AI takes lead in farm finance: Machine learning beats legacy models in bankruptcy prediction
While both models showed remarkable prediction accuracy, decision trees offered an added advantage: interpretability. Decision trees allow for clear threshold identification, rule-based decision paths, and easier communication of financial risks to non-technical stakeholders—an area where ANN models fall short due to their “black-box” nature.
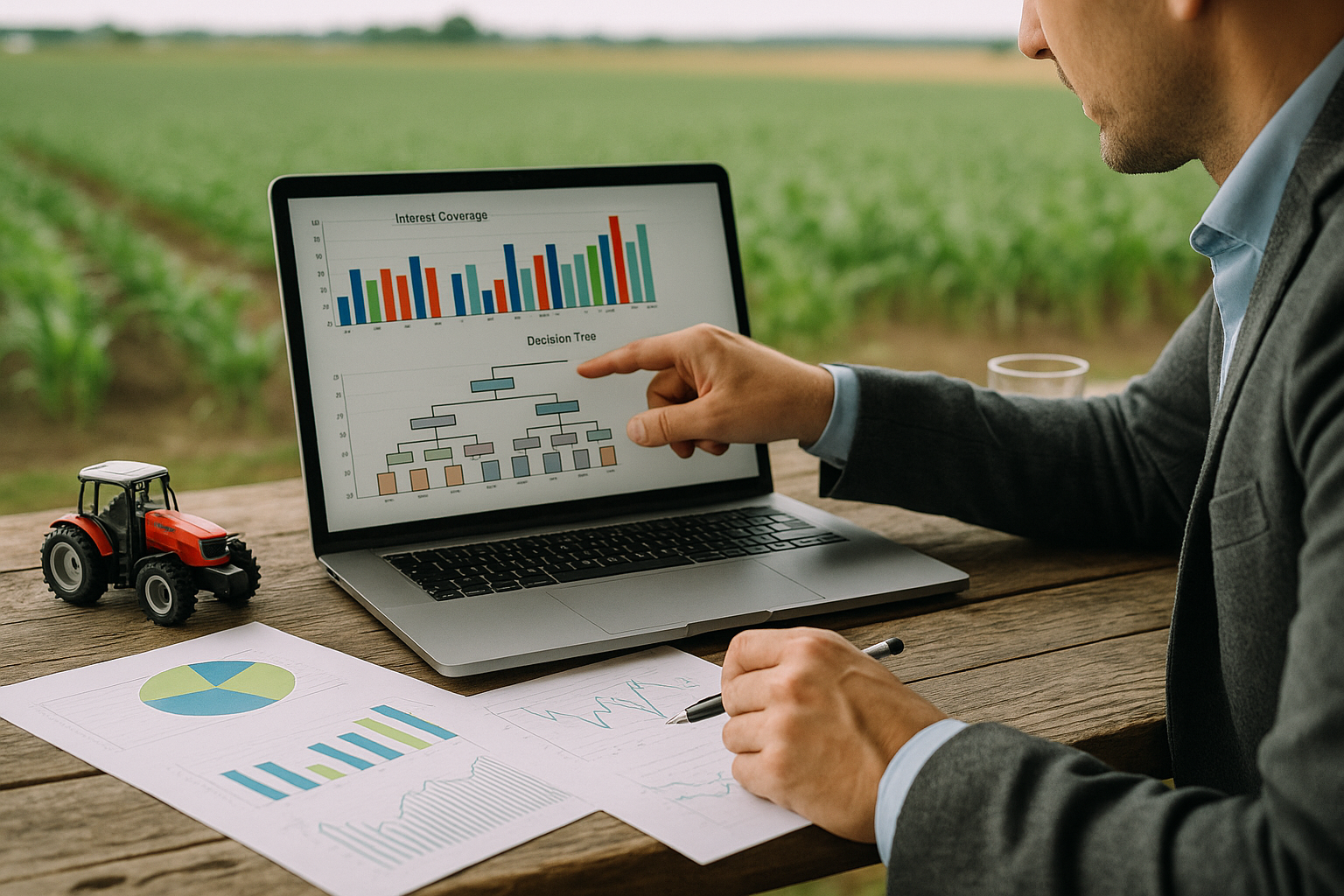
A groundbreaking comparative study has revealed that artificial intelligence (AI) models, specifically artificial neural networks (ANN) and decision trees (DT), significantly outperform traditional statistical methods in predicting bankruptcy among agricultural firms. The study, titled “Artificial Intelligence Models for Bankruptcy Prediction in Agriculture: Comparing the Performance of Artificial Neural Networks and Decision Trees,” was published in the journal Agriculture.
