How machine learning is revolutionizing real-time ocean monitoring
Ocean color remote sensing (OCRS) provides crucial insights into marine ecosystems, detecting phytoplankton blooms, measuring chlorophyll-a concentrations, monitoring carbon cycles, and assessing ocean health. However, traditional models have struggled to process the nonlinear and high-dimensional relationships within ocean reflectance data, particularly in coastal waters where atmospheric interference and optical complexity are highest.
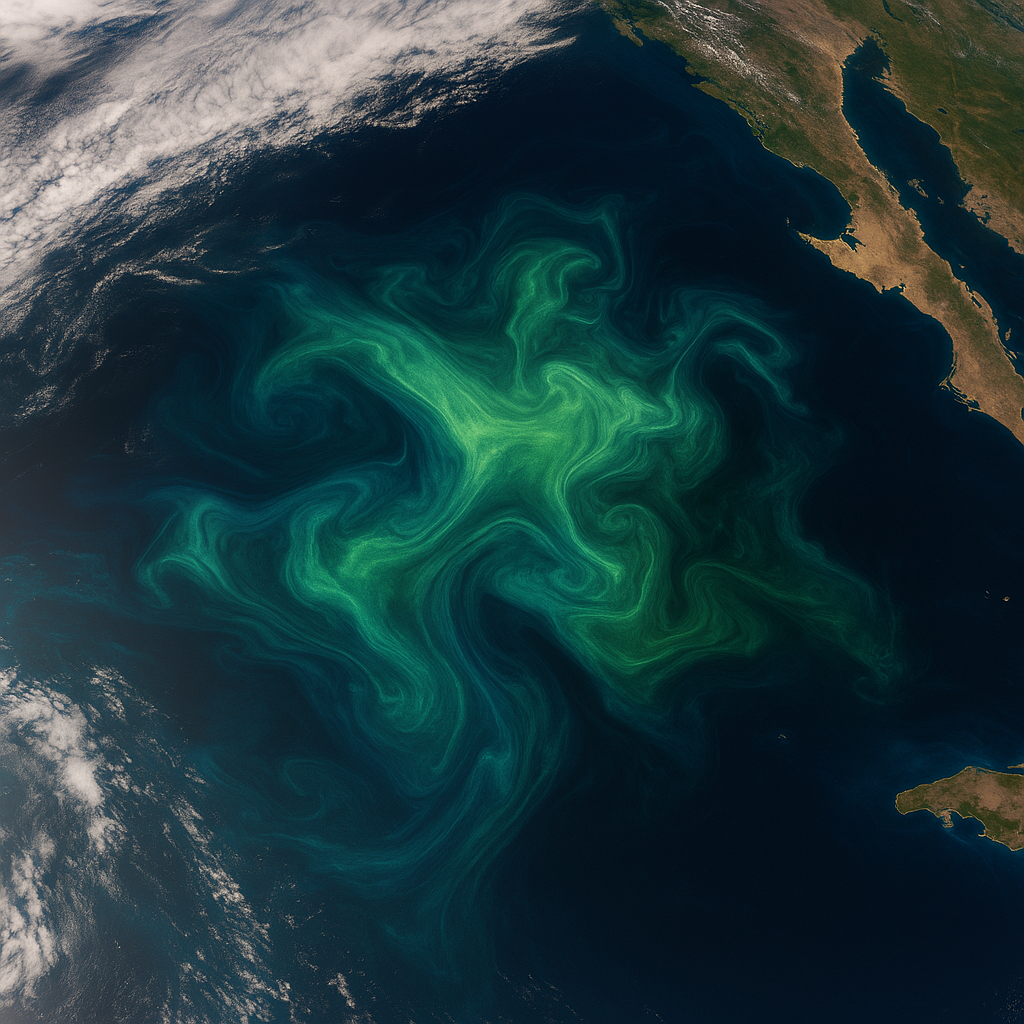
A review paper titled “A Review of Machine Learning Applications in Ocean Color Remote Sensing”, published in the journal Remote Sensing, presents the most comprehensive analysis to date of how machine learning (ML) is reshaping satellite-based oceanographic monitoring. Conducted by researchers from the Southern Marine Science and Engineering Guangdong Laboratory and the State Key Laboratory of Satellite Ocean Environment Dynamics, the study surveys the breadth of ML use in atmospheric correction, bio-optical inversion, data reconstruction, and carbon cycle analysis.
