AI-driven climate models enable smarter, faster urban adaptation planning
AI models extract high-level features from diverse sources - satellites, ground sensors, historical climate records, and even emerging streams like IoT networks and citizen science. These data streams improve responsiveness and enable localized assessments, which are critical for disaster preparedness and infrastructure planning.
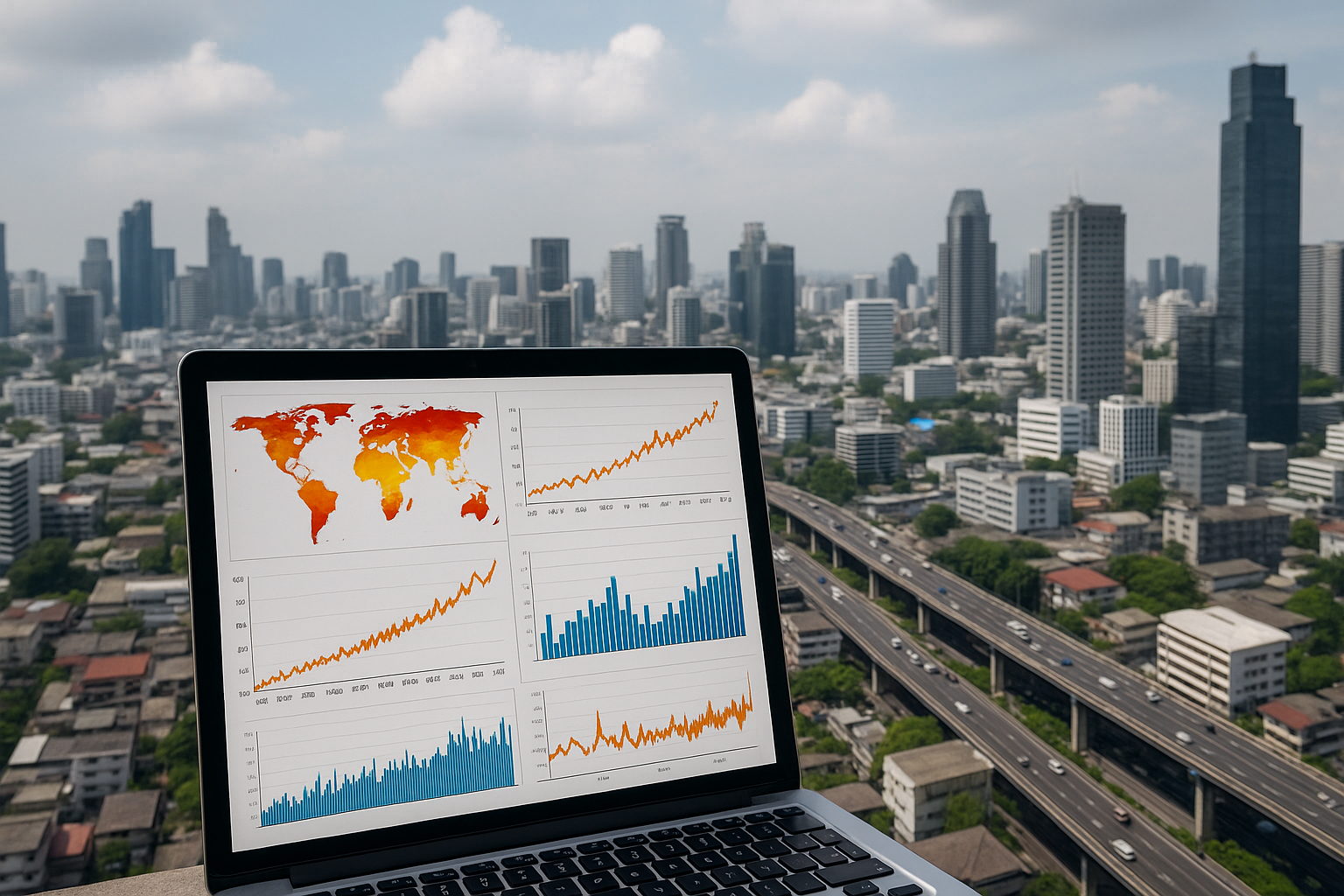
In a rapidly urbanizing world increasingly vulnerable to climate change, artificial intelligence (AI) is transforming how cities plan for climate resilience. A new mini review published in Frontiers in Artificial Intelligence, titled “Advancements and Challenges of Artificial Intelligence in Climate Modeling for Sustainable Urban Planning”, explores how machine learning (ML) and deep learning (DL) techniques are enhancing predictive accuracy, risk assessment, and policy development in urban environments.
