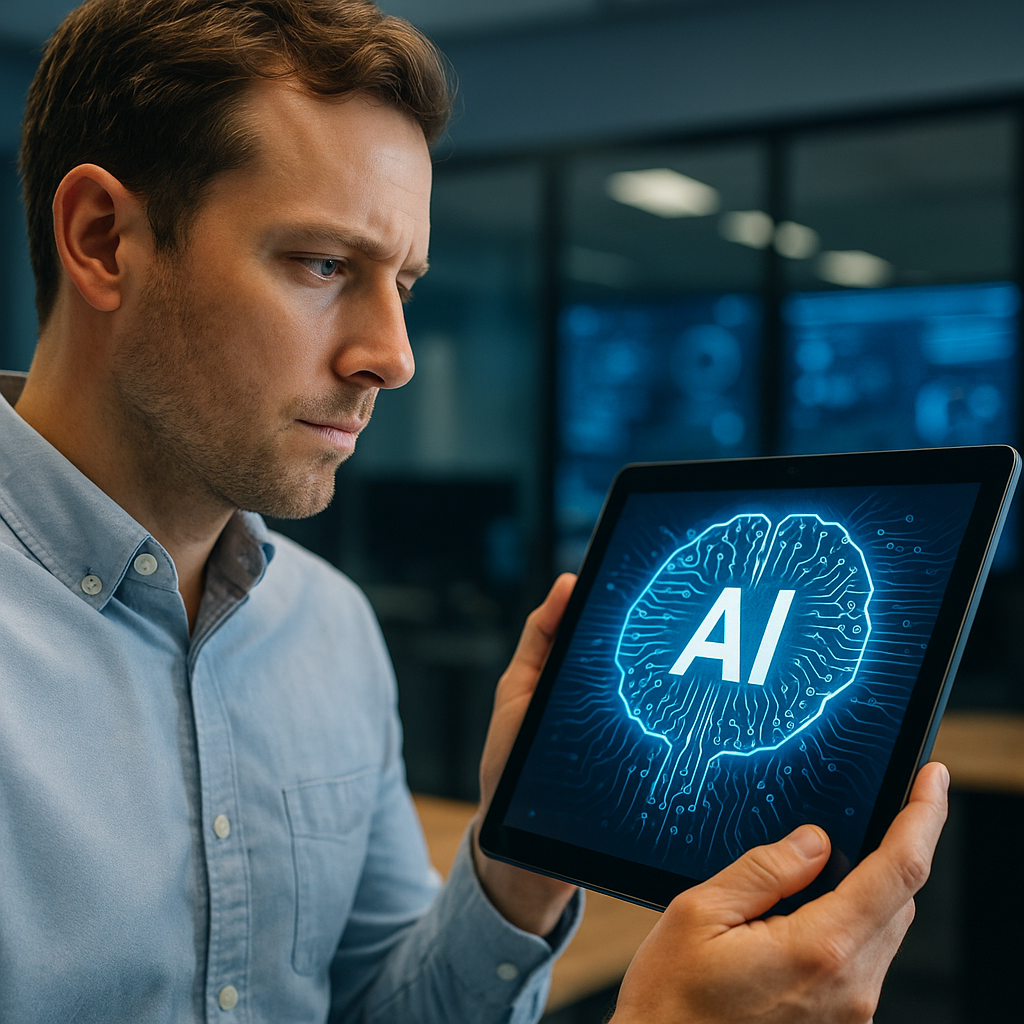
With artificial intelligence (AI) systems increasingly making high-stakes decisions in healthcare, mobility, and finance, accurately measuring human trust in these systems has become critical. A newly published study titled “Measuring Trust in Artificial Intelligence: Validation of an Established Scale and Its Short Form” addresses this growing need by validating both the full and a newly developed short form of the Trust in Automation Scale (TIAS). The study establishes these tools as essential instruments for capturing how much people trust the AI systems they interact with, and why that trust matters for real-world adoption.
