GenAI agents show human-like forecasting in market simulations but lack behavioral diversity
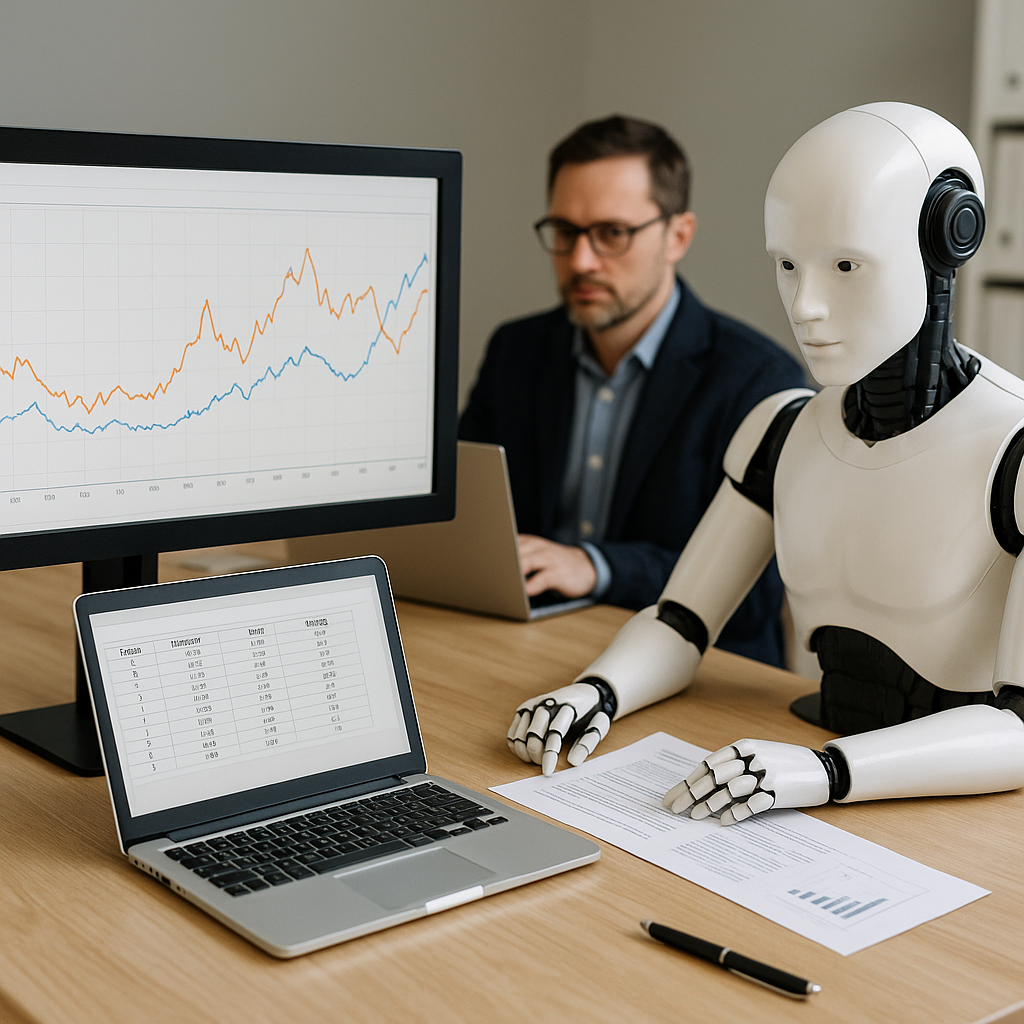
In a study titled "Can Generative AI Agents Behave Like Humans? Evidence from Laboratory Market Experiments" published, researchers R. Maria del Rio-Chanona, Marco Pangallo, and Cars Hommes investigate whether large language models (LLMs) like GPT-3.5 and GPT-4 can realistically replicate human behavior in controlled economic experiments. The study leverages established economic frameworks, particularly the experimental designs of Heemeijer et al. (2009), to assess how AI agents behave in dynamic market environments characterized by feedback loops between predictions and prices.
The researchers simulate laboratory-style economic experiments using LLMs rather than human participants. Each virtual agent must predict future market prices based on prior outcomes and its own historical performance. Two key market types are tested: positive feedback markets, which often create speculative bubbles, and negative feedback markets, which typically stabilize around fundamental values. To compare outcomes, the researchers meticulously replicate the conditions of human experiments, using the same mathematical structures and prediction-based earnings incentives, and analyze the alignment between LLM and human behavior using trend-following regression models.