Drawing from over two decades of research, the paper charts an exponential growth in scientific output since 2015, reflecting a shift from conventional radiative transfer models to complex ML architectures. It emphasizes how neural networks, deep learning, and hybrid AI methods are overcoming traditional challenges in analyzing massive and complex datasets generated by satellite ocean color sensors.
Why ocean color remote sensing needs machine learning
Ocean color remote sensing (OCRS) provides crucial insights into marine ecosystems, detecting phytoplankton blooms, measuring chlorophyll-a concentrations, monitoring carbon cycles, and assessing ocean health. However, traditional models have struggled to process the nonlinear and high-dimensional relationships within ocean reflectance data, particularly in coastal waters where atmospheric interference and optical complexity are highest.
The study explains that ML models are particularly well-suited to OCRS because they can handle intricate relationships between inputs like chlorophyll-a, colored dissolved organic matter (CDOM), suspended sediments, and the remote sensing reflectance (Rrs) detected by satellites. Algorithms such as multilayer perceptrons (MLPs), convolutional neural networks (CNNs), and random forests (RFs) have demonstrated superior performance in retrieving accurate biogeochemical parameters and atmospheric corrections.
One standout application involves the estimation of air–sea carbon fluxes using self-organizing maps, significantly improving the accuracy of partial pressure of CO₂ (pCO₂) retrievals. Another example includes the use of physics-informed neural networks that embed oceanographic laws into training procedures, thus enhancing model reliability in real-world conditions.
How machine learning models are changing ocean monitoring
According to the bibliometric analysis included in the study, the integration of ML into OCRS began in earnest after 1997 but saw a sharp acceleration post-2015. Since then, researchers have developed and deployed a range of supervised, unsupervised, and reinforcement learning techniques. Applications include classification of water types, chlorophyll-a retrieval, inversion modeling, and anomaly detection for harmful algal blooms.
The report outlines key developments:
-
Atmospheric Correction: Deep neural networks have been trained to replace complex physical models for removing atmospheric effects, offering both speed and accuracy.
-
Chlorophyll and Particulate Retrieval: ML outperforms polynomial or empirical models in estimating pigment and carbon metrics from spectral data.
-
Multisource Data Fusion: By combining satellite data with in-situ and aerial measurements, ML models improve spatial and temporal resolution for critical parameters.
-
Automated Feature Extraction: Deep learning models reduce the need for manual preprocessing by learning abstract features directly from input data.
A co-occurrence network of frequently used keywords in the reviewed literature reveals dominant themes such as “chlorophyll-a,” “harmful algal blooms,” “classification,” “optical properties,” and “remote sensing reflectance.” These thematic concentrations underscore how ML has become a backbone of applied ocean color science, with tangible implications for fisheries, climate monitoring, and marine health surveillance.
What challenges remain and what’s next?
Despite remarkable progress, the study cautions that several challenges must be addressed for ML applications in OCRS to reach full maturity:
- Generalization: Many ML models are site- or dataset-specific, reducing their effectiveness across different marine regions or seasons.
- Data Quality: High-quality, labeled data remain limited, especially in optically complex coastal waters.
- Computational Demand: Deep models require substantial training time and GPU resources, potentially limiting use in real-time onboard satellite systems.
- Explainability: The ‘black box’ nature of many models complicates scientific interpretation and policy acceptance.
To overcome these barriers, the authors propose the expanded use of explainable AI (XAI), data fusion techniques, and hybrid modeling frameworks that combine ML with physical oceanographic principles. They also call for open-access benchmarking datasets to enable cross-study validation and reproducibility.
The paper also sheds light on emerging frontiers, including transfer learning for low-data environments, generative models for simulation-based training, and real-time onboard processing for next-generation satellite missions.
- READ MORE ON:
- ocean color remote sensing
- machine learning ocean monitoring
- AI in environmental monitoring
- AI for ocean health analysis
- machine learning applications in ocean color satellite imagery
- remote sensing of marine ecosystems using deep learning
- machine learning for real-time ocean monitoring
- FIRST PUBLISHED IN:
- Devdiscourse