Conducted by researchers at CSIRO, the University of Adelaide, and the University of Melbourne, the study tested the TIAS and its shortened version, the Short Trust in Automation Scale (S-TIAS), across multiple AI scenarios. The researchers assessed the scales' sensitivity to system performance and integrity, their predictive power regarding user behavior, and the correlation with individual predispositions to trust humans or machines.
How was trust in AI measured and why does it matter?
The study identifies a major gap in the AI-human interaction research landscape: while dozens of trust measures exist, few have undergone rigorous validation across diverse AI contexts. The researchers addressed this by putting the 12-item TIAS, a widely cited yet under-validated scale, through comprehensive psychometric testing.
Participants were shown real-life vignettes involving different AI applications, including self-driving cars, medical diagnostic tools, virtual assistants, and flight booking agents. These systems were presented in either high-trustworthiness or low-trustworthiness variants, manipulating either performance quality or integrity (e.g., bias in recommendations).
In each case, trust was measured using the TIAS and compared against behavioral intent (BI), whether participants would follow AI advice, as well as trust propensities toward both humans (HTP) and machines (MTP). The researchers found that trust scores significantly increased when participants were exposed to more capable or more ethical AI systems. Notably, the TIAS also predicted whether participants were likely to rely on AI-generated recommendations, regardless of their general attitudes toward machines or people.
What is the short trust in automation scale and why is it useful?
While the TIAS proved effective, the researchers acknowledged that its 12-item length is impractical for studies needing repeated, real-time trust assessments. This led to the development of the S-TIAS, a condensed three-item version derived from the best-performing items in the original scale: confidence in the system, reliability, and overall trustworthiness.
Across two additional studies, the S-TIAS demonstrated similar sensitivity and predictive power as the full version. It was particularly effective in distinguishing between high and low trust conditions, both in terms of AI performance and ethical integrity. Importantly, trust scores from the S-TIAS explained over 70% of the variance in behavioral intent, proving its value as a quick, reliable alternative for trust measurement.
One key insight from the analysis was that machine trust propensity (MTP) consistently correlated with both scales, while human trust propensity (HTP) showed no significant relationship. This suggests that trust in AI is more strongly related to how individuals perceive technology in general, rather than how they trust people. The finding challenges previous assumptions that generalized interpersonal trust translates to machine trust and underscores the need to treat trust in AI as a distinct psychological construct.
What are the broader implications for AI design, deployment, and research?
The validated scales have direct implications for both the development and governance of AI systems. For developers, the TIAS and S-TIAS offer robust tools for evaluating how users perceive trustworthiness across system updates, transparency changes, or deployment environments. In sectors such as healthcare, defense, and finance, where AI decisions can carry life-altering consequences, calibrating trust accurately is essential to prevent either over-reliance or under-utilization.
For researchers, these scales open new pathways to investigate trust trajectories, repair after failure, and longitudinal dynamics of AI trust. The S-TIAS, in particular, is expected to facilitate episodic measurements during human-AI collaboration studies and operational deployments, where minimal interruption is key. The authors note that future studies should explore how these validated scales perform in live behavioral settings beyond self-reported intent, potentially integrating physiological or behavioral trust indicators.
The study also sheds light on theoretical trust models. It reinforces the view that trust in AI is not monolithic but multidimensional, anchored in factors like reliability, fairness, and transparency. Importantly, the authors found that performance-based trust cues (e.g., whether the AI worked well) were more influential in shaping user trust than integrity cues (e.g., fairness or bias). This finding may help explain why technically competent AI tools are often trusted despite controversies around ethics or data use.
The researchers suggest that the Perfect Automation Schema (PAS) - a cognitive model where users assume automation is either perfect or untrustworthy - might further moderate trust dynamics. Future research may investigate how expectations formed by past experiences or media narratives influence real-time trust calibration.
- READ MORE ON:
- trust in artificial intelligence
- trust in AI scale
- measuring trust in automation
- human-AI interaction trust
- trust in automation assessment
- how to measure trust in artificial intelligence
- trust indicators in human-AI collaboration
- real-time trust monitoring in AI
- explainable AI and trust measurement
- FIRST PUBLISHED IN:
- Devdiscourse