The research is based on financial data from 1,123 Slovak agricultural enterprises, aiming to identify key indicators of financial distress and evaluate which machine learning model provides the most accurate, reliable, and interpretable predictions. The findings are expected to aid financial analysts, agricultural policymakers, and rural development stakeholders in designing effective early warning systems tailored to the unique challenges of the agriculture sector.
Can AI models like ANN and DT accurately predict financial distress in agriculture?
The researchers developed predictive models using both ANN and DT algorithms and validated them through a rigorous evaluation process involving accuracy, precision, recall, F1-score, and area under the receiver operating characteristic curve (AUC). The ANN model achieved an AUC of 0.9500, a classification accuracy of 96.37%, precision of 96.60%, recall of 99.68%, and an F1-score of 98.12%. The DT model slightly outperformed the ANN, registering an AUC of 0.9550, accuracy of 97.78%, precision of 98.69%, recall of 99.01%, and an F1-score of 98.85%.
While both models showed remarkable prediction accuracy, decision trees offered an added advantage: interpretability. Decision trees allow for clear threshold identification, rule-based decision paths, and easier communication of financial risks to non-technical stakeholders—an area where ANN models fall short due to their “black-box” nature.
The research also demonstrated the shortcomings of traditional statistical models like discriminant analysis and logistic regression, which rely on assumptions of linearity and homoscedasticity that do not hold in the volatile and complex financial environment of the agricultural sector. AI models, by contrast, handle non-linearities and noisy data more effectively, making them better suited for real-world financial forecasting in agriculture.
What are the key financial indicators that drive bankruptcy risk in agricultural firms?
The study identified specific financial indicators that significantly influence a firm's likelihood of bankruptcy. The most influential variables included the current indebtedness ratio, total indebtedness ratio, financial independence ratio, and self-financing ratio.
In the ANN model, the current indebtedness ratio was the most critical, with normalized importance at 100%. This was followed by the financial independence ratio (90.7%) and the total indebtedness ratio (89.1%). These indicators reflect a firm's reliance on short-term liabilities, its ability to fund operations through equity, and overall financial stability.
The DT model similarly emphasized total indebtedness, financial independence, and self-financing ratios, each receiving the highest importance scores. The debt-to-equity and equity leverage ratios were also weighted significantly, underscoring the model’s sensitivity to leverage and liquidity metrics.
Some variables, such as the interest burden ratio and debt-to-cash flow ratio, while not statistically significant in isolation, were retained in the models due to their theoretical relevance in assessing solvency. Their inclusion enhanced the multidimensional assessment of financial health and mitigated risks of omitting critical contextual indicators.
How can these AI models be applied to improve financial stability in agriculture?
The study’s findings offer substantial implications for the application of AI in rural economic policy and farm management. The authors argue that ANN and DT models can serve as effective early warning systems, allowing stakeholders to identify at-risk firms before insolvency becomes irreversible.
For policymakers, these tools provide empirical support to inform subsidy allocations, credit risk assessments, and targeted financial interventions. Decision trees, in particular, can be incorporated into financial monitoring dashboards to provide real-time, interpretable updates on enterprise stability.
Agricultural managers and financial analysts can integrate these AI models into internal systems to monitor key financial ratios such as debt-to-equity and interest coverage. These tools enable proactive restructuring decisions, investment planning, and engagement with creditors and insurers.
In addition, the study calls attention to the potential for expanding AI-based bankruptcy prediction frameworks by incorporating macroeconomic data, environmental risk indicators, and qualitative governance metrics. Advanced techniques like ensemble models and explainable AI (e.g., SHAP or LIME) were recommended for future research to further enhance predictive power and transparency.
- FIRST PUBLISHED IN:
- Devdiscourse