The team introduces three critical experimental controls: memory (how many past steps agents recall), temperature (randomness in predictions), and model type (GPT-3.5 or GPT-4). Memory lengths of 1, 3, and 5 steps, combined with temperatures of 0.3, 0.7, and 1.0, are tested across both feedback markets to identify optimal conditions for human-like behavior.
How well do AI agents simulate human market behavior?
The study finds that under certain conditions, LLM agents can replicate several broad patterns observed in human market experiments. Most notably, GPT-based agents mirror human trends in both positive and negative feedback markets, especially when granted a memory of at least three steps and high temperature settings (i.e., increased variability in responses).
In positive feedback markets, both human subjects and LLMs often exhibit volatile, trend-amplifying behavior. Human agents typically show persistent fluctuations or slow convergence to equilibrium. GPT-3.5 mimics this behavior, showing extended cycles of price oscillations, sometimes resembling market bubbles. GPT-4, by contrast, converges more steadily and aligns more closely with one of the observed human group patterns.
In negative feedback markets, where expectations dampen excessive price movements, human subjects stabilize within roughly 10 steps. GPT-4 shows similar convergence timelines, but GPT-3.5 is more erratic, requiring over 20 steps and demonstrating less consistent correction.
The researchers also uncover a critical dependency on memory length. With only a 1-step memory, even GPT-4 fails to achieve human-like convergence. Increasing memory to 3 or 5 steps dramatically improves alignment. Temperature has a secondary effect: higher randomness increases behavioral diversity, aiding in realism without sacrificing coherence.
Do LLMs think like humans? A deep dive into strategy and reasoning
To quantify behavioral similarities, the researchers estimate each agent’s forecasting strategy using a first-order heuristic model that captures reliance on recent prices, past predictions, and price trends. Human participants are shown to rely on diverse strategies, ranging from naïve trend-followers to adaptive learners and fundamentalists (those anchoring forecasts to a fixed “true” price of 60). This heterogeneity is far greater in humans than in LLMs.
GPT-3.5 and GPT-4, in contrast, consistently behave as trend followers in positive feedback markets and show little to no trend-following in negative feedback environments - a key alignment with human behavior. However, they lack the rich strategic diversity observed in human participants. For instance, LLMs tend to cluster narrowly around naïve and adaptive strategies, seldom venturing into outlier forecasting behaviors. GPT-4 performs better than GPT-3.5 in replicating nuanced strategy shifts across different market types.
The study goes a step further by analyzing the narratives and rationale generated by GPT-3.5 agents during market simulations. These qualitative texts reveal that LLMs not only follow price trends numerically but also articulate trend-following logic in their reasoning. As markets approach a turning point (e.g., the peak of a speculative bubble), agent narratives remain optimistic - often lagging behind actual price reversals. This lag suggests that while LLMs articulate strategy well, their reasoning may still be susceptible to noise, especially in high-temperature settings.
One notable limitation is the reduced behavioral variability in LLMs compared to humans. Despite fine-tuning prompts and environmental memory, LLMs struggle to spontaneously generate the broad range of heuristics and idiosyncratic forecasting patterns characteristic of real-world agents.
Implications for economics and future research directions
This study marks a significant advance in the simulation of economic behavior using artificial agents. It confirms that, with careful design and parameter tuning, LLMs can replicate core market dynamics traditionally observed in costly human-based laboratory experiments. Importantly, these AI agents exhibit bounded rationality, a cornerstone concept in behavioral economics, rather than strict adherence to idealized rational expectations.
The authors suggest that such simulations could soon form the backbone of general-purpose bounded rationality models in economics, offering scalable alternatives to traditional agent-based modeling. This aligns with ongoing efforts to make economic theory more realistic and empirically grounded, especially in light of recent advances in computational social science.
However, critical challenges remain. LLMs still exhibit limited heterogeneity and may carry biases reflecting training data, potentially skewing their mimicry of human behavior. The authors advocate for future work incorporating demographic, psychological, or ideological variability into LLM agents. Additional research could also explore more complex market structures, bubble dynamics, and behavioral transitions to further test AI realism.
- FIRST PUBLISHED IN:
- Devdiscourse