Authored by Teerachai Amnuaylojaroen of the University of Phayao, the study draws on over 150 rigorously selected peer-reviewed publications from 2016 to 2025 to examine the capabilities and limitations of AI-based climate models. It provides a global perspective on how AI is deployed in different regions and proposes future directions to address technical, interpretability, and ethical challenges in climate-adaptive urban planning.
How is AI reshaping climate modeling for urban resilience?
The study outlines a three-phase AI-driven climate modeling pipeline: Data and Model Development, Analysis and Prediction, and Policy Action and Implementation. This framework supports sustainable urban planning by providing real-time forecasts, improved spatial resolution, and actionable insights on climate risks such as extreme heat, floods, and air pollution.
AI models extract high-level features from diverse sources - satellites, ground sensors, historical climate records, and even emerging streams like IoT networks and citizen science. These data streams improve responsiveness and enable localized assessments, which are critical for disaster preparedness and infrastructure planning.
For instance, the review notes that Gradient Boosting Machines and Decision Trees have been particularly effective in Southeast Asia for refining temperature and air quality projections in data-scarce contexts, while more complex hybrid models such as LSTM-CNN networks are deployed in data-rich settings like Europe to enhance wind energy forecasts.
AI’s predictive strength lies in its ability to model nonlinear relationships and process large, high-dimensional datasets. These capabilities enable it to outperform traditional climate models in both accuracy and speed. However, the scalability of such models across regions with inconsistent data quality remains a central obstacle.
What are the main technical and ethical challenges?
Despite their promise, AI-driven climate models face persistent data quality and interpretability barriers. The study emphasizes that inconsistencies in spatial and temporal coverage, particularly in developing regions, hinder the reliability and transferability of these models. To address this, data preprocessing methods like outlier detection, Z-score normalization, and imputation techniques are used to fill gaps and reduce noise.
The issue of interpretability, often referred to as the “black box” problem, is another major barrier to AI adoption in policymaking. Ensemble methods like Random Forests and deep networks such as CNNs offer high performance but obscure how predictions are made. To improve transparency, the study highlights the use of explainability tools such as SHAP (Shapley Additive Explanations), LIME (Local Interpretable Model-Agnostic Explanations), and permutation importance. These tools allow planners and stakeholders to understand which variables drive specific predictions.
Further, the paper raises ethical concerns related to fairness, transparency, and accountability. AI models trained on biased datasets may misrepresent climate risks in underrepresented areas, leading to unequal resource distribution. For example, poor predictions in vulnerable regions like sub-Saharan Africa or Southeast Asia could result in inadequate adaptation measures.
Compliance with data protection regulations like GDPR and the need for documented model limitations are also essential to responsible deployment. The study advocates for routine bias audits, diverse training datasets, and governance frameworks that ensure equitable outcomes from AI-driven urban policies.
What are the global applications and future research priorities?
The study presents six illustrative case studies from around the world that demonstrate the versatility of AI in climate adaptation:
- Europe: LSTM-CNN hybrid models improved wind energy prediction by 20%.
- India: U-Net autoencoders accurately forecast PM2.5 air pollution levels in dense urban zones.
- Southeast Asia: Gradient Boosting and Decision Trees have been useful for temperature projections in regions with limited observational data.
- East Africa: SVMs are employed for drought prediction, aiding food security interventions.
- South America: Transfer learning with ResNet CNNs supports near real-time deforestation monitoring in the Amazon.
These examples reveal how model selection depends heavily on data availability and infrastructure readiness. Simpler, interpretable ML models perform well in data-scarce regions, while deep learning architectures are suited for dense, high-quality datasets.
Looking forward, the review recommends integrating reinforcement learning for real-time infrastructure adaptation, federated learning for decentralized AI with privacy protections, and multi-modal systems combining satellite, sensor, and socio-economic data for more holistic forecasts. Quantum computing is also highlighted as a potential game-changer for simulating complex climate interactions, particularly in extreme weather scenarios.
- READ MORE ON:
- AI in climate modeling
- sustainable urban planning
- climate resilience
- machine learning climate predictions
- deep learning for urban planning
- artificial intelligence and sustainability
- AI-driven disaster mitigation
- how AI improves climate modeling accuracy
- machine learning for sustainable city planning
- ethical challenges in AI climate forecasting
- real-time climate predictions using deep learning
- AI tools for policy-making in climate-vulnerable cities
- FIRST PUBLISHED IN:
- Devdiscourse